A Scalable Rao-Blackwellised Sequential MCMC Sampler for Joint Detection and Tracking in Clutter.
FUSION(2023)
摘要
This paper addresses the joint detection and tracking of an unknown and time-varying number of targets in clutter. Here we formulate the tracking task in a variable-dimension state space, under which the reversible jump sequential Markov chain Monte Carlo sampling methods can be utilised to online estimate the target number, their kinematic states, and the association variables. In particular, a fast Rao-Blackwellisation scheme is devised to improve the tracking accuracy and sampling efficiency for linear Gaussian models. Based on the nonhomogeneous Poisson process measurement model, the developed tracker enjoys a partially parallel sampling structure, thereby being able to efficiently tackle the data association under massive measurements and clutter. The simulation results demonstrate that the developed tracker exhibits superior tracking performance in comparison to existing trackers in both accuracy and computational efficiency when tracking multiple targets under heavy clutter.
更多查看译文
关键词
data association,target tracking,detection,Rao-Blackwellisation,reversible jump sequential MCMC
AI 理解论文
溯源树
样例
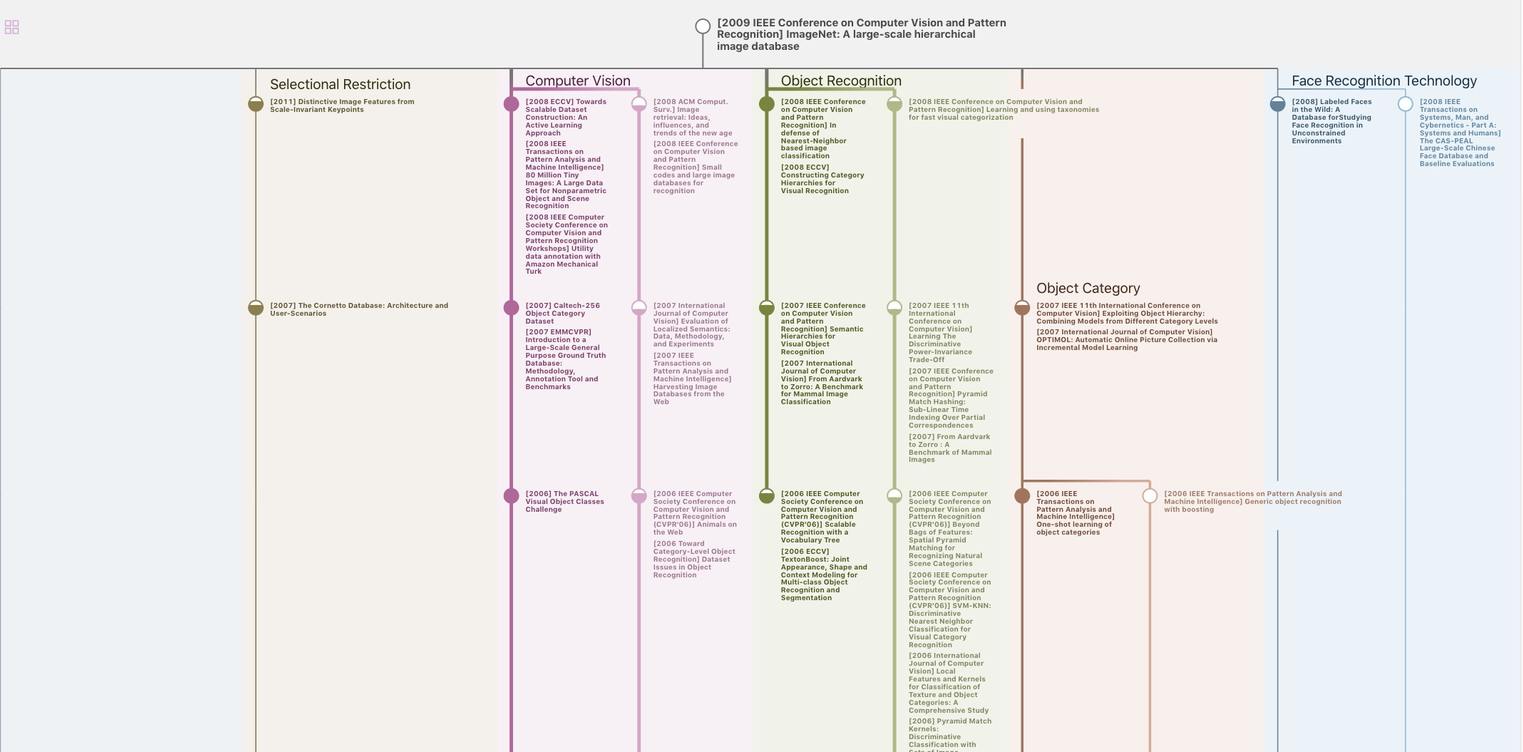
生成溯源树,研究论文发展脉络
Chat Paper
正在生成论文摘要