DFCP: Few-Shot DeepFake Detection via Contrastive Pretraining.
ICME(2023)
摘要
Abuses of forgery techniques have created a considerable problem of misinformation on social media. Although scholars devote many efforts to face forgery detection (a.k.a DeepFake detection) and achieve some results, two issues still hinder the practical application. 1) Most detectors do not generalize well to unseen datasets. 2) In a supervised manner, most previous works require a considerable amount of manually labeled data. To address these problems, we propose a simple contrastive pertaining framework for DeepFake detection (DFCP), which works in a finetuning-after-pretraining manner, and requires only a few labels (5%). Specifically, we design a two-stream framework to simultaneously learn high-frequency texture features and high-level semantics information during pretraining. In addition, a video-based frame sampling strategy is proposed to mitigate potential noise data in the instance-discriminative contrastive learning to achieve better performance. Experimental results on several downstream datasets show the state-of-the-art performance of the proposed DFCP, which works at frame-level (w/o temporal reasoning) with high efficiency but outperforms video-level methods.
更多查看译文
关键词
Face Forgery Detection,DeepFake,Self-supervised Learning,Contrastive Learning
AI 理解论文
溯源树
样例
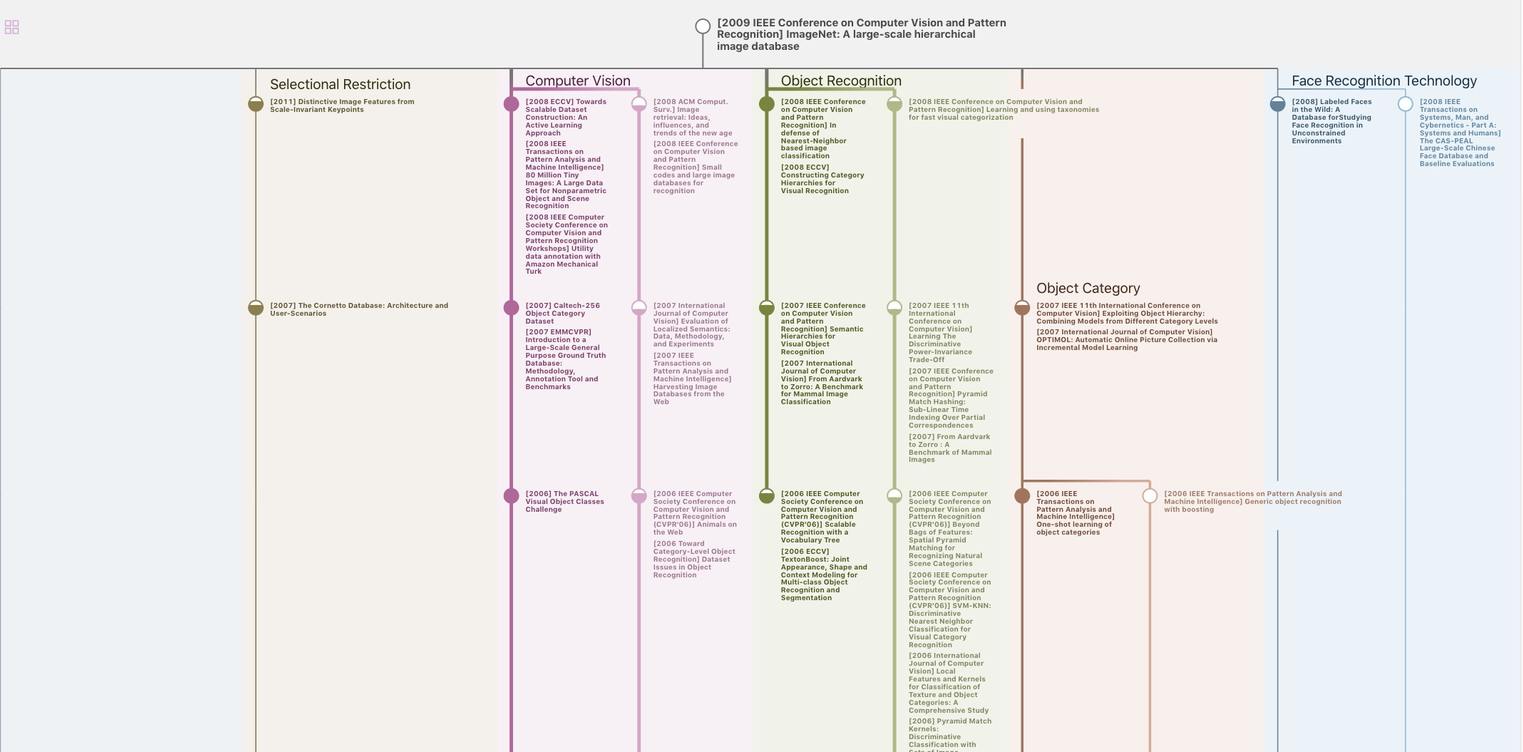
生成溯源树,研究论文发展脉络
Chat Paper
正在生成论文摘要