SQT: Debiased Visual Question Answering via Shuffling Question Types
ICME(2023)
摘要
Visual Question Answering (VQA) aims to obtain answers through image-question pairs. Nowadays, the VQA model tends to get answers only through questions, ignoring the information in the images. This phenomenon is caused by bias. As indicated by previous studies, the bias in VQA mainly comes from text modality. Our analysis of bias suggests that the question type is a crucial factor in bias formation. To interrupt the shortcut from question type to answer for de-biasing, we propose a self-supervised method for Shuffling Question Types (SQT) to reduce bias from text modality, which overcomes the prior language problem by mitigating the question-to-answer bias without introducing external annotations. Moreover, we propose a new objective function for negative samples. Experimental results show that our approach can achieve 61.76% accuracy on the VQA-CP v2 dataset, which outperforms the state-of-the-art in both self-supervised and supervised methods.
更多查看译文
关键词
Visual question answering,De-biasing,Self-supervised
AI 理解论文
溯源树
样例
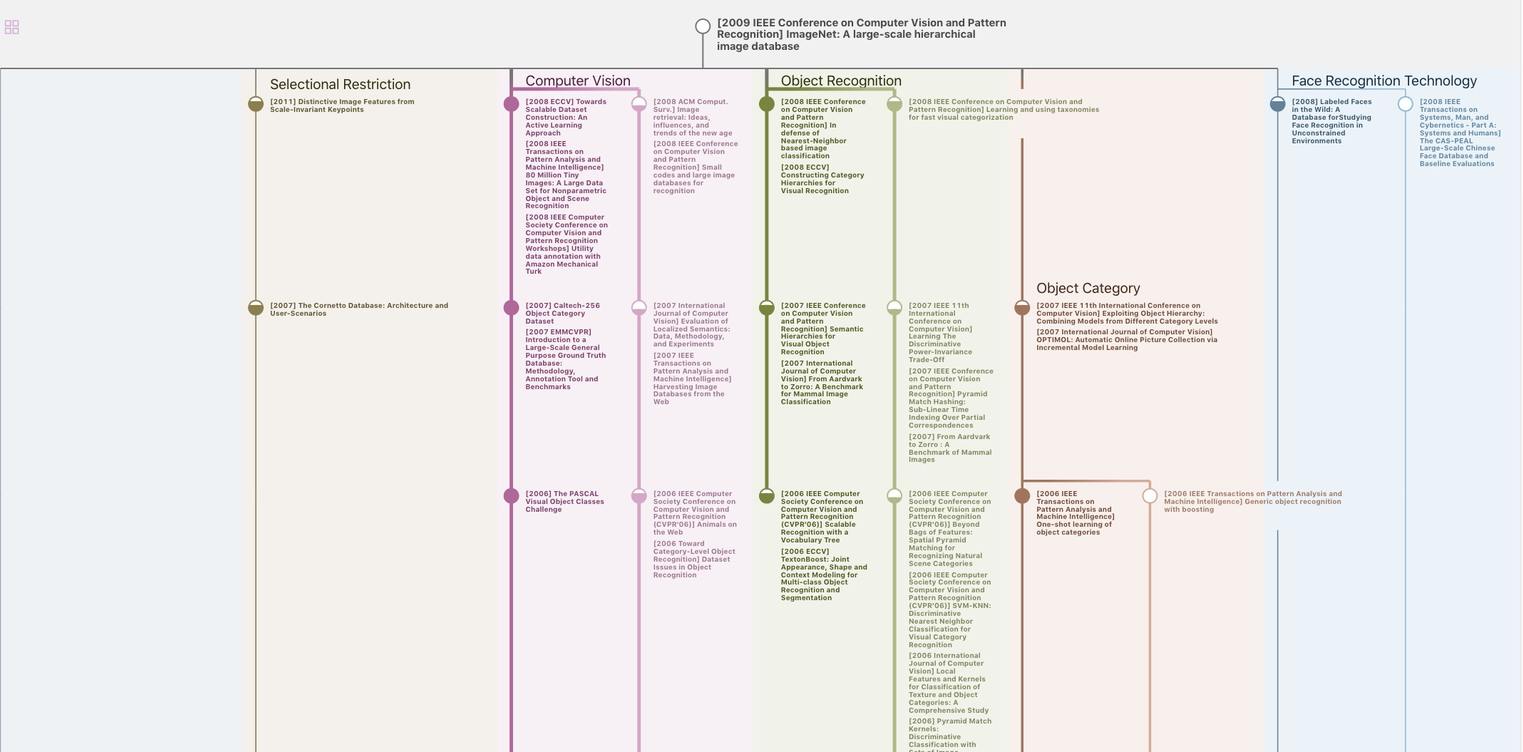
生成溯源树,研究论文发展脉络
Chat Paper
正在生成论文摘要