Federated Domain Adaptation via Pseudo-label Refinement
ICME(2023)
摘要
Unsupervised domain adaptation (UDA) methods usually assume data from multiple domains can be put together for centralized adaptation. Unfortunately, this assumption impairs data privacy, which leads to the failure of traditional methods in practical scenarios. To cope with the above issue, we present a novel decentralized domain adaptation approach which conducts target adaptation in an iterative training process during which only models can be delivered across domains. More specifically, to train a promising target model, we leverage Adversarial Examples (AEs) to filter out error prone predictions of source models towards each target sample based on both robustness and confidence, and then treat the most frequent prediction as the pseudo-label. Besides, to improve central model aggregation, we introduce Knowledge Contribution (KC) to compute reasonable aggregation weights. Extensive experiments conducted on several standard datasets verify the superiority of the proposed method.
更多查看译文
关键词
Decentralized Domain Adaptation, Adversarial Examples, Federated Learning
AI 理解论文
溯源树
样例
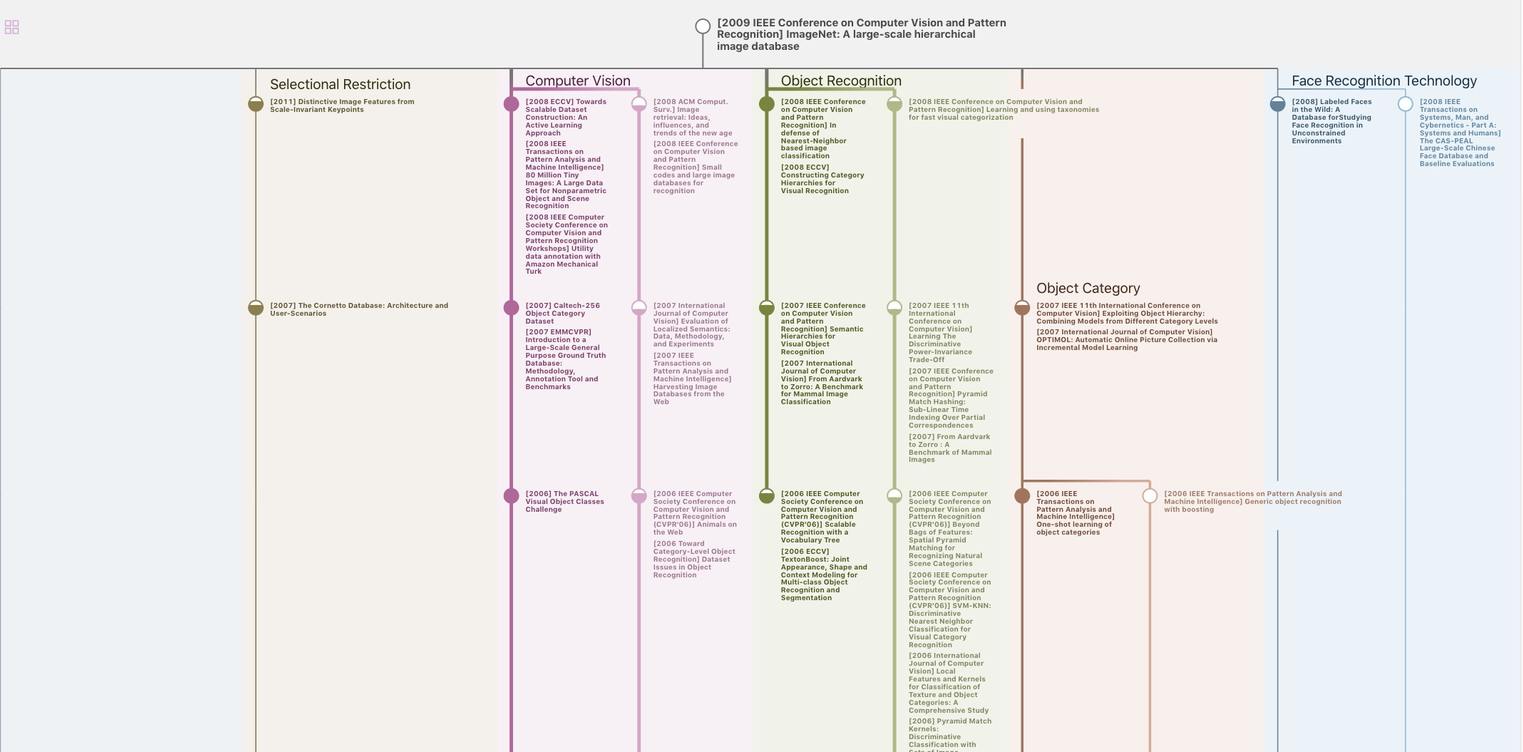
生成溯源树,研究论文发展脉络
Chat Paper
正在生成论文摘要