Improving Vision Transformers with Nested Multi-head Attentions.
ICME(2023)
摘要
Vision transformers have significantly advanced the field of computer vision in recent years. The cornerstone of these transformers is the multi-head attention mechanism, which models interactions between visual elements within a feature map. However, the vanilla multi-head attention paradigm independently learns parameters for each head, which ignores crucial interactions across different attention heads and may result in redundancy and under-utilization of the model's capacity. To enhance model expressiveness, we propose a novel nested attention mechanism, Ne-Att, that explicitly models cross-head interactions via a hierarchical variational distribution. We conducted extensive experiments on image classification, and the results demonstrate the superiority of Ne-Att.
更多查看译文
关键词
Vision Transformers,Disentangled Representation
AI 理解论文
溯源树
样例
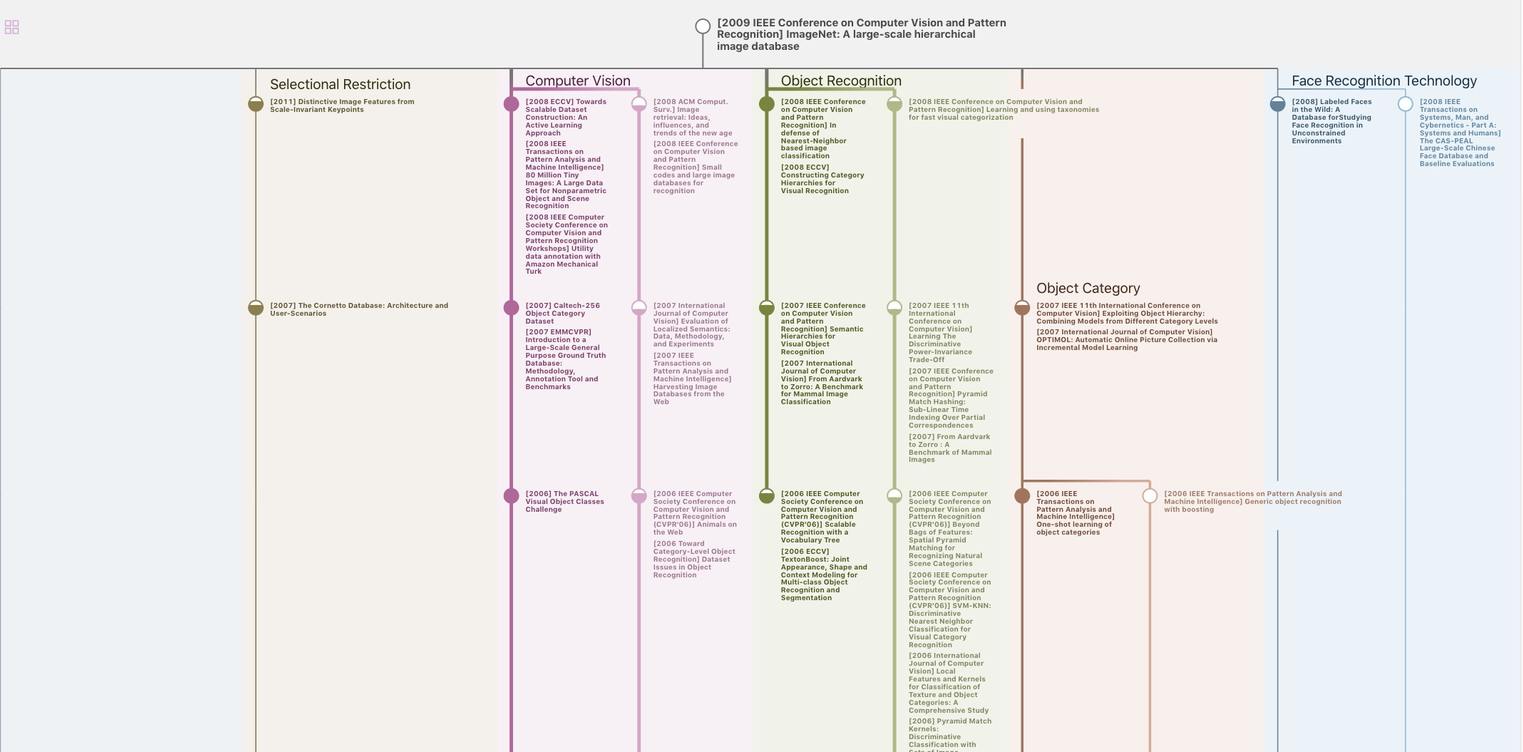
生成溯源树,研究论文发展脉络
Chat Paper
正在生成论文摘要