Explainable Unfolding Network For Joint Edge-Preserving Depth Map Super-Resolution.
ICME(2023)
摘要
Although color-guided depth map super-resolution methods based on deep learning have achieved great progress, these methods are not explainable and their super-resolution results are suffered from texture-copying and boundary blurring problems. In order to alleviate these problems, we propose an explainable depth map super-resolution network by unfolding edge-constrained optimization model, dubbed Ex-DSRNet. First, we propose an edge reconstruction network to recover accurate edge information, then feed edge information and color information into different depth map reconstruction networks to reconstruct depth information. Finally, a high-fidelity network is proposed to fuse the above two kinds of depth information to obtain high-quality depth map. A large number of experimental results have demonstrated that the proposed Ex-DSRNet can compete against many state-of-the-art depth map super-resolution methods in term of root mean square error.
更多查看译文
关键词
edge-constrained optimization, depth map, image super-resolution, deep explainable network
AI 理解论文
溯源树
样例
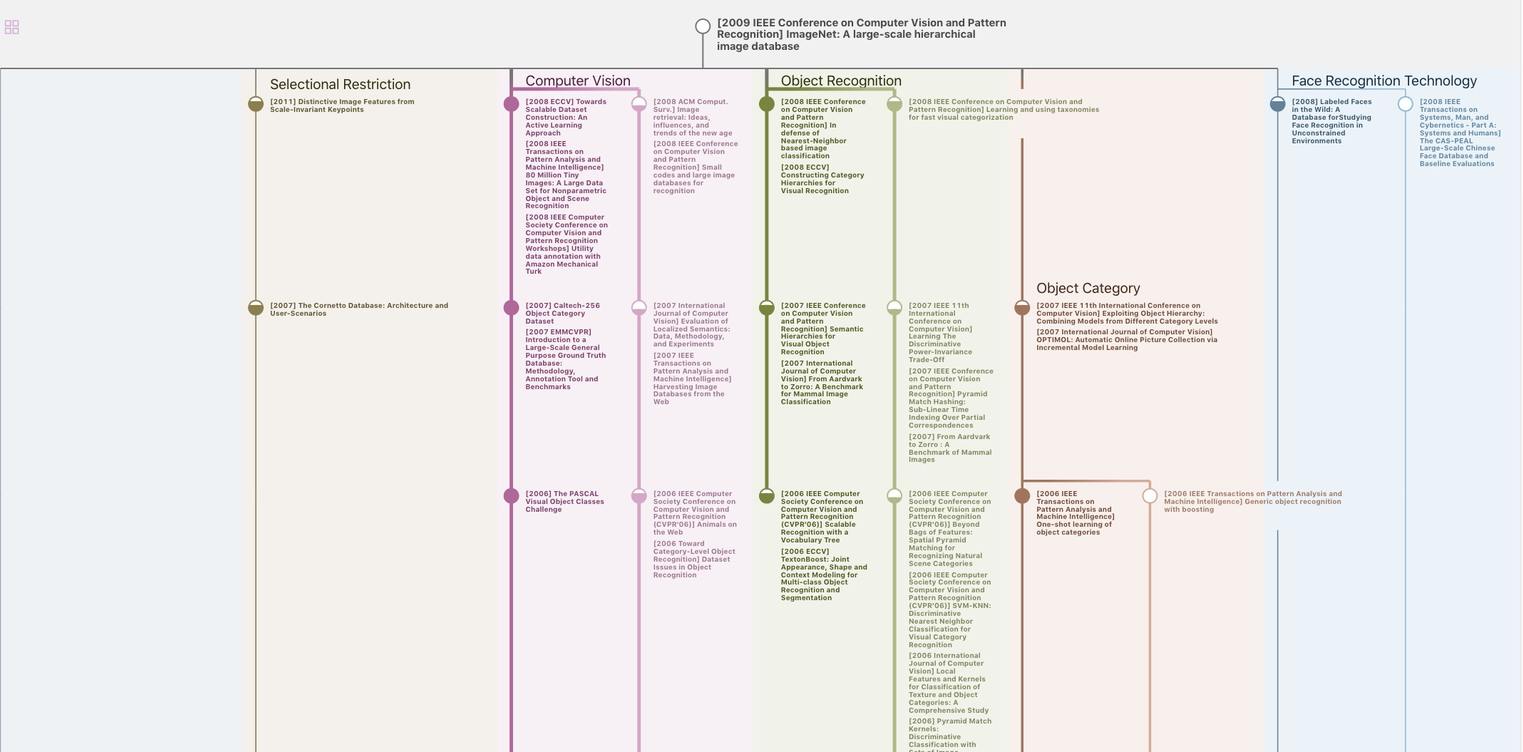
生成溯源树,研究论文发展脉络
Chat Paper
正在生成论文摘要