Adversarially Robust Source-free Domain Adaptation with Relaxed Adversarial Training.
ICME(2023)
摘要
Unsupervised Domain Adaptation (UDA) learns a model for an unlabeled target domain, utilizing a labeled source domain. Most existing works on UDA assume the availability of source data and neglect the adversarial robustness of the models, hindering security-sensitive real-world applications. In this paper, we study adversarially robust source-free UDA, aiming to train a robust target model by adapting a non-robust source model without using source data. A basic approach is to train a non-robust teacher model via conventional source-free UDA to predict pseudo-labels for target data, and then train a robust student model via adversarial training (AT). However, AT tends to magnify the errors of the teacher model, reducing the accuracy. Hence, we propose Relaxed Adversarial Training (RAT) that relieves the constraints on the confidence of predictions in AT to balance the robustness and accuracy. Extensive experiments validate that RAT can improve the accuracy on clean and adversarial samples, and is superior to related methods. Our code is available at https://github.com/Coxy7/RAT.
更多查看译文
关键词
Unsupervised domain adaptation,adversarial robustness,adversarial training
AI 理解论文
溯源树
样例
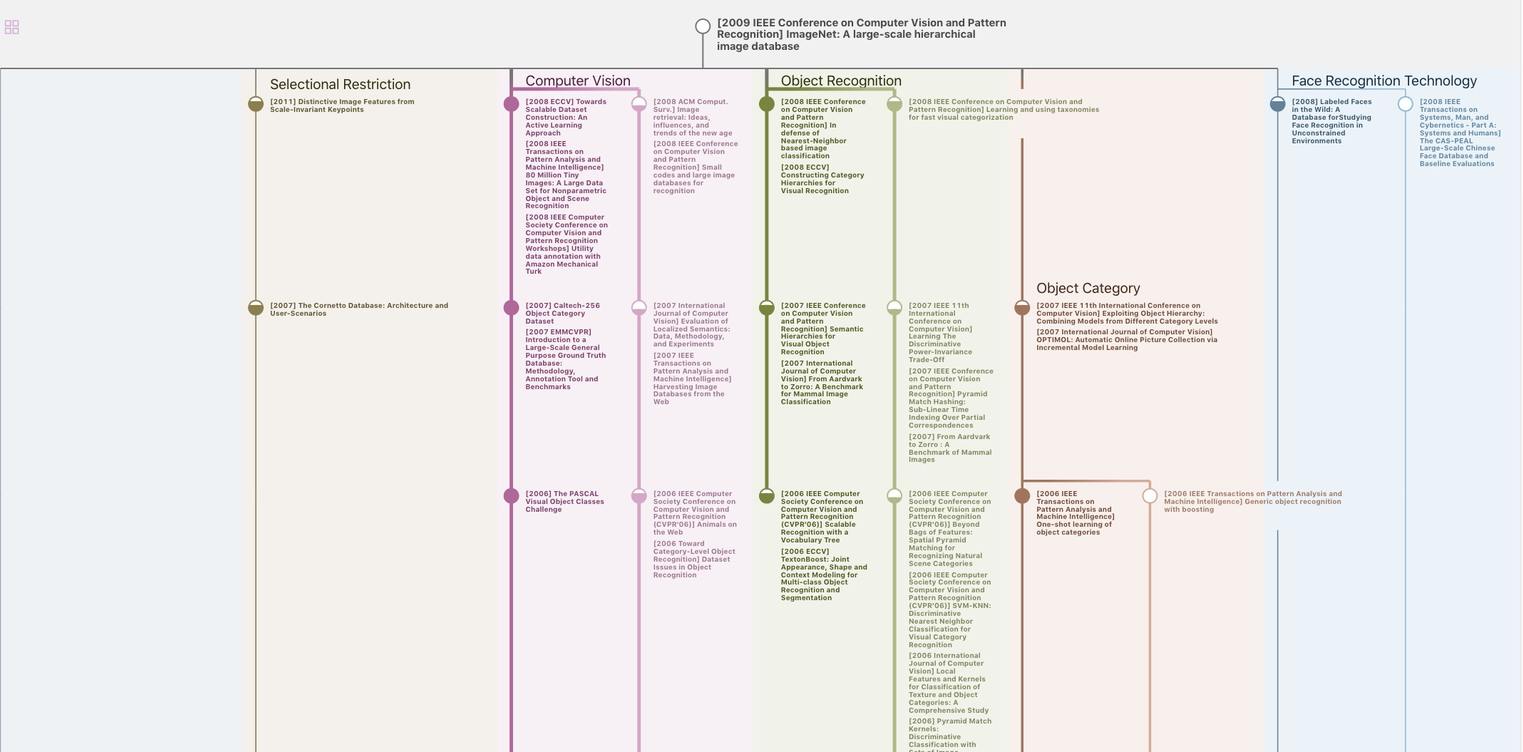
生成溯源树,研究论文发展脉络
Chat Paper
正在生成论文摘要