EFT: Expert Fusion Transformer for Voice-Face Association Learning.
ICME(2023)
摘要
Learning associations between voices and faces have recently attracted much research interest. Previous studies mainly focused on improving loss function design for learning better representations, neglecting that informative inputs are the prerequisite of association findings and effective representation alignments. Based on this motivation, we proposed an unsupervised transformer-based learning framework, which fuses the knowledge of readily available single-modal expert models to learn better representations. Benefiting from higher-quality inputs, a simple noise contrastive estimation (NCE) loss is used for training. In addition, we proposed a statistical batch construction (SBC) strategy, which obtains high-quality negative samples during the unsupervised learning process. Experiments on the VoxCeleb1 dataset demonstrate the effectiveness of our framework. It yields SOTA results in voice-face verification, matching, and retrieval tasks. In the challenging gender-constrained matching tasks, it achieves over 81% (ACC), which is 5% higher than the previous.
更多查看译文
关键词
voice-face association,cross-modal retrieval,multi-modal learning,unsupervised learning
AI 理解论文
溯源树
样例
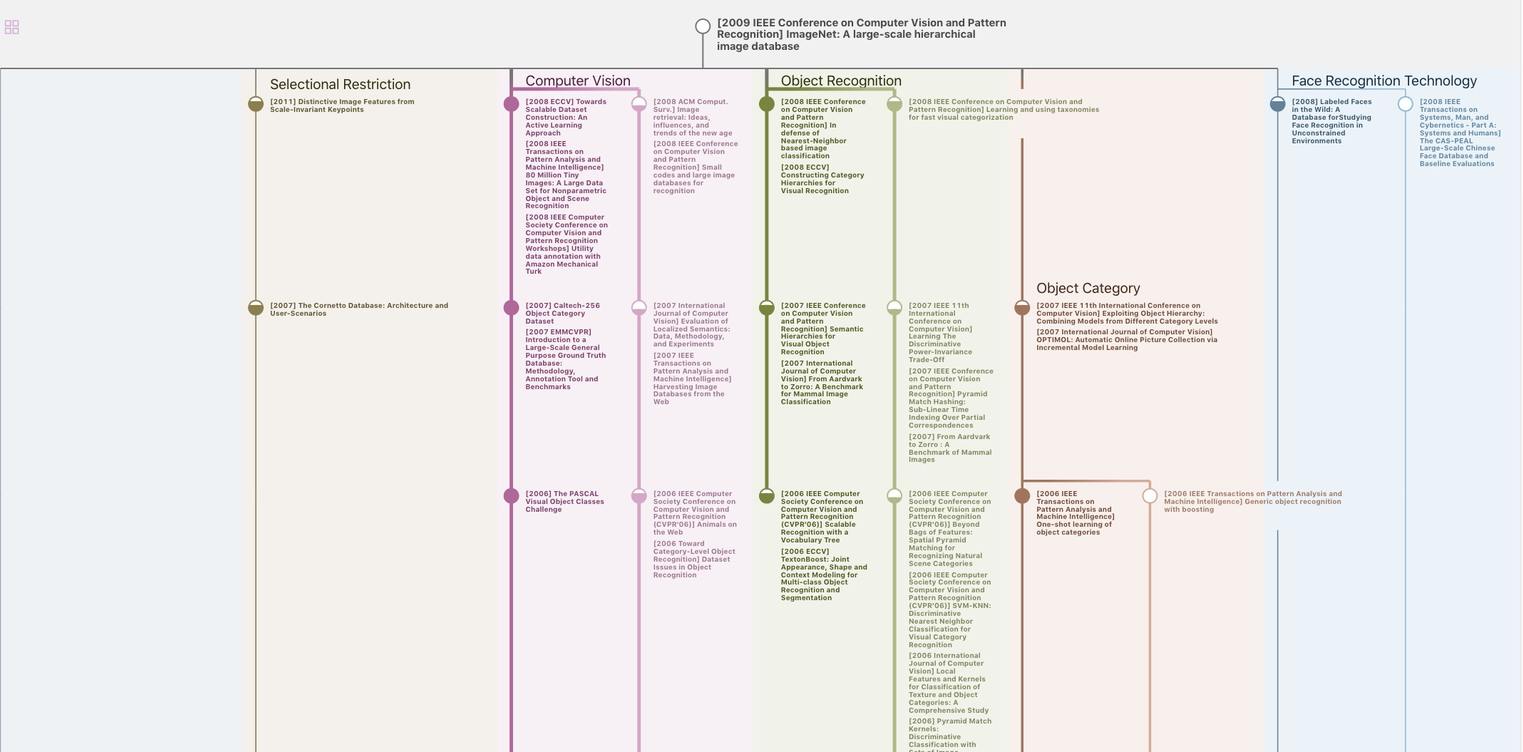
生成溯源树,研究论文发展脉络
Chat Paper
正在生成论文摘要