Gender Bias in AI Recruitment Systems: A Sociological-and Data Science-based Case Study.
ISTAS(2022)
摘要
This paper explores the extent to which gender bias is introduced in the deployment of automation for hiring practices. We use an interdisciplinary methodology to test our hypotheses: observing a human-led recruitment panel and building an explainable algorithmic prototype from the ground up, to quantify gender bias. The key findings of this study are threefold: identifying potential sources of human bias from a recruitment panel’s ranking of CVs; identifying sources of bias from a potential algorithmic pipeline which simulates human decision making; and recommending ways to mitigate bias from both aspects. Our research has provided an innovative research design that combines social science and data science to theorise how automation may introduce bias in hiring practices, and also pinpoint where it is introduced. It also furthers the current scholarship on gender bias in hiring practices by providing key empirical inferences on the factors contributing to bias.
更多查看译文
关键词
algorithmic bias,gender,recruitment,CV
AI 理解论文
溯源树
样例
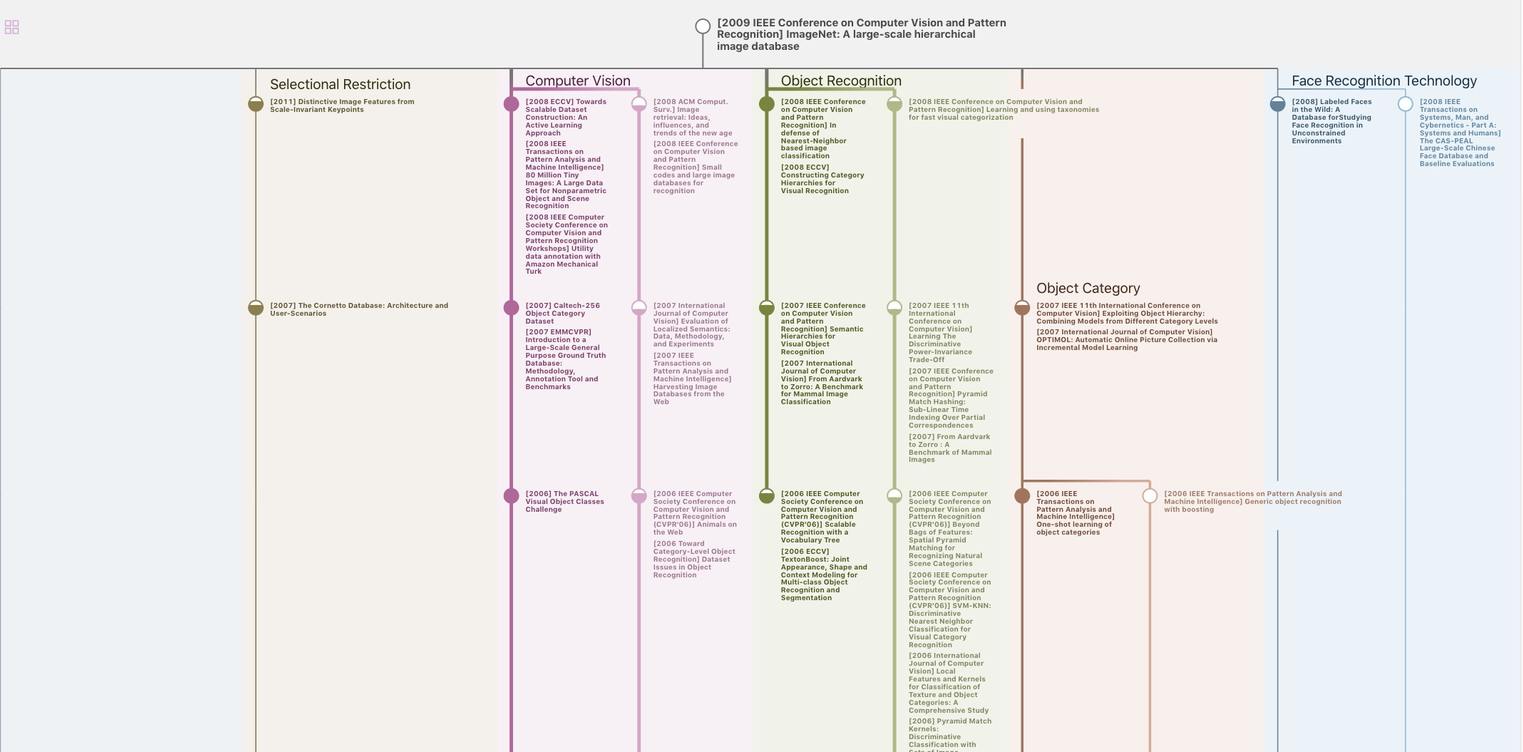
生成溯源树,研究论文发展脉络
Chat Paper
正在生成论文摘要