Max-Entropy Sampling for Deterministic Timed Automata Under Linear Duration Constraints
QUANTITATIVE EVALUATION OF SYSTEMS, QEST 2023(2023)
摘要
Adding probabilities to timed automata enables one to carry random simulation of their behaviors and provide answers with statistical guarantees to problems otherwise untractable. Thus, when just a timed language is given, the following natural question arises: What probability should we consider if we have no a priori knowledge except the given language and the considered length (i.e. number of events) of timed words? The maximal entropy principle tells us to take the probability measure that maximises the entropy which is the uniform measure on the language restricted to timed word of the given length (with such a uniform measure every timed word has the same chance of being sampled). The uniform sampling method developed in the last decade provides no control on the duration of sampled timed words. In the present article we consider the problem of finding a probability measure on a timed language maximising the entropy under general linear constraints on duration and for timed words of a given length. The solution we provide generalizes to timed languages a well-known result on probability measure over the real line maximising the Shannon continuous entropy under linear constraints. After giving our general theorem for general linear constraints and for general timed languages, we concentrate to the case when only the mean duration is prescribed (and again when the length is fixed) for timed languages recognised by deterministic timed automata. For this latter case, we provide an efficient sampling algorithm we have implemented and illustrated on several examples.
更多查看译文
AI 理解论文
溯源树
样例
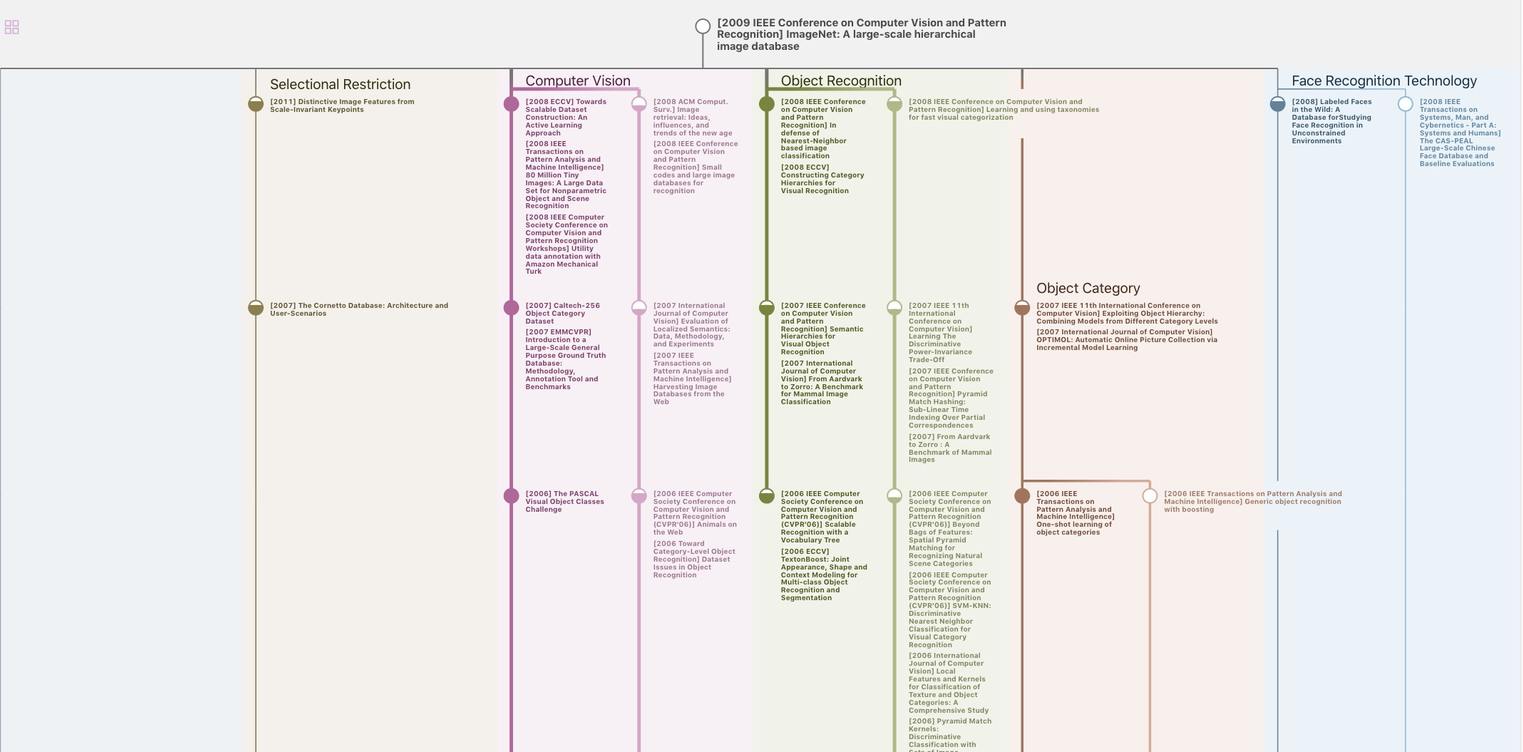
生成溯源树,研究论文发展脉络
Chat Paper
正在生成论文摘要