Enhancing Hierarchical Vector Quantized Autoencoders for Image Synthesis Through Multiple Decoders
IMAGE ANALYSIS AND PROCESSING, ICIAP 2023, PT II(2023)
摘要
Vector Quantized Variational Autoencoders (VQ-VAEs) have gained popularity in recent years due to their ability to represent images as discrete sequences of tokens that index a learned codebook of vectors, enabling efficient image compression. One variant of particular interest is VQ-VAE 2, which extends previous works by representing images as a hierarchy of sequences, resulting in finer-grained representations. In this study, we further enhance such hierarchical autoencoder approach by introducing multiple decoders, which allow to represent images as a sum of multi-scale contributions in the pixel space. Our proposed model, the Multi Scale (MS) VQ-VAE, not only enables better control over the encoding of each sequence (resulting in improved explainability and codebook usage) but, as a consequence, also shows advantages in image synthesis. Our experiments demonstrate that the MS-VQVAE achieves comparable or superior reconstructions on various datasets and resolutions, as well as greater stability across runs. Moreover, we include a proof-of-concept trial to showcase the potential applications of our model in image synthesis.
更多查看译文
关键词
VQ-VAE,Hierarchical Autoencoder,Image synthesis
AI 理解论文
溯源树
样例
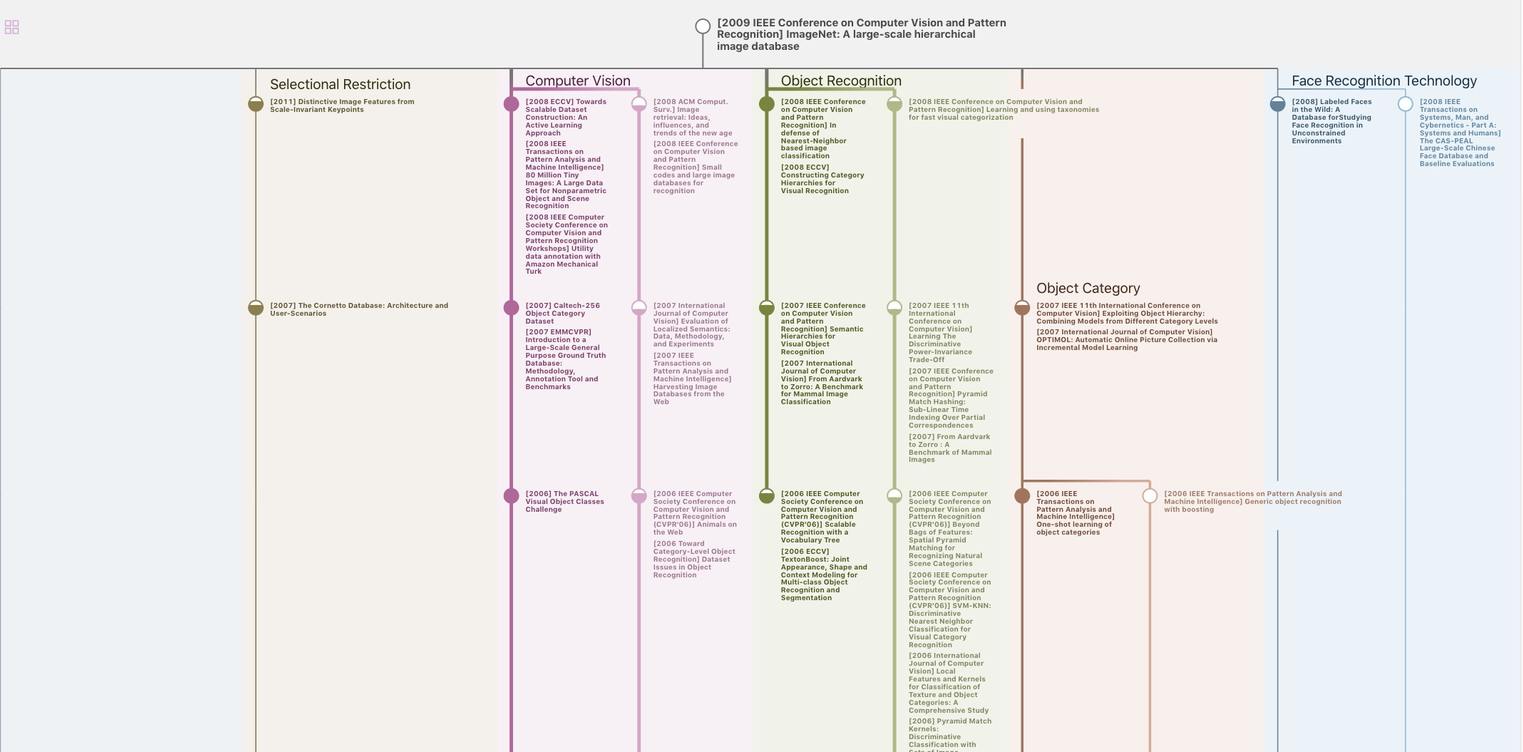
生成溯源树,研究论文发展脉络
Chat Paper
正在生成论文摘要