Optimizing a 5G mmWave Hybrid Beamforming Architecture in a Reinforcement Learning Approach.
ICCC(2023)
摘要
Hybrid beamforming is a commonly used technique to effectively balance system performance and hardware complexity in 5G communications, which focuses on millimeter wave (mmWave) and the massive multiple-input multiple-output (MIMO) technology. In this paper, we propose a dynamic port mapping hybrid beamforming architecture that exploits the advantages of dynamic subarrays and dynamic RF chains. The dynamic port mapping architecture adjusts the number of activated ports and the formation of subarrays in real time based on channel state information to achieve trade-off between spectral and energy efficiency. We consider the port mapping problem in the context of 5G NR applications and channel state information (CSI) measurement standards. We also design a reinforcement learning algorithm to interact with the environment and make port mapping decisions based on the current channel state. Our experimental results show that this dynamic port mapping architecture optimized by the DQN algorithm can significantly improve energy efficiency. Compared with dynamic subarray and dynamic RF chain architectures, it achieves a 15% and 25% energy efficiency gain, respectively, with a spectral efficiency loss of 3.3% and 5%. The proposed hybrid beamforming architecture effectively enables dynamic port mapping and helps optimize the energy efficiency of the hybrid beamforming architecture, which is expected to significantly reduce the cost of 5G network communication.
更多查看译文
关键词
Hybrid Beamforming,Reinforcement Learning,mmWave Massive MIMO,Beam Management
AI 理解论文
溯源树
样例
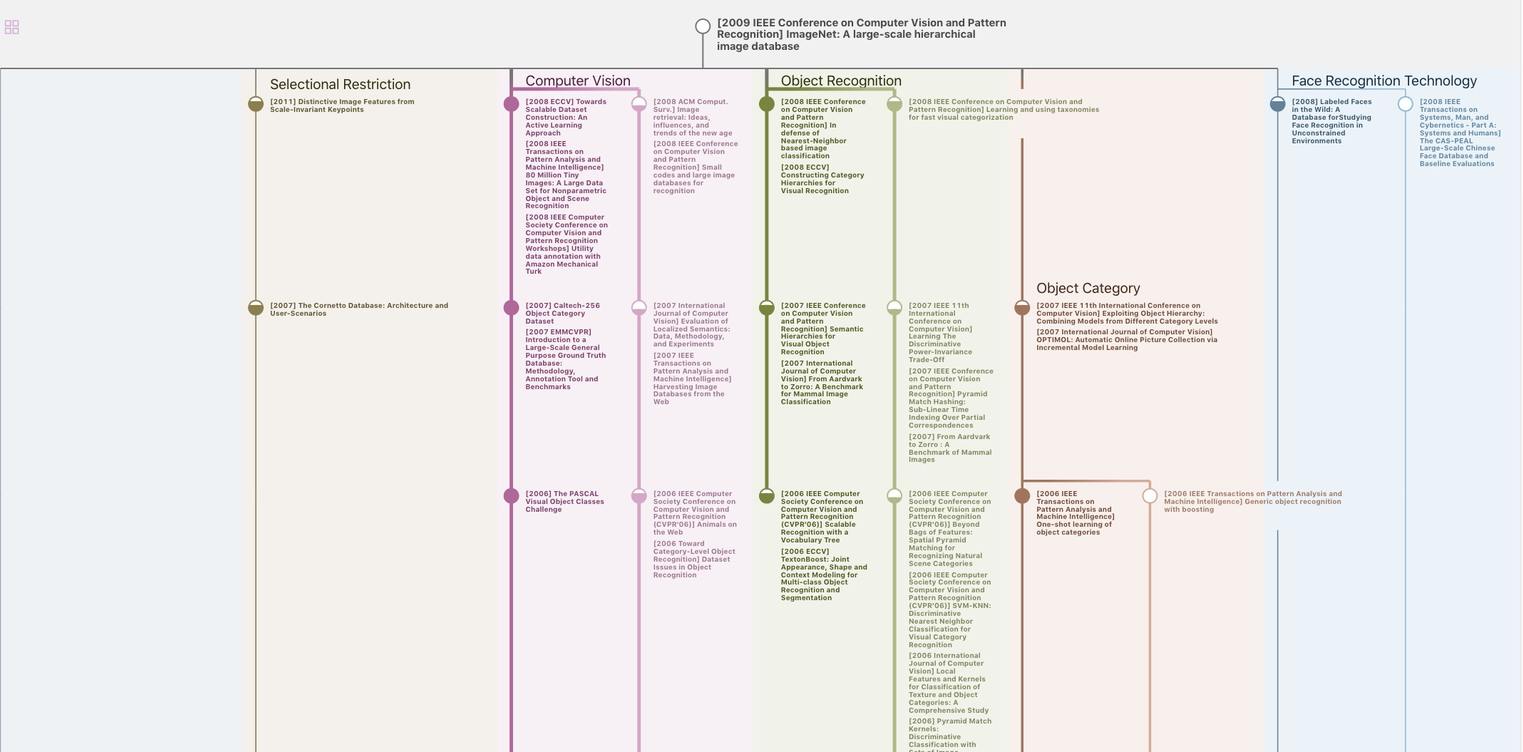
生成溯源树,研究论文发展脉络
Chat Paper
正在生成论文摘要