Maritime Search and Rescue Leveraging Heterogeneous Units: A Multi-Agent Reinforcement Learning Approach.
ICCC(2023)
摘要
In recent years, with the continuous growth of offshore operations, maritime search and rescue (MSAR) has received widespread attention as a crucial guarantee for safety. In this paper, we aim to achieve efficient and communication fault-tolerant MSAR operations by employing trajectory planning and resource scheduling for heterogeneous units involving observation unmanned aerial vehicles (UAVs), router UAVs, and rescue ships equipped with mobile edge computing (MEC). Firstly, we model several essential components related to MSAR, including the ocean current environment, UAVs for observing, fault tolerance of routing network, MEC scheduling, and energy consumption of UAVs. Secondly, we formulate the optimization problem into a decentralized partially observable Markov Decision Process (Dec-POMDP) and then introduce the multi-agent reinforcement learning (MARL) approach to search for an optimal joint strategy. Finally, experimental results demonstrate that in the model of MSAR we constructed, our improved MARL approach, named IPPO-nGAE, outperforms other benchmarks in both efficiency and fault tolerance.
更多查看译文
关键词
Maritime search and rescue,multi-agent reinforcement learning,efficiency,fault-tolerant communication,unmanned aerial vehicle,rescue ship
AI 理解论文
溯源树
样例
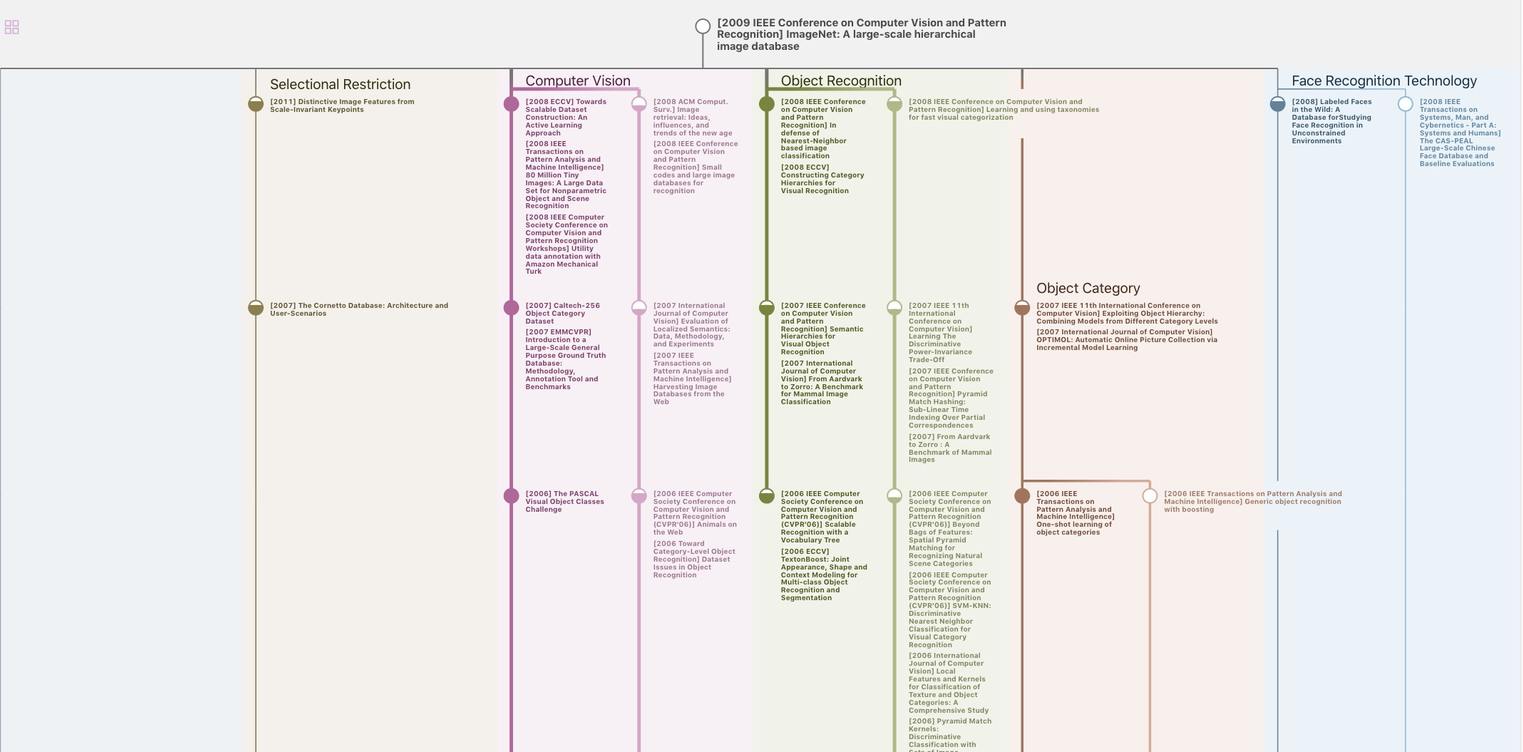
生成溯源树,研究论文发展脉络
Chat Paper
正在生成论文摘要