Color-coded Attribute Graph: Visual Exploration of Distinctive Traits of IoT-Malware Families.
ISCC(2023)
摘要
This study investigates the use of explainable artificial intelligence (XAI) to identify the unique features distinguishing malware families and subspecies. The proposed method, called the color-coded attribute graph (CAG), employs XAI and visualization techniques to create a visual representation of malware samples. The CAG utilizes the feature importance scores (ISs) obtained from a pre-trained classifier model and a scale function to normalize the scores for visualization. The approach assigns each family a representative color. The features are color-coded according to their relevance to the malware family. This work evaluates the proposed method on a dataset of 13,823 Internet of Things malware samples and compares two approaches for feature IS extraction using Linear Support Vector Machine and Local Interpretable Model-Agnostic Explanations. The experimental results demonstrate the effectiveness of the CAG in interpreting machine learning-based methods for malware detection and classification, leading to more accurate analyses.
更多查看译文
关键词
Internet of Things,XAI,Data Visualization,Malware Analysis
AI 理解论文
溯源树
样例
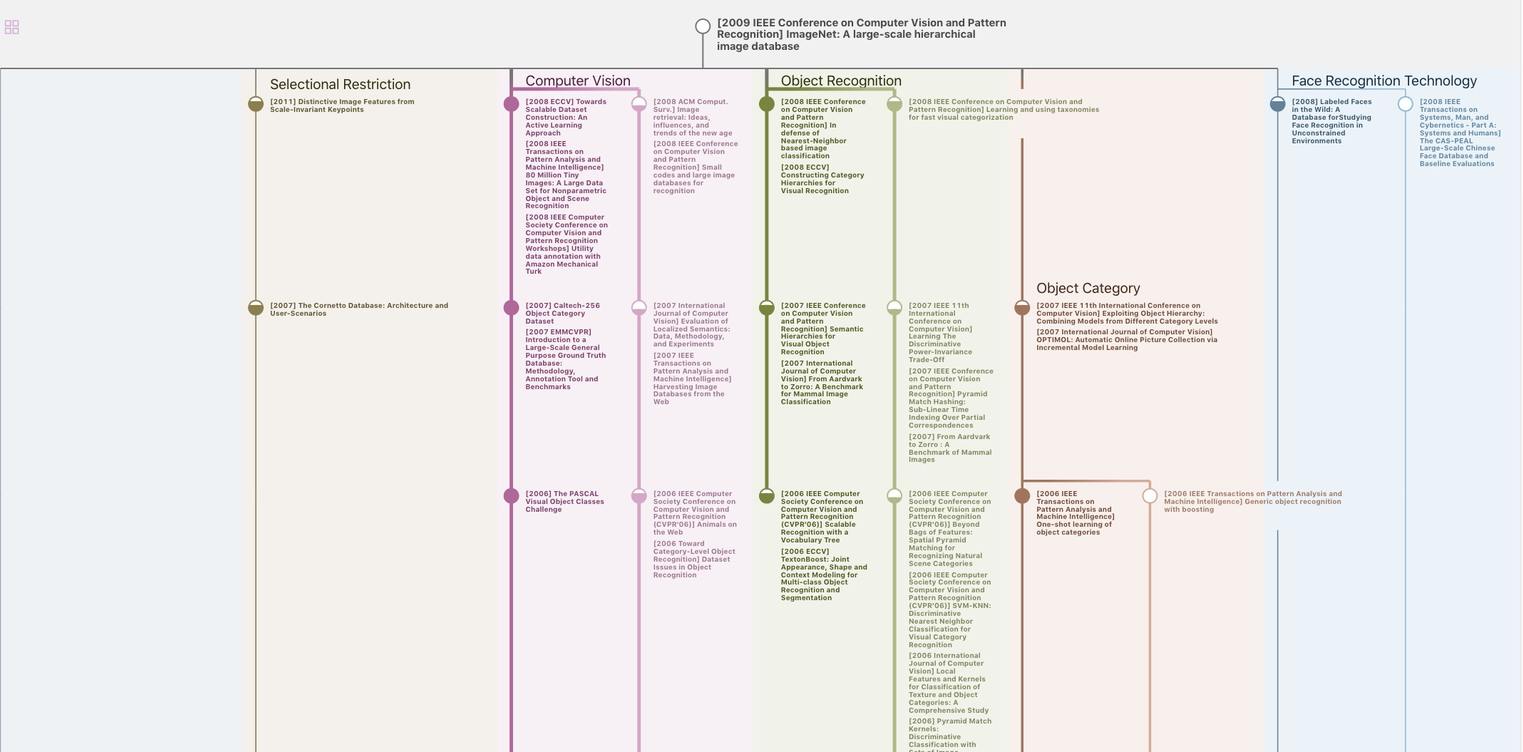
生成溯源树,研究论文发展脉络
Chat Paper
正在生成论文摘要