CoTuner: A Hierarchical Learning Framework for Coordinately Optimizing Resource Partitioning and Parameter Tuning
PROCEEDINGS OF THE 52ND INTERNATIONAL CONFERENCE ON PARALLEL PROCESSING, ICPP 2023(2023)
摘要
The performance of modern multi-core systems is reliant upon two crucial configurations: how the resources are partitioned among the co-located applications to mitigate resource contention, and the setting of the parameters of applications. However, finding the optimal resource partition configuration and parameter setting for the co-located applications is challenging due to the prohibitively large search space and the high interdependency between resource partitioning and parameter tuning. We propose CoTuner, a hierarchical learning framework designed to simultaneously and efficiently explore optimal combinations of resource partitioning and application parameters. The central proposition of CoTuner is to decompose the co-tuning challenge into multiple sub-problems and tackle them using small-scale learning-based models (namely Bayesian Optimization) collaboratively. Additionally, we have introduced several refinements to ensure that this learning-based framework operates in an efficient manner. Extensive evaluations show that CoTuner can find better configurations faster than state-of-the-art baselines by 2.16x to 4.96x.
更多查看译文
关键词
Workload consolidation,Resource partitioning,Parameter tuning
AI 理解论文
溯源树
样例
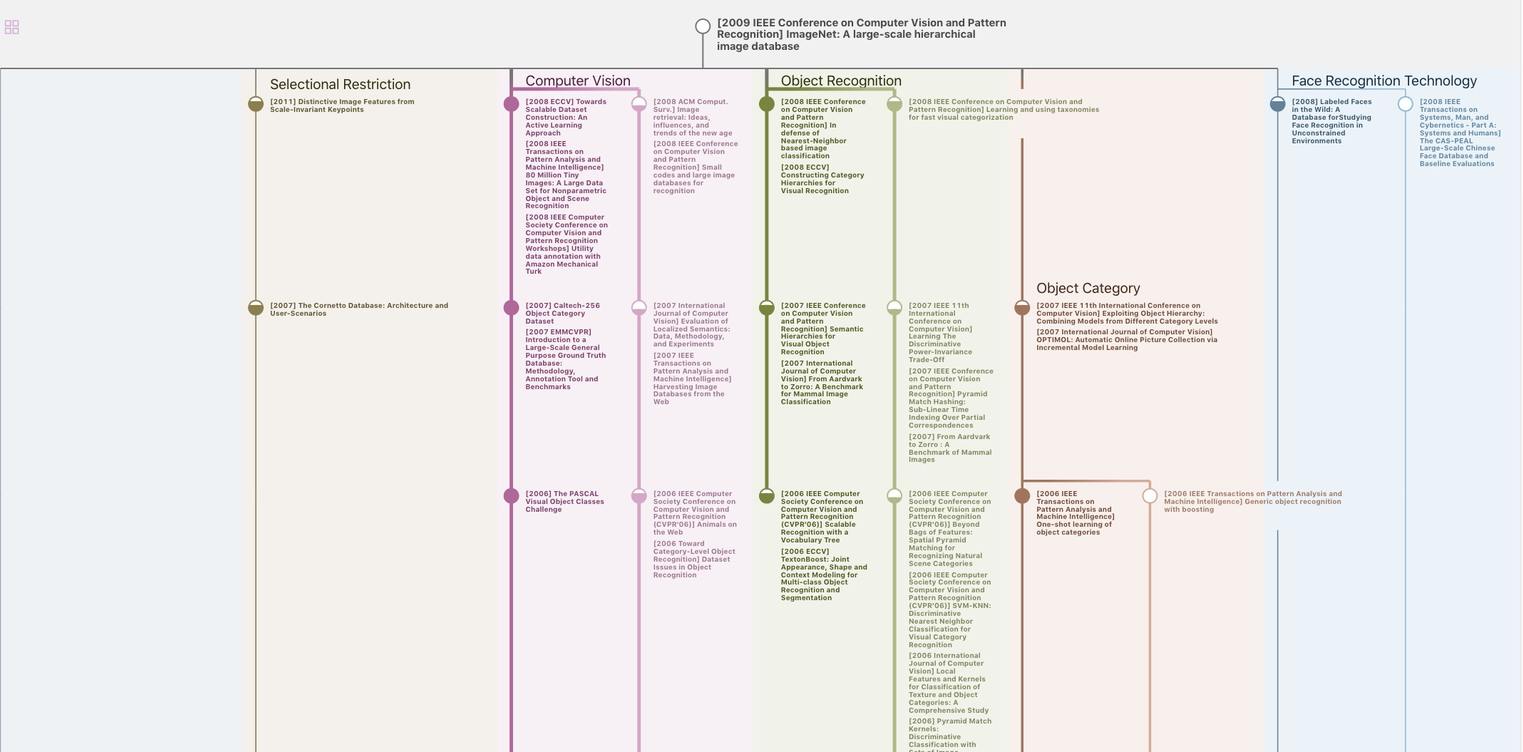
生成溯源树,研究论文发展脉络
Chat Paper
正在生成论文摘要