Grading Diabetic Retinopathy Using Transfer Learning-Based Convolutional Neural Networks.
CISIM(2023)
摘要
Diabetic Retinopathy (DR) is a disease that affect the retina, consequence of a diabetes complication. An accurate an on time diagnosis could delay severe damage in the eye or vision loss. Currently, the diagnosis is supported by a retinography and visual evaluation by a trained clinician. However, retinography evaluation is a difficult task due to differences in contrast, brightness and the presence of artifacts. In this work we propose a convolutional neural network (CNN) model to detect and grading DR to support the diagnosis from a fundus image. Moreover, we used the transfer learning technique to reuse the first layers from deep neural networks previously trained. We carried out experiments using different convolutional architectures and their performance for DR grading was evaluated on the APTOS database. The VGG-16 was the architecture with higher results, overcoming the other networks and other related works. The best experimentation we obtained reached an accuracy value of 83.52% for DR grading tasks. Experimental results show that the CNN based transfer learning achieve high performance taking the knowledge learning from pretrained network and saving computational time in the training process, which turns out suitable for small dataset as DR medical images.
更多查看译文
关键词
diabetic retinopathy,convolutional neural networks,learning-based
AI 理解论文
溯源树
样例
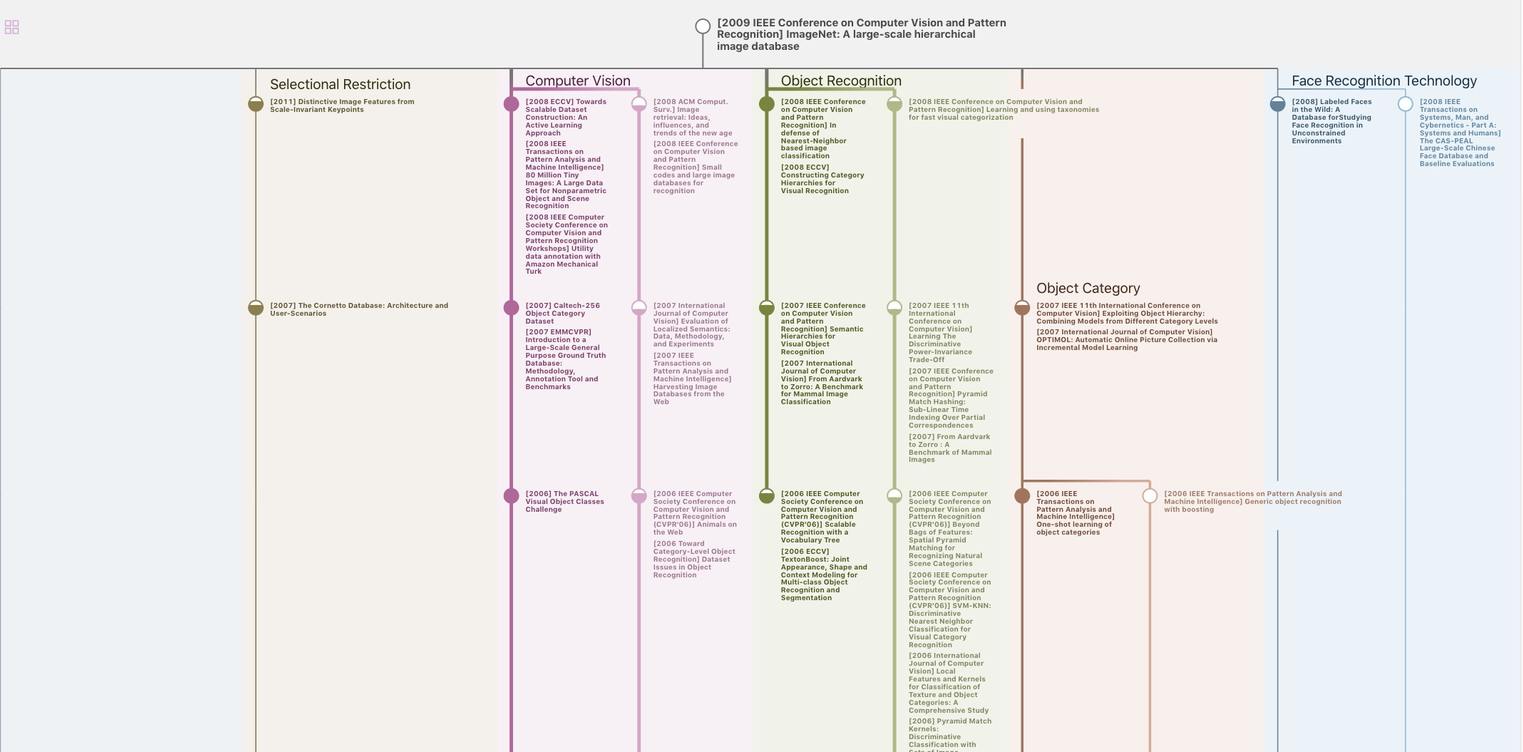
生成溯源树,研究论文发展脉络
Chat Paper
正在生成论文摘要