MMDF-ESI: Multi-Modal Deep Fusion of EEG and MEG for Brain Source Imaging.
BI(2023)
摘要
Electrophysiological Source Imaging (ESI) aims to reconstruct the underlying electric brain sources based on Electroencephalography (EEG) or Magnetoencephalography (MEG) measurements of brain activities. Due to the ill-posed nature of the ESI problem, faithfully recovering the latent brain sources has been a significant challenge. Classical algorithms have primarily focused on the design of regularization terms according to predefined priors derived from neurophysiological assumptions. Deep learning frameworks attempt to learn the mapping between brain source signals and scalp EEG/MEG measurements in a data-driven manner, and have demonstrated improved performance compared to the classical methods. Given that EEG and MEG can be complementary for measuring the tangential and radial electrical signals of the cortex, combining both modalities is believed to be advantageous in improving the reconstruction performance of ESI. However, the fusion of these two modalities for the ESI problem has not been fully explored in existing deep learning frameworks. In this paper, we propose a Multi-Modal Deep Fusion (MMDF) framework for solving the ESI inverse problem, termed as MMDF-ESI. Our framework integrates both EEG and MEG in a deep learning framework with a specially designed squeeze-and-excitation module. This integration of EEG and MEG is conducted at an early phase in the deep learning framework rather than on the final decision level fusion. Our experimental results show that (1) the localization accuracy of MMDF-ESI consistently outperforms that of using a single modality; (2) MMDF-ESI exhibits excellent stability, characterized by significantly smaller error variance in source reconstruction compared to benchmark methods, particularly for sources with larger extended activation areas and under low signal-to-noise ratio (SNR) conditions; (3) the evaluation on a real EEG/MEG dataset demonstrates that MMDF-ESI enables a more concentrated reconstruction, effectively recovering an extended area of underlying source activation.
更多查看译文
关键词
brain source imaging,fusion,meg,eeg,mmdf-esi,multi-modal
AI 理解论文
溯源树
样例
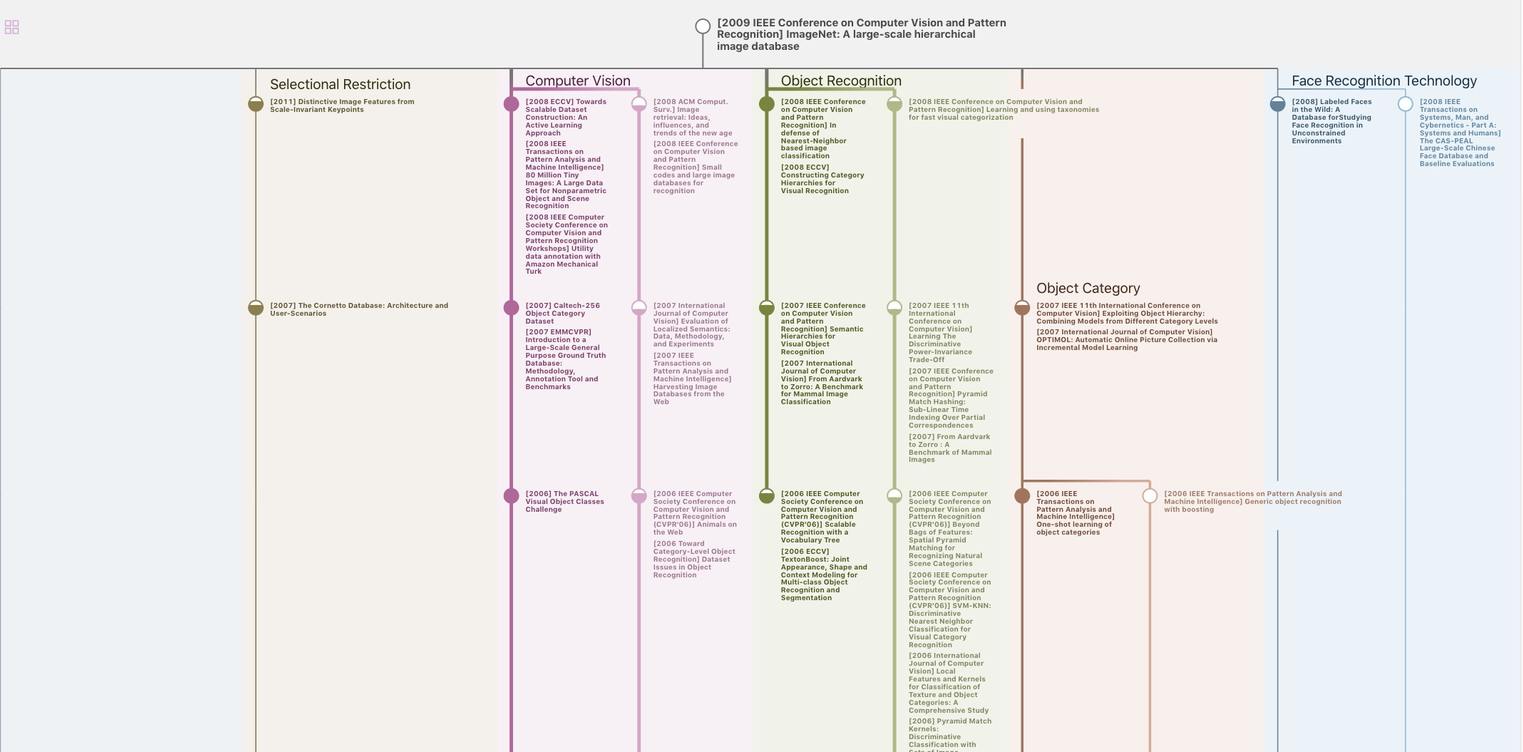
生成溯源树,研究论文发展脉络
Chat Paper
正在生成论文摘要