A Scalable Asynchronous Federated Learning for Privacy-Preserving Real-Time Surveillance Systems.
INFOCOM Workshops(2023)
摘要
A rise in popularity of decentralized Machine Learning (ML) algorithms, attributed to ensuring the privacy and security of users, has seen them being increasingly applied within the domain of healthcare, and civilian and military mission-critical applications. Federated Learning (FL) is a promising privacy-preserving approach to distributed ML paradigm that enables collaboratively training a single high-quality intelligent model on a large corpus of decentralized data without sharing the local data with the central server. Most current state-of-the-art approaches to FL are based on synchronous communication strategies. Synchronous FL systems are communication efficient but have slow learning convergence due to stranglers and high energy costs. Furthermore, synchronous FL approaches have significant drawbacks in terms of scalability and efficiency as the size and complexity of the underlying system scale up, given the heterogeneity of participating devices. In this paper, we are expanding beyond the current state-of-the-art FL approaches by experimentally exploring the use of scalable asynchronous FL for privacy-preserving real-time surveillance systems.
更多查看译文
关键词
Asynchronous,Federated Learning,Surveillance Systems,Privacy-Preserving Machine Learning
AI 理解论文
溯源树
样例
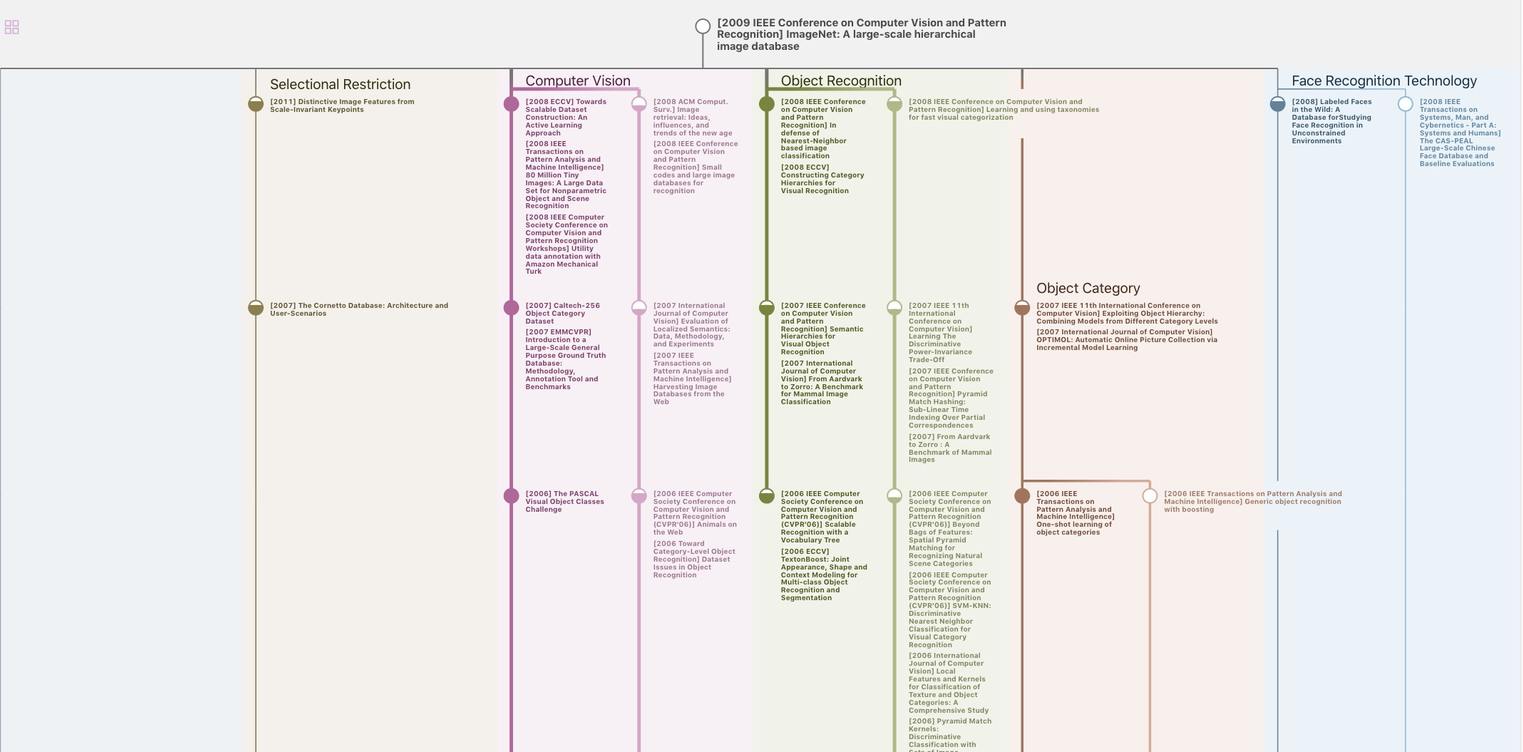
生成溯源树,研究论文发展脉络
Chat Paper
正在生成论文摘要