Spotting Deep Neural Network Vulnerabilities in Mobile Traffic Forecasting with an Explainable AI Lens.
INFOCOM(2023)
摘要
The ability to forecast mobile traffic patterns is key to resource management for mobile network operators and planning for local authorities. Several Deep Neural Networks (DNN) have been designed to capture the complex spatio-temporal characteristics of mobile traffic patterns at scale. These models are complex black boxes whose decisions are inherently hard to explain. Even worse, they have proven vulnerable to adversarial attacks which undermine their applicability in production networks. In this paper, we conduct a first in-depth study of the vulnerabilities of DNNs for large-scale mobile traffic forecasting. We propose DeExp, a new tool that leverages EXplainable Artificial Intelligence (XAI) to understand which Base Stations (BSs) are more influential for forecasting from a spatio-temporal perspective. This is challenging as existing XAI techniques are usually applied to computer vision or natural language processing and need to be adapted to the mobile network context. Upon identifying the more influential BSs, we run state-of-the art Adversarial Machine Learning (AML) techniques on those BSs and measure the accuracy degradation of the predictors. Extensive evaluations with real-world mobile traffic traces pinpoint that attacking BSs relevant to the predictor significantly degrades its accuracy across all the scenarios.
更多查看译文
关键词
deep neural network vulnerabilities,mobile traffic forecasting,explainable explainable lens
AI 理解论文
溯源树
样例
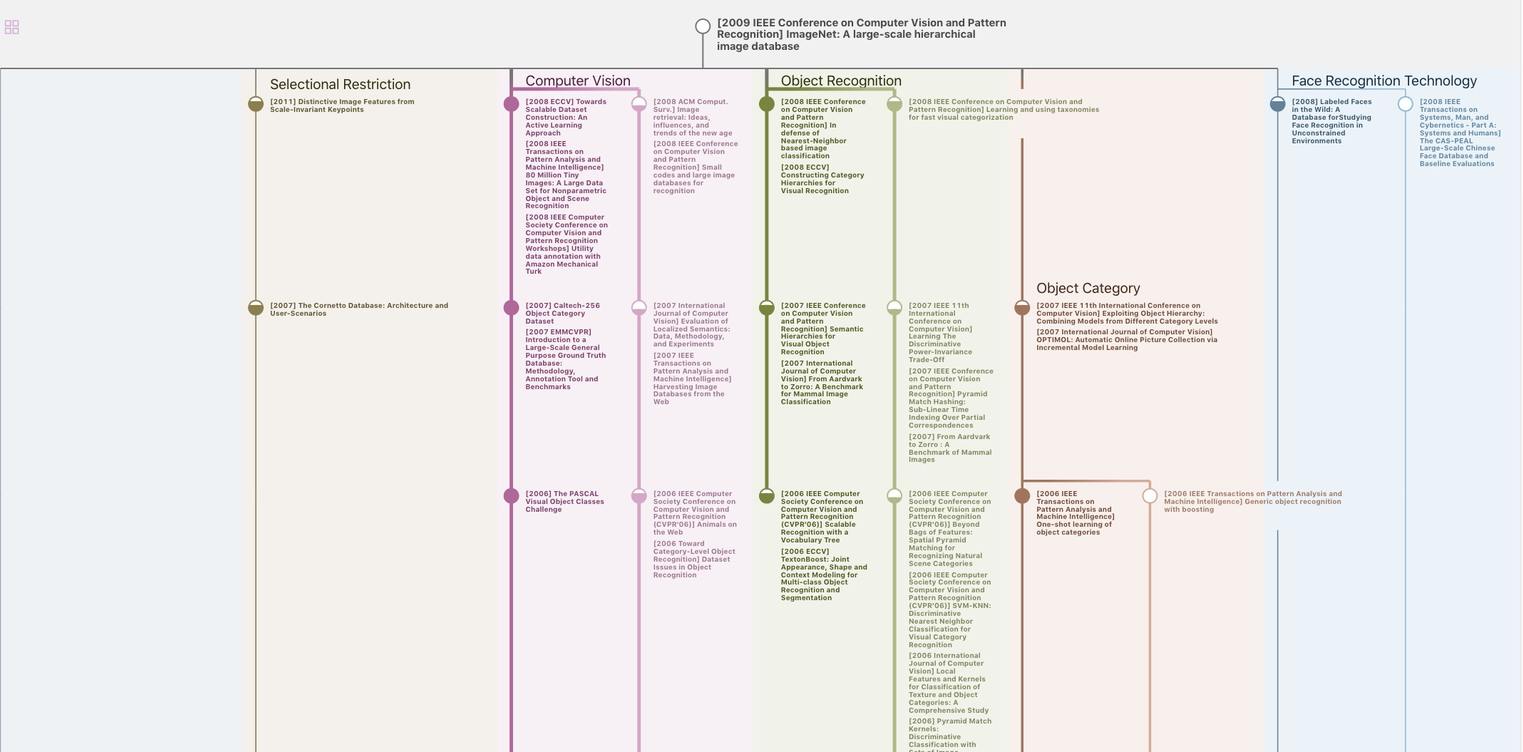
生成溯源树,研究论文发展脉络
Chat Paper
正在生成论文摘要