Rule-Enhanced Evolutional Dual Graph Convolutional Network for Temporal Knowledge Graph Link Prediction.
WISA(2023)
摘要
The aim of link prediction over temporal knowledge graphs is to discover new facts by capturing the interdependencies between historical facts. Embedding-based methods learn the interdependencies into low-dimentional vector space while rule-based methods mine logic rules that can precisely infer missing facts. However, most of the embedding-based methods divide the temporal knowledge graph into a snapshot sequence with timestamps which increases the inherent lack of information, and the structural dependency of relations is often overlooked, which is crucial for learning high-quality relation embeddings. To address these challenges, we introduce a novel Rule-enhanced Evolutional Dual Graph Convolutional Network, called RED-GCN, which leverages rule learning to enhance the density of information via inferring and injecting new facts into every snapshot, and an evolutional dual graph convolutional network is employed to capture the structural dependency of relations and the temporal dependency across adjacent snapshots. We conduct experiments on four real-world datasets. The results demonstrate that our model outperforms the baselines, and enhancing information in snapshots is beneficial to learn high-quality embeddings.
更多查看译文
关键词
knowledge,graph,prediction,rule-enhanced
AI 理解论文
溯源树
样例
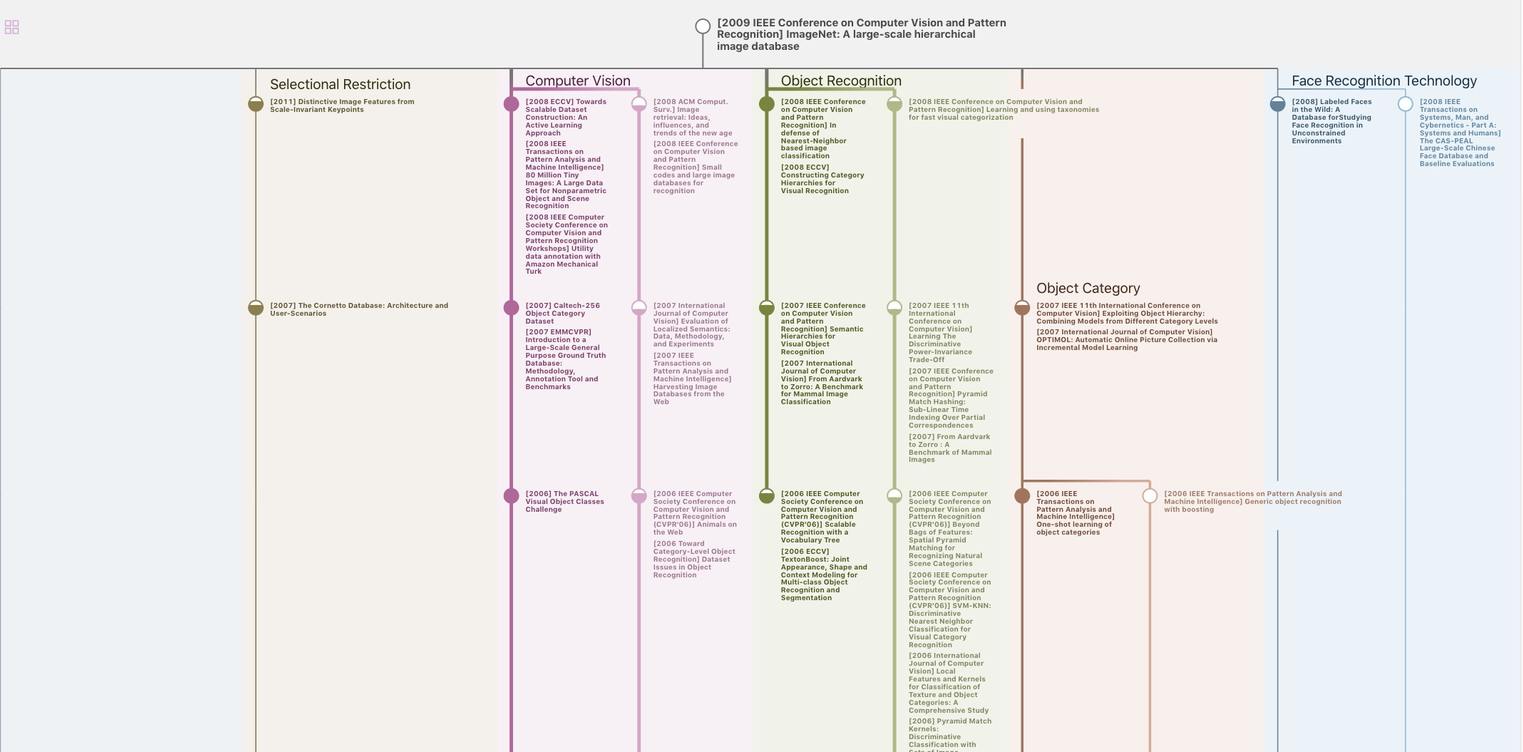
生成溯源树,研究论文发展脉络
Chat Paper
正在生成论文摘要