Disentangling Motives behind Item Consumption and Social Connection for Mutually-enhanced Joint Prediction
PROCEEDINGS OF THE 17TH ACM CONFERENCE ON RECOMMENDER SYSTEMS, RECSYS 2023(2023)
摘要
Item consumption and social connection, as common user behaviors in many web applications, have been extensively studied. However, most current works separately perform either item consumption or social link prediction tasks, possibly with the help of the other as an auxiliary signal. Moreover, they merely consider the behaviors in a holistic manner yet neglect the multi-faceted motives behind them. For example, the intention of watching a movie could be killing time or watching it with friends; Likewise, one might connect with others due to friendships or colleagues. To fill this gap, we propose to Disentangle the multi-faceted Motives in each network (i.e., the user-item interaction network and social network) defined respectively by the two types of behaviors, for mutually-enhanced Joint Prediction (DMJP). Specifically, we first learn the disentangled user representations driven by motives of multi-facets in both networks. Thereafter, the mutual influence of the two networks is subtly discriminated at the facet-to-facet level. The fine-grained mutual influence is then exploited asymmetrically to help refine user representations in both networks, with the goal of achieving a mutually-enhanced joint item and social link prediction. Empirical studies on three public datasets showcase the superiority of DMJP over state-of-the-arts (SOTAs) on both tasks.
更多查看译文
关键词
Recommender systems,Social networks,Multi-task learning,Multi-faceted consumption intentions,Multi-faceted social relations
AI 理解论文
溯源树
样例
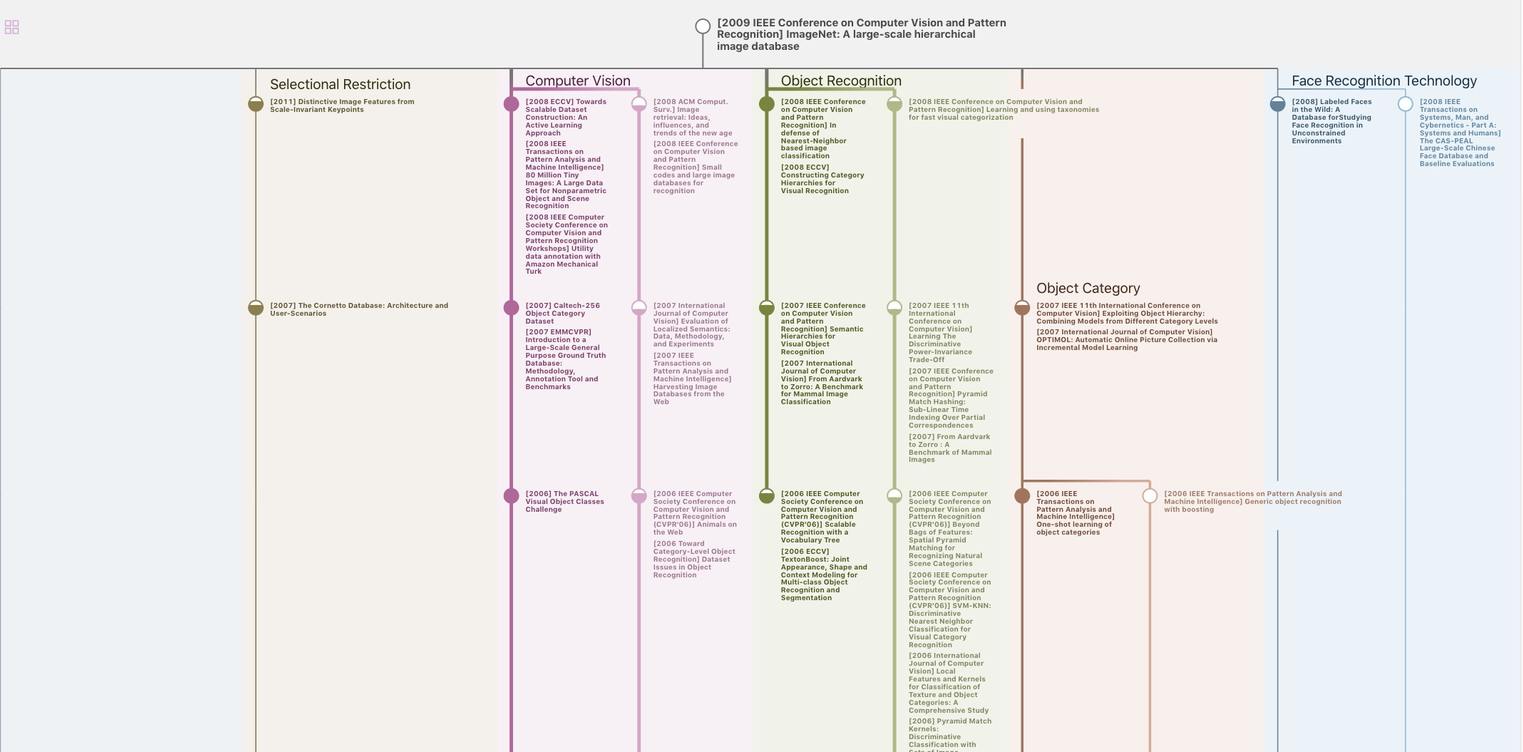
生成溯源树,研究论文发展脉络
Chat Paper
正在生成论文摘要