Using Learnable Physics for Real-Time Exercise Form Recommendations
PROCEEDINGS OF THE 17TH ACM CONFERENCE ON RECOMMENDER SYSTEMS, RECSYS 2023(2023)
摘要
Good posture and form are essential for safe and productive exercising. Even in gym settings, trainers may not be readily available for feedback. Rehabilitation therapies and fitness workouts can thus benefit from recommender systems that provide real-time evaluation. In this paper, we present an algorithmic pipeline that can diagnose problems in exercises technique and offer corrective recommendations, with high sensitivity and specificity, in real-time. We use MediaPipe for pose recognition, count repetitions using peak-prominence detection, and use a learnable physics simulator to track motion evolution for each exercise. A test video is diagnosed based on deviations from the prototypical learned motion using statistical learning. The system is evaluated on six full and upper body exercises. These real-time recommendations, counseled via low-cost equipment like smartphones, will allow exercisers to rectify potential mistakes making self-practice feasible while reducing the risk of workout injuries.
更多查看译文
关键词
real-time exercise pose recommendations,physics-inspired neural networks
AI 理解论文
溯源树
样例
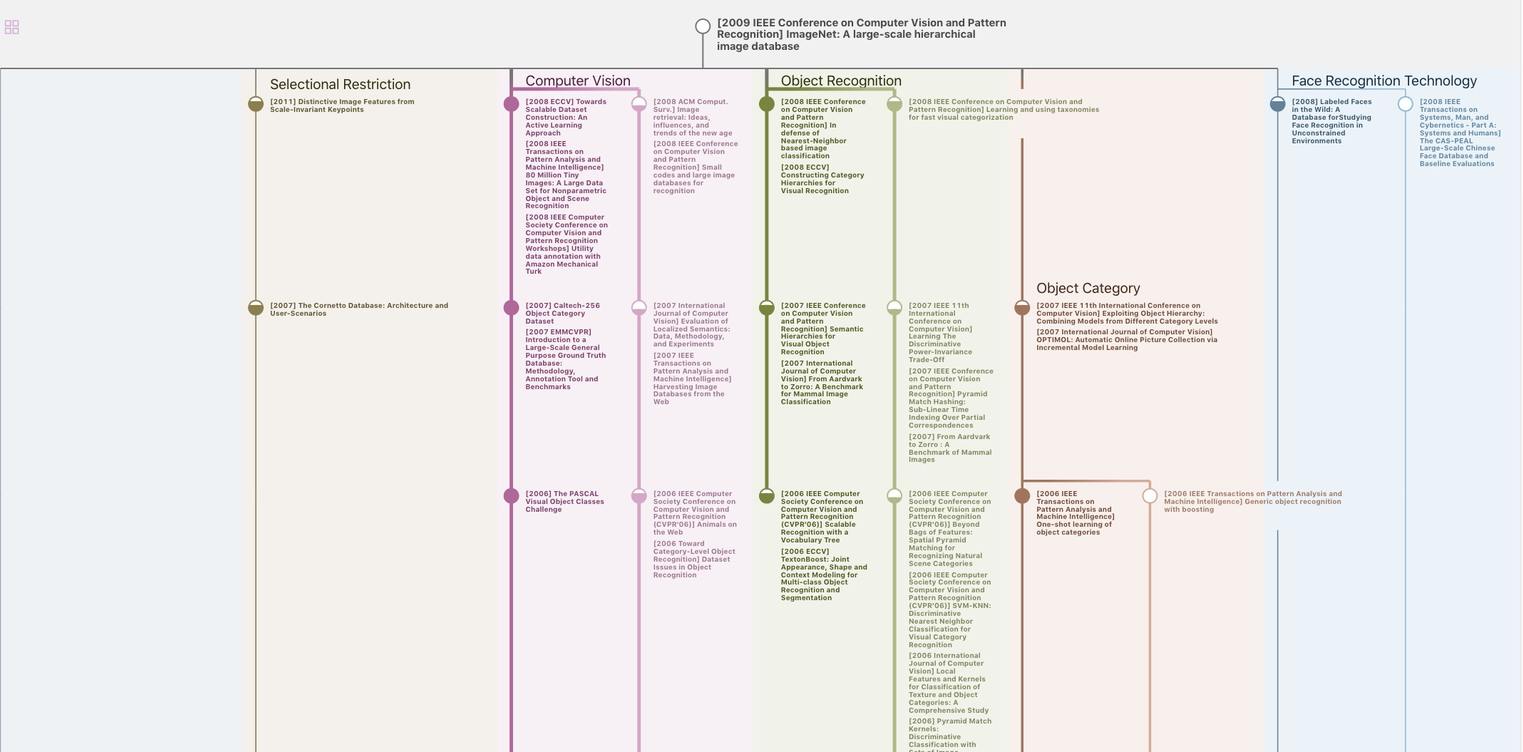
生成溯源树,研究论文发展脉络
Chat Paper
正在生成论文摘要