Efficient Fine-Tuning Large Language Models for Knowledge-Aware Response Planning
MACHINE LEARNING AND KNOWLEDGE DISCOVERY IN DATABASES: RESEARCH TRACK, ECML PKDD 2023, PT II(2023)
摘要
Large Language Models (LLMs) have shown impressive emergent language capabilities, especially in applications with high ambiguity, such as language reasoning and knowledge consolidation. However, previous work explores the use of LLMs for acquiring information using either parametric or external knowledge, which might lead to serious issues such as hallucination. Toward solving these issues, we present a novel approach of knowledge-aware response planning (KARP) and propose a novel framework that employs (i) a knowledge retriever to obtain relevant information from web documents or databases for a given user query, and (ii) a robust fine-tuning strategy for LLMs to exploit the retrieved external knowledge for planning a final response. Experimental results show that our proposed framework can provide natural, concise answers for open-domain questions with high accuracy.
更多查看译文
关键词
Knowledge-Aware Response Planning,Question Answering,Large Language Models,Fine-tuning
AI 理解论文
溯源树
样例
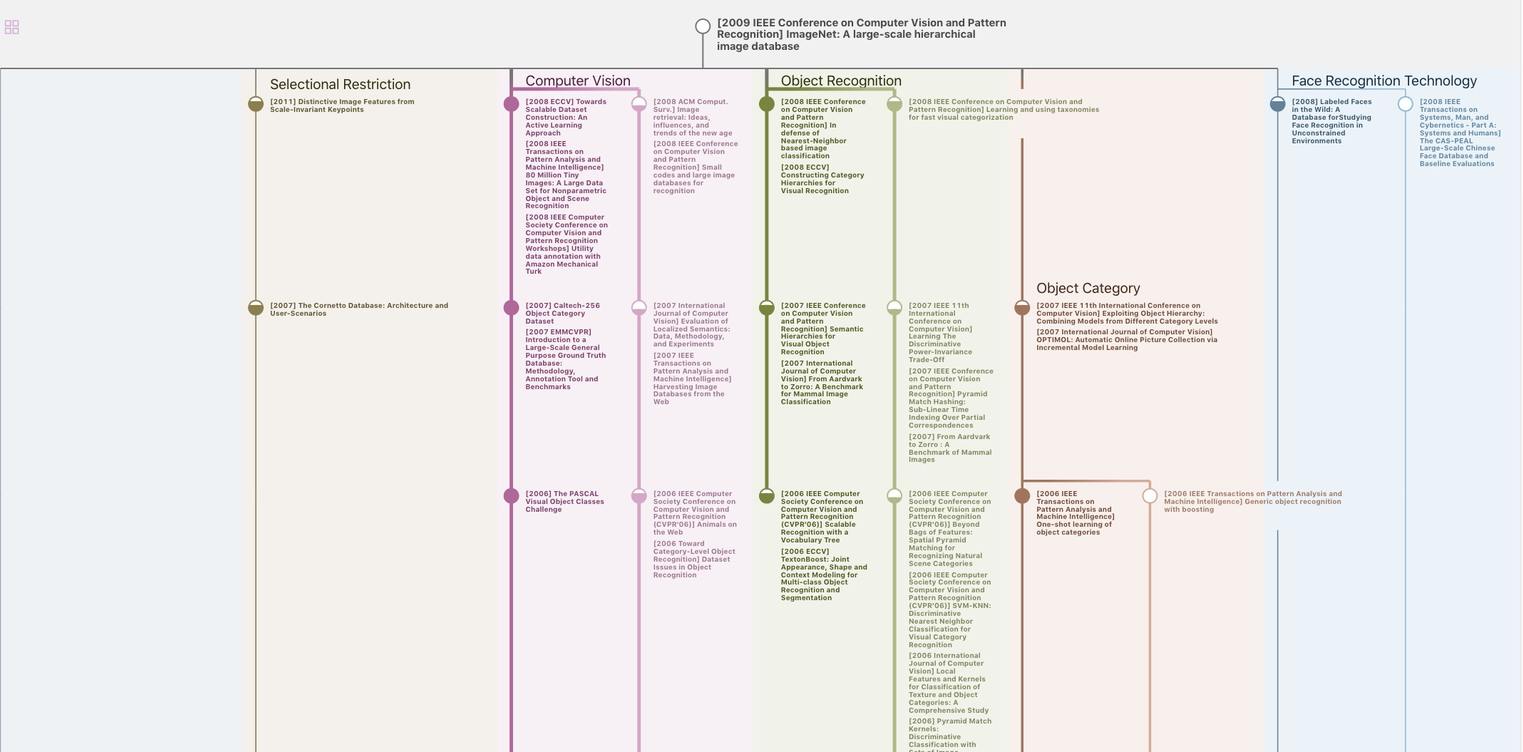
生成溯源树,研究论文发展脉络
Chat Paper
正在生成论文摘要