Online Deep Hybrid Ensemble Learning for Time Series Forecasting
MACHINE LEARNING AND KNOWLEDGE DISCOVERY IN DATABASES: RESEARCH TRACK, ECML PKDD 2023, PT V(2023)
摘要
The complex and changing nature of time series data renders forecasting one of the most challenging tasks in time series analysis. It is also commonly acknowledged that no single ML model can be perfectly appropriate for all applications. One solution to tackle this issue is to learn a heterogeneous ensemble by combining a diverse set of forecasters. In addition, ML models usually reveal a time-dependent performance. This can be explained by the fact that different models have varying regions of expertise or so-called Regions of Competence (RoCs) over the time series. In this paper, we propose a novel online deep hybrid ensemble architecture for time series forecasting. The architecture is composed of convolutional layers for learning new enriched time series representation connected to a pool of heterogeneous models composed of classical ML models and neural nets. The models are combined using a weighted average, where the weights are set in a timely adaptive manner using their pre-computed RoCs. The RoCs are computed using a gradient-based approach that maps the performance of these models to input regions in the time series and can therefore be exploited to generate saliency maps that provide suitable explanations for particular ensemble aggregation, i.e., weights setting at a particular time. The RoCs are updated in an informed manner following drift detection in the time series. An extensive empirical study on various real-world datasets demonstrates that our method achieves excellent or on-par results in comparison to the state-of-the-art approaches as well as several baselines.
更多查看译文
关键词
Ensemble Learning,Time Series Forecasting,Aggregation,Regions-of-Competence,Concept Drift,Explainability
AI 理解论文
溯源树
样例
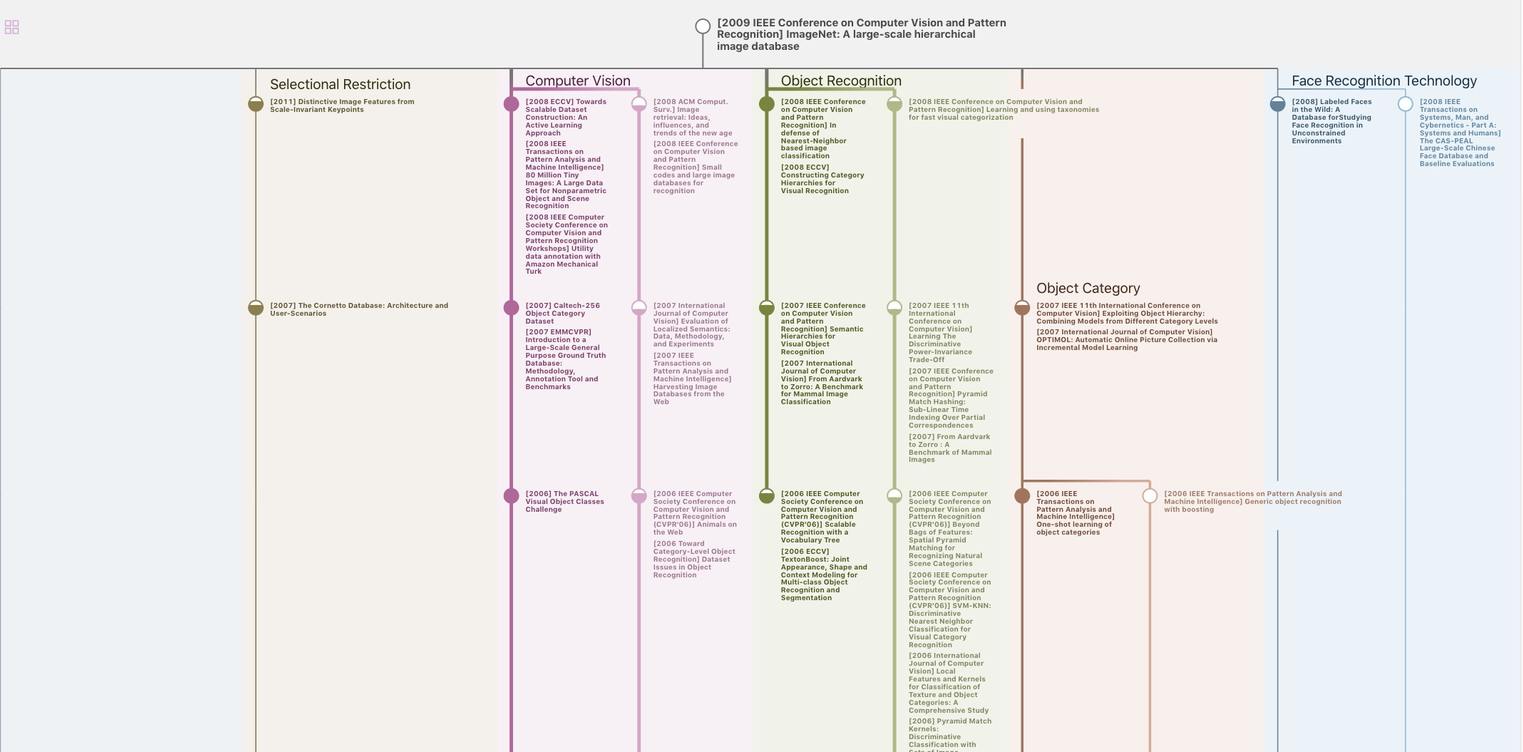
生成溯源树,研究论文发展脉络
Chat Paper
正在生成论文摘要