On the Good Behaviour of Extremely Randomized Trees in Random Forest-Distance Computation
MACHINE LEARNING AND KNOWLEDGE DISCOVERY IN DATABASES: RESEARCH TRACK, ECML PKDD 2023, PT IV(2023)
摘要
Originally introduced in the context of supervised classification, ensembles of Extremely Randomized Trees (ERT) have shown to provide surprisingly effective models also in unsupervised settings, e.g., for anomaly detection (via Isolation Forests) and for distance computation. In this paper, we focus on this latter application of ERT, namely in the context of Random Forest (RF) distance computation. We aim at narrowing the gap between the established empirical evidence of the good behaviour of ERT and the still limited theoretical understanding of their (somehow) surprisingly good performance when compared to more involved methodologies. Our main contribution is the following: we assume the existence of a proper representation on a given domain, i.e., a vectorial representation of the objects which satisfies the Compactness Hypothesis formulated by Arkadev and Braverman in 1967. Under such a hypothesis, given the "true" distance between two objects, we show how to derive a bound on the approximation guaranteed by two main RF-distances obtained by employing ensembles of ERTs, with respect to such "true" distance. In other words, we show that there exists a constant c such that if two objects are epsilon-close in the true distance, then with high probability they are (c center dot epsilon)-close in the RF-distances computed with ERT forests.
更多查看译文
关键词
Random Forest-distances,Extremely Randomized Trees,Compactness hyphotesis
AI 理解论文
溯源树
样例
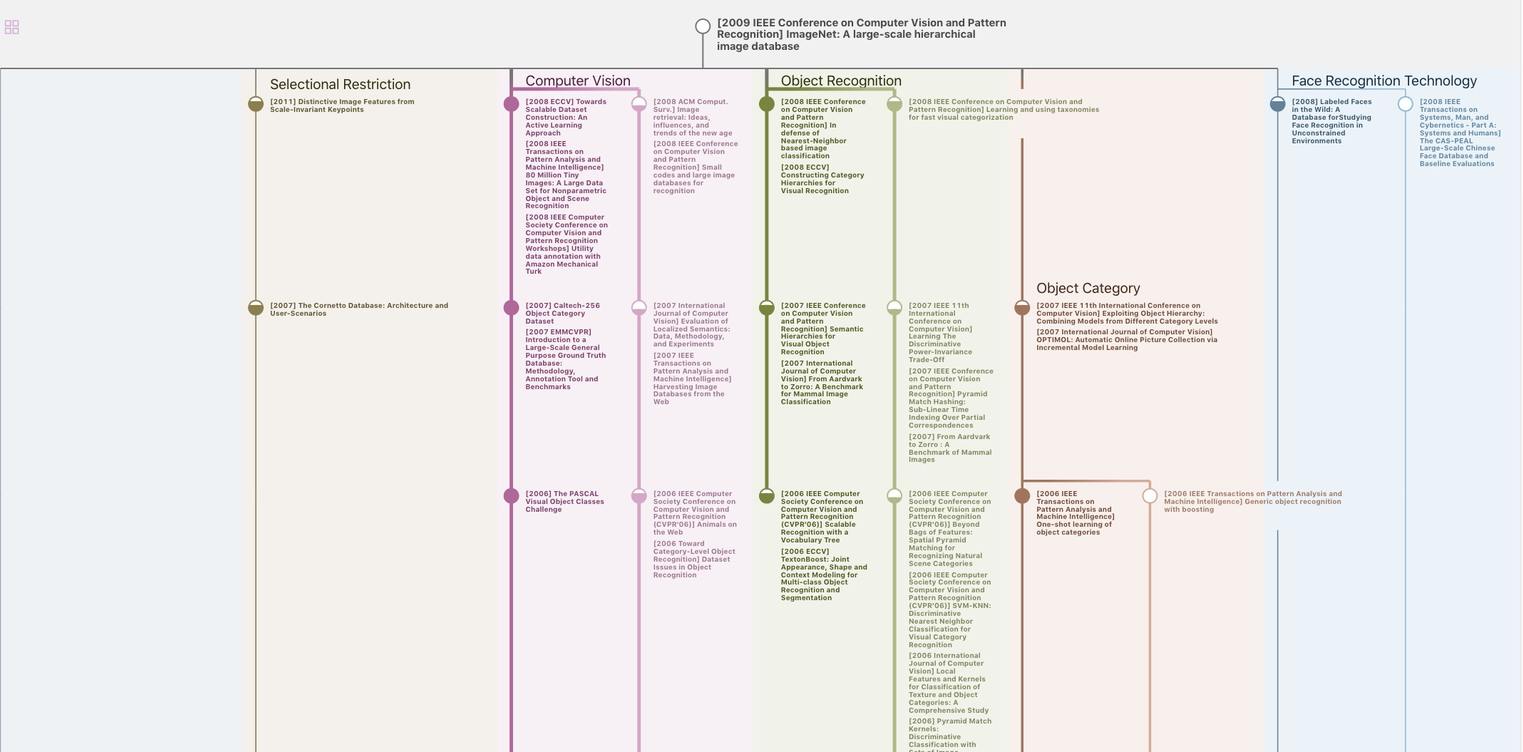
生成溯源树,研究论文发展脉络
Chat Paper
正在生成论文摘要