Spatial-Temporal Graph Sandwich Transformer for Traffic Flow Forecasting
MACHINE LEARNING AND KNOWLEDGE DISCOVERY IN DATABASES: APPLIED DATA SCIENCE AND DEMO TRACK, ECML PKDD 2023, PT VII(2023)
摘要
Traffic flow forecasting has primarily relied on the spatial-temporal models. However, yielding accurate traffic prediction is still challenging due to that the dynamic temporal pattern, intricate spatial dependency and their affluent interaction are difficult to depict. Existing models are often restricted since they can only capture limited-range temporal dependency, shallow spatial dependency, or faint spatial-temporal interaction. In this work, to overcome these limitations, we propose a novel spatial-temporal graph sandwich Transformer (STGST) for traffic flow forecasting. In STGST, we design two temporal Transformers equipped with time encoding and a spatial Transformer equipped with structure and spatial encoding to characterize long-range temporal and deep spatial dependencies, respectively. These two types of Transformers are further structured in a sandwich manner with two temporal Transformers as buns and a spatial Transformer as sliced meat to capture prosperous spatial-temporal interactions. We also assemble a set of such sandwich Transformers together to strengthen the correlations between spatial and temporal domains. Extensive experimental studies are performed on public traffic benchmarks. Promising results demonstrate that the proposed STGST outperforms state-of-the-art baselines.
更多查看译文
关键词
Spatial-temporal graph,Transformer,Traffic forecasting
AI 理解论文
溯源树
样例
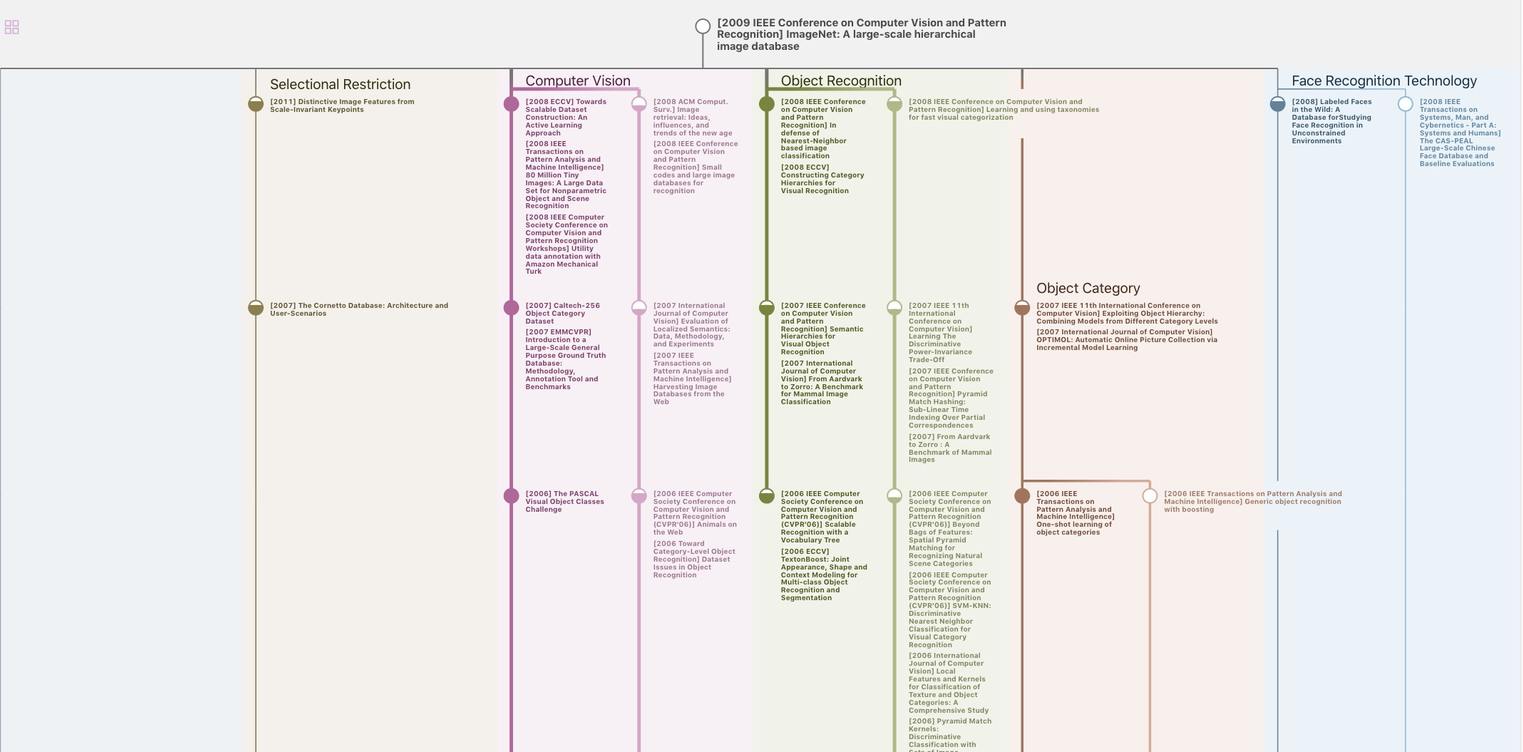
生成溯源树,研究论文发展脉络
Chat Paper
正在生成论文摘要