Filtered Observations for Model-Based Multi-agent Reinforcement Learning
MACHINE LEARNING AND KNOWLEDGE DISCOVERY IN DATABASES: RESEARCH TRACK, ECML PKDD 2023, PT IV(2023)
摘要
Reinforcement learning (RL) pursues high sample efficiency in practical environments to avoid costly interactions. Learning to plan with a world model in a compact latent space for policy optimization significantly improves sample efficiency in single-agent RL. Although world model construction methods for single-agent can be naturally extended, existing multi-agent schemes fail to acquire world models effectively as redundant information increases rapidly with the number of agents. To address this issue, we in this paper leverage guided diffusion to filter this noisy information, which harms teamwork. Obtained purified global states are then used to build a unified world model. Based on the learned world model, we denoise each agent observation and plan for multi-agent policy optimization, facilitating efficient cooperation. We name our method UTOPIA, a model-based method for cooperative multi-agent reinforcement learning (MARL). Compared to strong model-free and model-based baselines, our method shows enhanced sample efficiency in various testbeds, including the challenging StarCraft Multi-Agent Challenge tasks.
更多查看译文
关键词
Model-based planning,Multi-agent reinforcement learning,Generative models
AI 理解论文
溯源树
样例
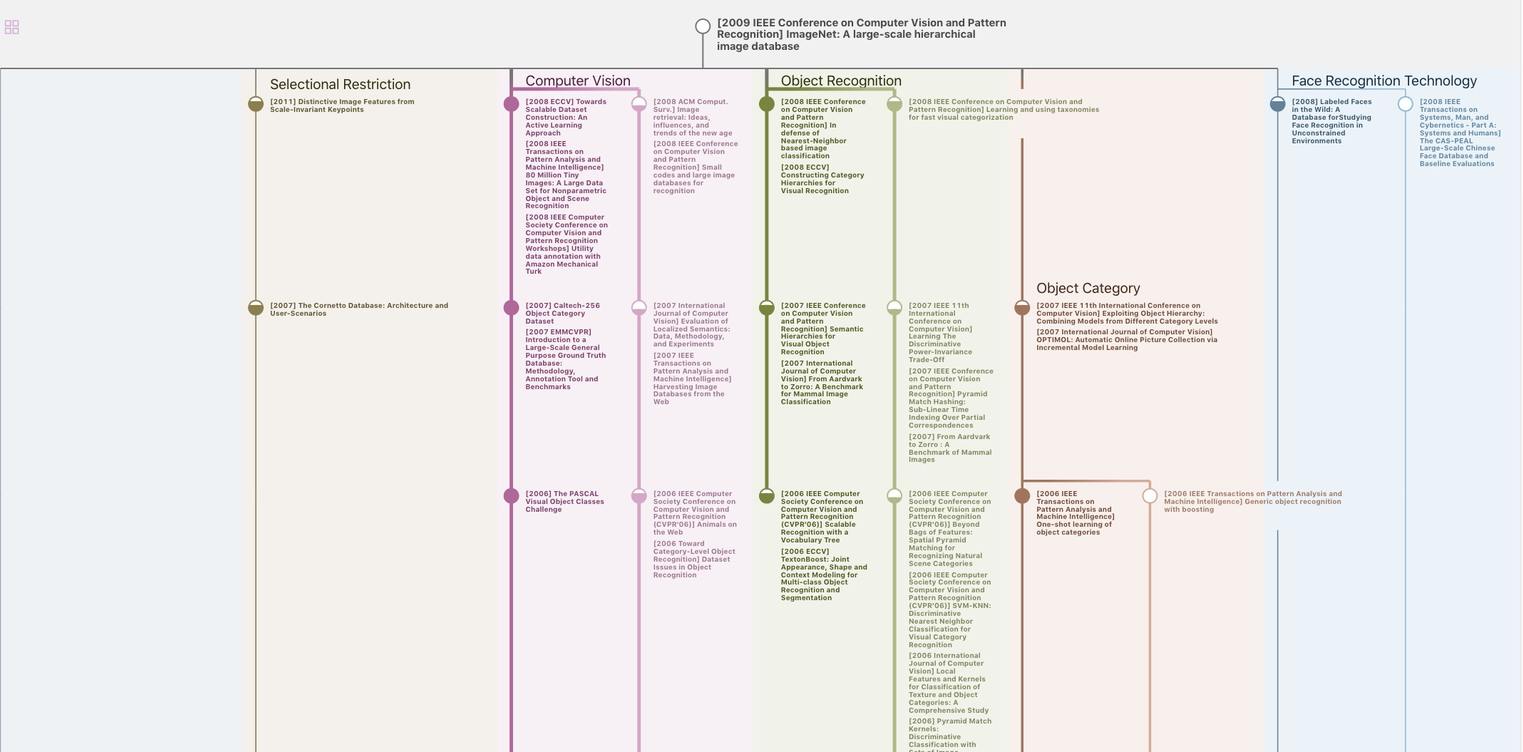
生成溯源树,研究论文发展脉络
Chat Paper
正在生成论文摘要