BipNRL: Mutual Information Maximization on Bipartite Graphs for Node Representation Learning
MACHINE LEARNING AND KNOWLEDGE DISCOVERY IN DATABASES: RESEARCH TRACK, ECML PKDD 2023, PT IV(2023)
摘要
Unsupervised representation learning on bipartite graphs is of particular interest due to the lack of explicit labels and its application in a variety of domains. Several unsupervised representation learning methods make use of mutual information maximization between node-level and graph/sub-graph-level information. We argue that such methods are better suited to graph level tasks than node level tasks because local information in node representation is dominated by graph level information. Additionally, current approaches rely on building a global summary vector in which information diminishes as the graph scales. In this work, we concentrate on optimizing representations for node-level downstream tasks such as node classification/regression. The Bipartite Node Representation Learning (BipNRL) method, which aims to learn the node embeddings by maximizing MI between the node's representation and self features without using summary vector is introduced. Furthermore, we propose the decoupled graph context loss on projected graphs to preserve the bipartite graph structure, enhancing representation for node-level tasks. Finally, we utilize attention-based neighborhood aggregation and modify the neighbourhood sampling algorithm to account for the high variance of node degree that is common in bipartite graphs. Extensive experiments show that BipNRL achieves cutting-edge results on multiple downstream tasks across diverse datasets.
更多查看译文
关键词
Node Representation Learning,Bipartite Graph
AI 理解论文
溯源树
样例
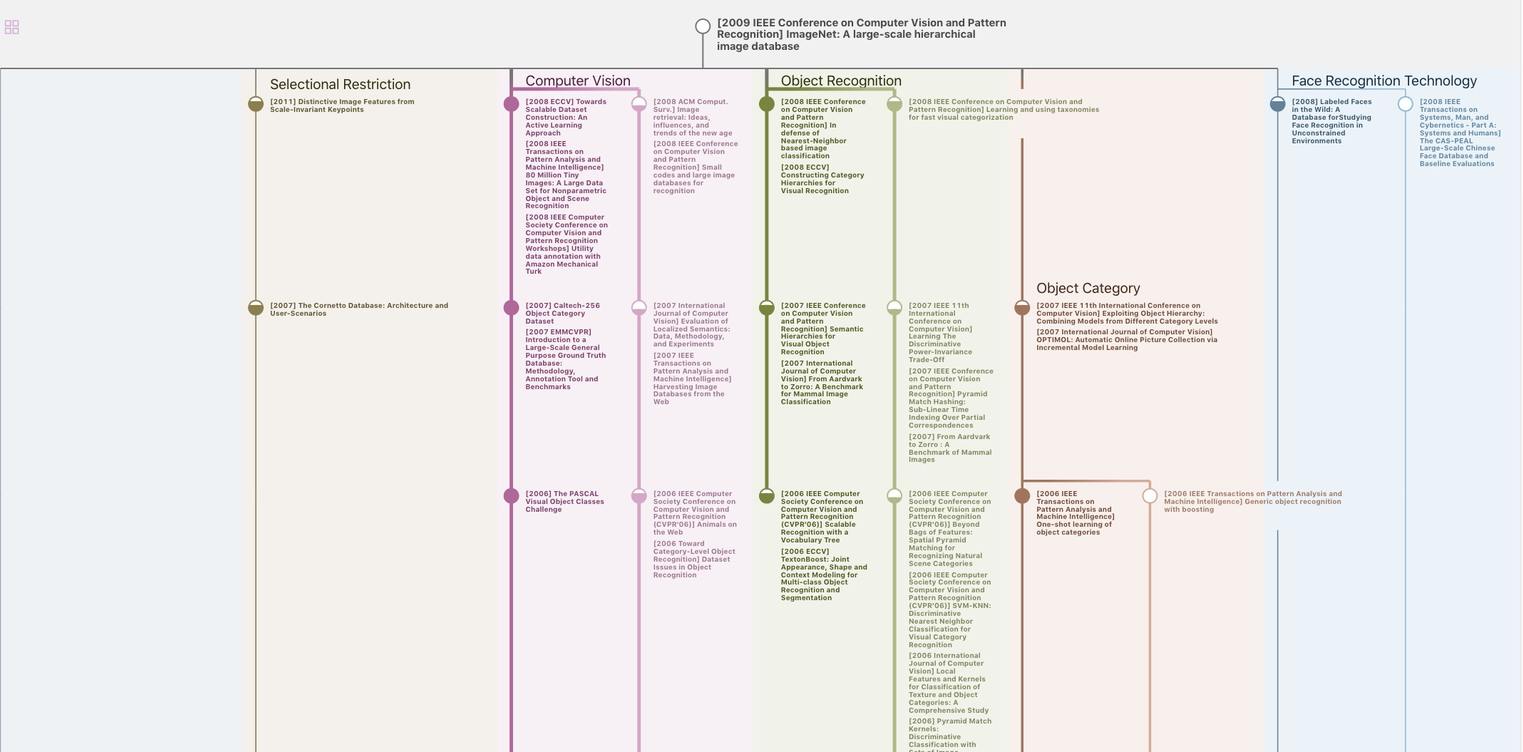
生成溯源树,研究论文发展脉络
Chat Paper
正在生成论文摘要