Semi-Supervised Social Bot Detection with Initial Residual Relation Attention Networks
MACHINE LEARNING AND KNOWLEDGE DISCOVERY IN DATABASES: APPLIED DATA SCIENCE AND DEMO TRACK, ECML PKDD 2023, PT VI(2023)
摘要
Social bot detection is a challenging task and receives extensive attention in social security. Previous researches for this task often assume the labeled samples are abundant, which neglects the fact that labels of social bots are usually hard to derive from the real world. Meanwhile, graph neural networks (GNNs) have recently been applied to bot detection. Whereas most GNNs are based on the homophily assumption, where nodes of the same type are more likely to connect to each other. So methods relying on these two assumptions will degrade while encountering graphs with heterophily or lack of labeled data. To solve these challenges above, we analyze human-bot networks and propose SIRAN, which combines relation attention with initial residual connection to reduce and prevent the noise aggregated from neighbors to improve the capability of distinguishing different kinds of nodes on social graphs with heterophily. Then we use a consistency loss to boost the detection performance of the model for limited annotated data. Extensive experiments on two publicly available and independent social bot detection datasets illustrate SIRAN achieves state-of-the-art performance. Finally, further studies demonstrate the effectiveness of our model as well. We have deployed SIRAN online: https://botdetection.aminer.cn/robotmain.
更多查看译文
关键词
social networks,social bot detection,heterophily-aware attention,semi-supervised learning
AI 理解论文
溯源树
样例
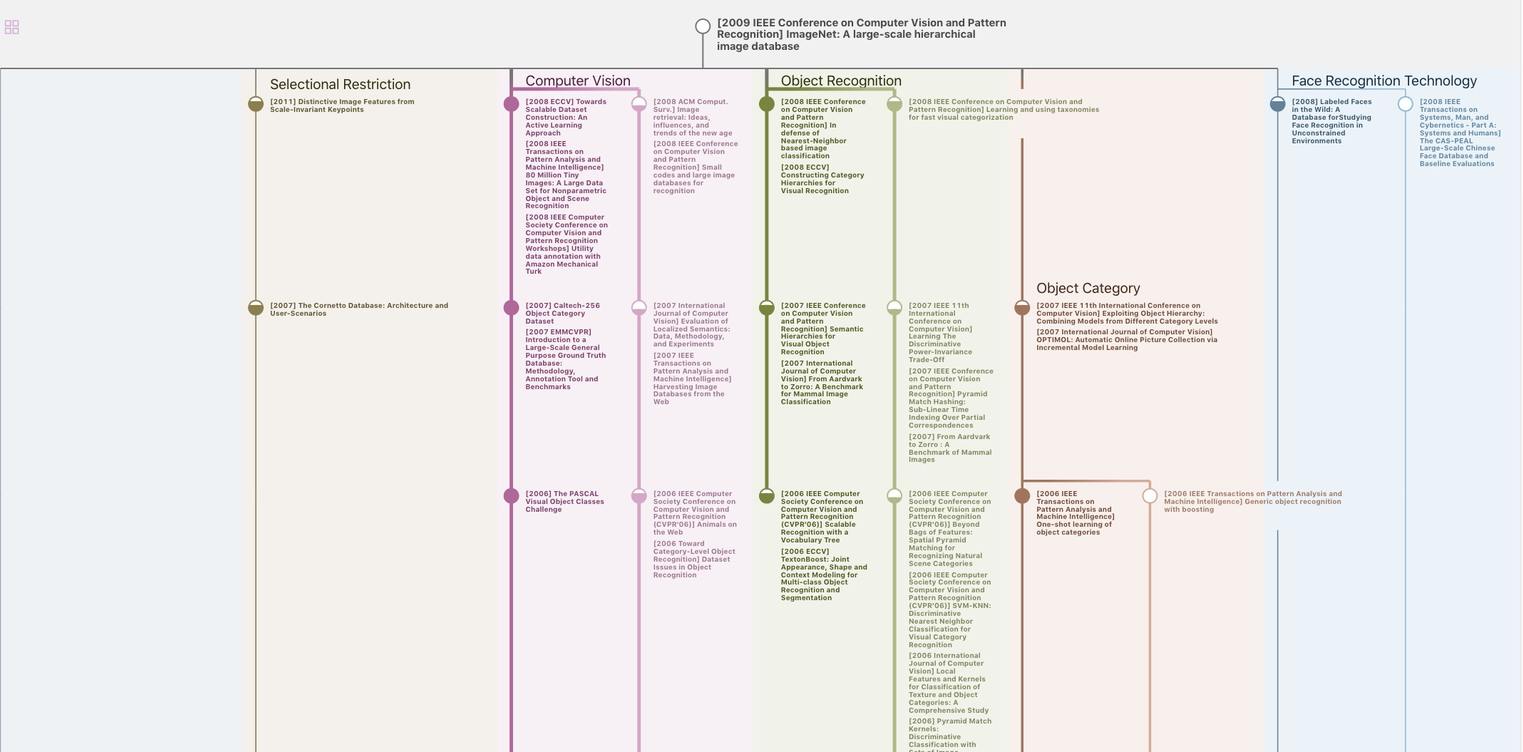
生成溯源树,研究论文发展脉络
Chat Paper
正在生成论文摘要