ConGCN: Factorized Graph Convolutional Networks for Consensus Recommendation
MACHINE LEARNING AND KNOWLEDGE DISCOVERY IN DATABASES: RESEARCH TRACK, ECML PKDD 2023, PT IV(2023)
摘要
An essential weakness of existing personalized recommender systems is that the learning is biased and dominated by popular items and users. Existing methods, particularly graph-based approaches, primarily focus on the "heterogeneous interaction" between user-item, leading to a disproportionately large influence of popular nodes during the graph learning process. Recently, popularity debiasing models have been proposed to address this issue, but they excessively concentrate on considering cause-effect or re-weighting the item/user popularity. These approaches artificially alter the nature of the data, inadvertently down-playing the representation learning of popular items/users. Consequently, balancing the trade-off between global recommendation accuracy and unpopular items/users exposure is challenging. In this paper, we advocate the concept of "homogeneous effect" from both user and item perspectives to explore the intrinsic correlation and alleviate the bias effects. Our core theme is to simultaneously factorize user-item interactions, user-user similarity, and item-item correlation, thereby learning balanced representations for items and users. To pursue well-balanced representations, we propose a Consensus factorized Graph Convolution neural Network (ConGCN), which leverages graph-based nonlinear representation learning and manifold constraints to regulate the embedding learning. An inherent advantage of ConGCN is its consensus optimization nature, where item-item and user-user relationships ensure that unpopular items are well preserved in the embedding space. The experiments on four real-world datasets demonstrate that ConGCN outperforms existing single-task-oriented methods on two typical tasks with opposite goals (global recommendation and popularity debiasing recommendation), indicating that our model can perform a balanced recommendation with both higher global and debiasing recommendation accuracy with greater long-tail item/user exposure.
更多查看译文
关键词
Recommender System,Graph Neural Networks,Consensus Learning,Popularity Bias
AI 理解论文
溯源树
样例
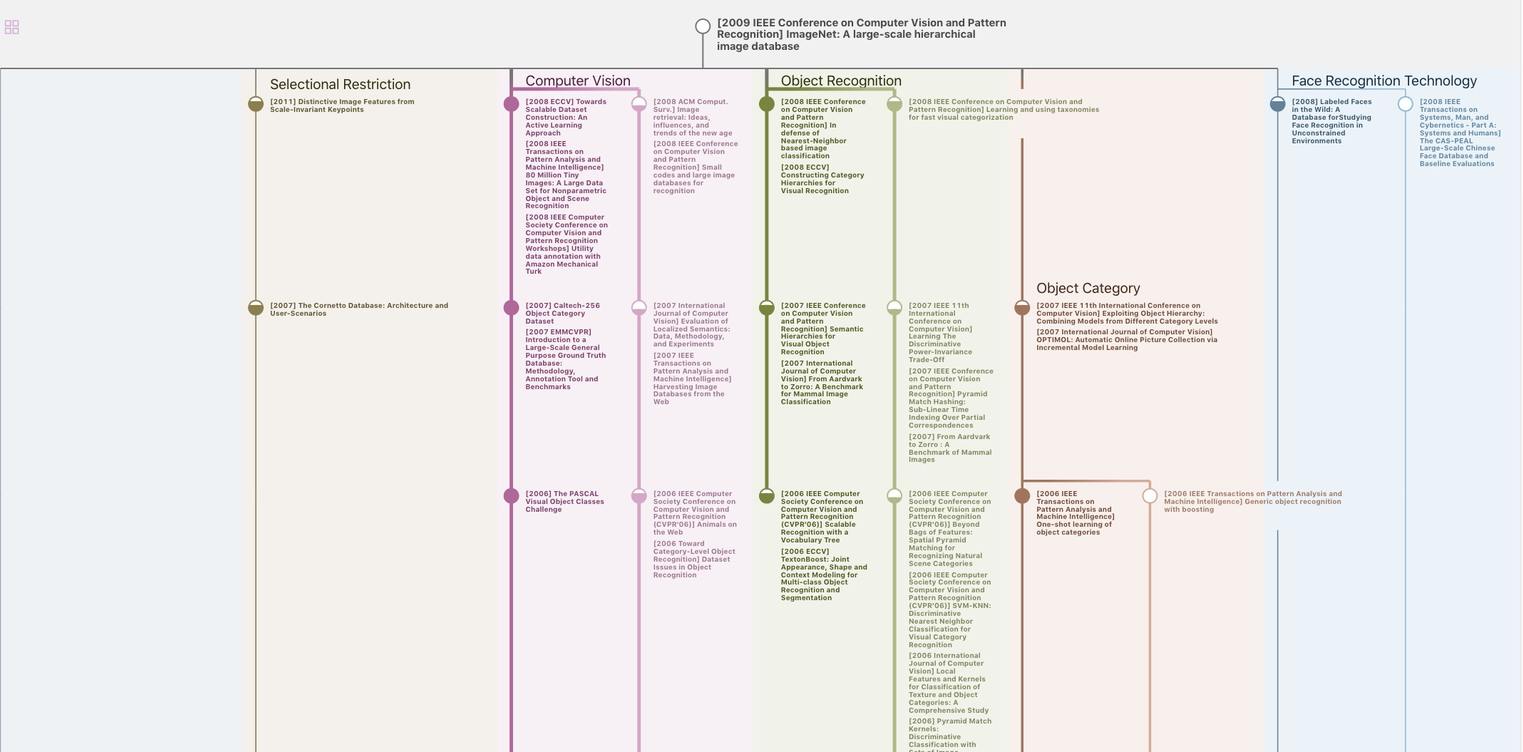
生成溯源树,研究论文发展脉络
Chat Paper
正在生成论文摘要