Safety and Robustness for Deep Neural Networks: An Automotive Use Case
COMPUTER SAFETY, RELIABILITY, AND SECURITY, SAFECOMP 2023 WORKSHOPS(2023)
摘要
Current automotive safety standards are cautious when it comes to utilizing deep neural networks in safety-critical scenarios due to concerns regarding robustness to noise, domain drift, and uncertainty quantification. In this paper, we propose a scenario where a neural network adjusts the automated driving style to reduce user stress. In this scenario, only certain actions are safety-critical, allowing for greater control over the model's behavior. To demonstrate how safety can be addressed, we propose a mechanism based on robustness quantification and a fallback plan. This approach enables the model to minimize user stress in safe conditions while avoiding unsafe actions in uncertain scenarios. By exploring this use case, we hope to inspire discussions around identifying safety-critical scenarios and approaches where neural networks can be safely utilized. We see this also as a potential contribution to the development of new standards and best practices for the usage of AI in safety-critical scenarios. The work done here is a result of the TEACHING project, an European research project around the safe, secure and trustworthy usage of AI.
更多查看译文
关键词
recurrent neural networks,adversarial robustness,human-in-the-loop,automotive,dependability
AI 理解论文
溯源树
样例
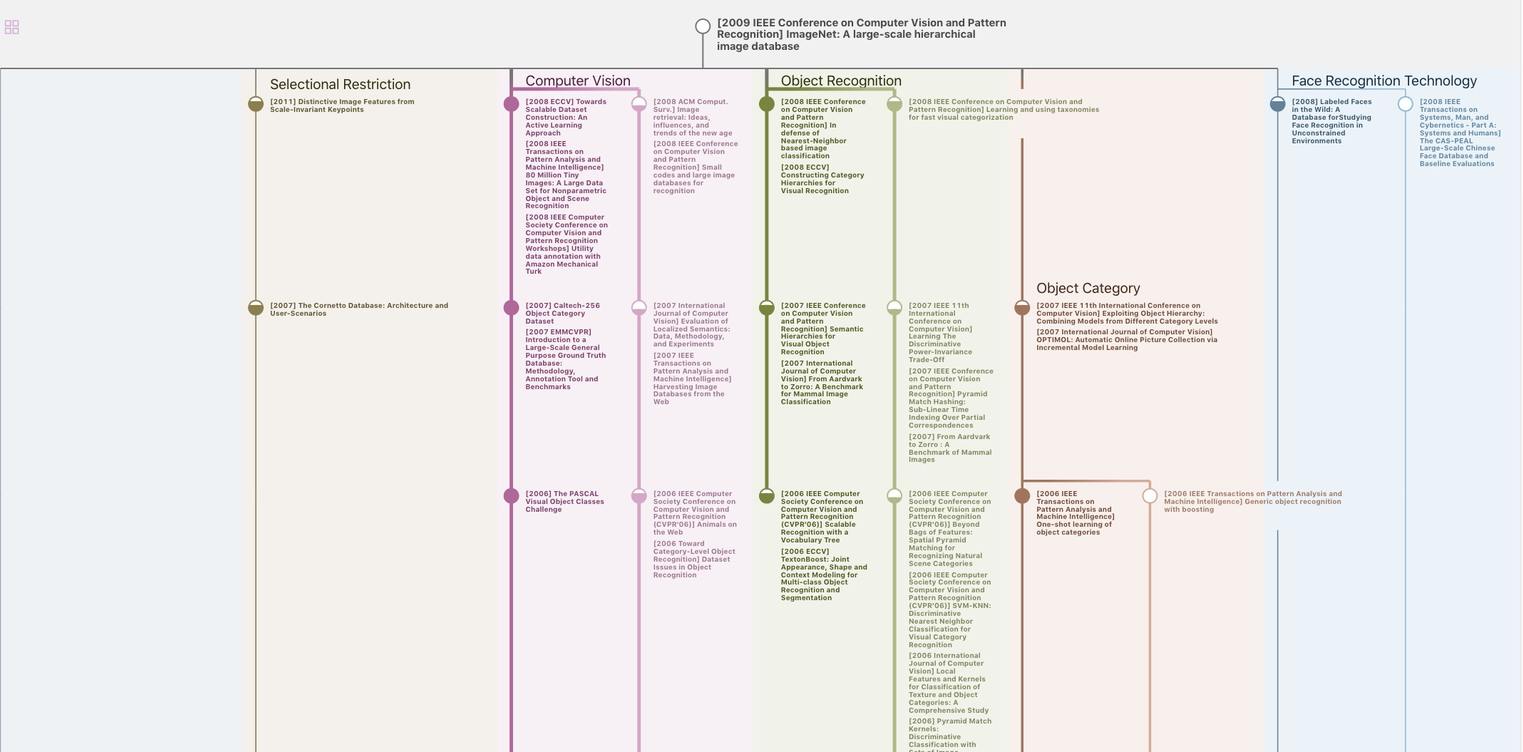
生成溯源树,研究论文发展脉络
Chat Paper
正在生成论文摘要