Interpreting Predictive Models through Causality: A Query-Driven Methodology.
FLAIRS(2023)
摘要
Machine learning algorithms have been widely adopted in recent years due to their efficiency and versatility across many fields. However, the complexity of predictive models has led to a lack of interpretability in automatic decision-making. Recent works have improved general interpretability by estimating the contributions of input features to the prediction of a pre-trained model. Despite these advancements, practitioners still seek to gain causal insights into the underlying data-generating mechanisms. To this end, some works have attempted to integrate causal knowledge into interpretability, as non-causal techniques can lead to paradoxical explanations. These efforts have provided answers to various queries, but relying on a single pre-trained model may result in quantification problems. In this paper, we argue that each causal query requires its own reasoning; thus, a single predictive model is not suited for all questions. Instead, we propose a new framework that prioritizes the query of interest and then derives a query-driven methodology accordingly to the structure of the causal model. It results in a tailored predictive model adapted to the query and an adapted interpretability technique. Specifically, it provides a numerical estimate of causal effects, which allows for accurate answers to explanatory questions when the causal structure is known.
更多查看译文
关键词
causality,predictive models,methodology,query-driven
AI 理解论文
溯源树
样例
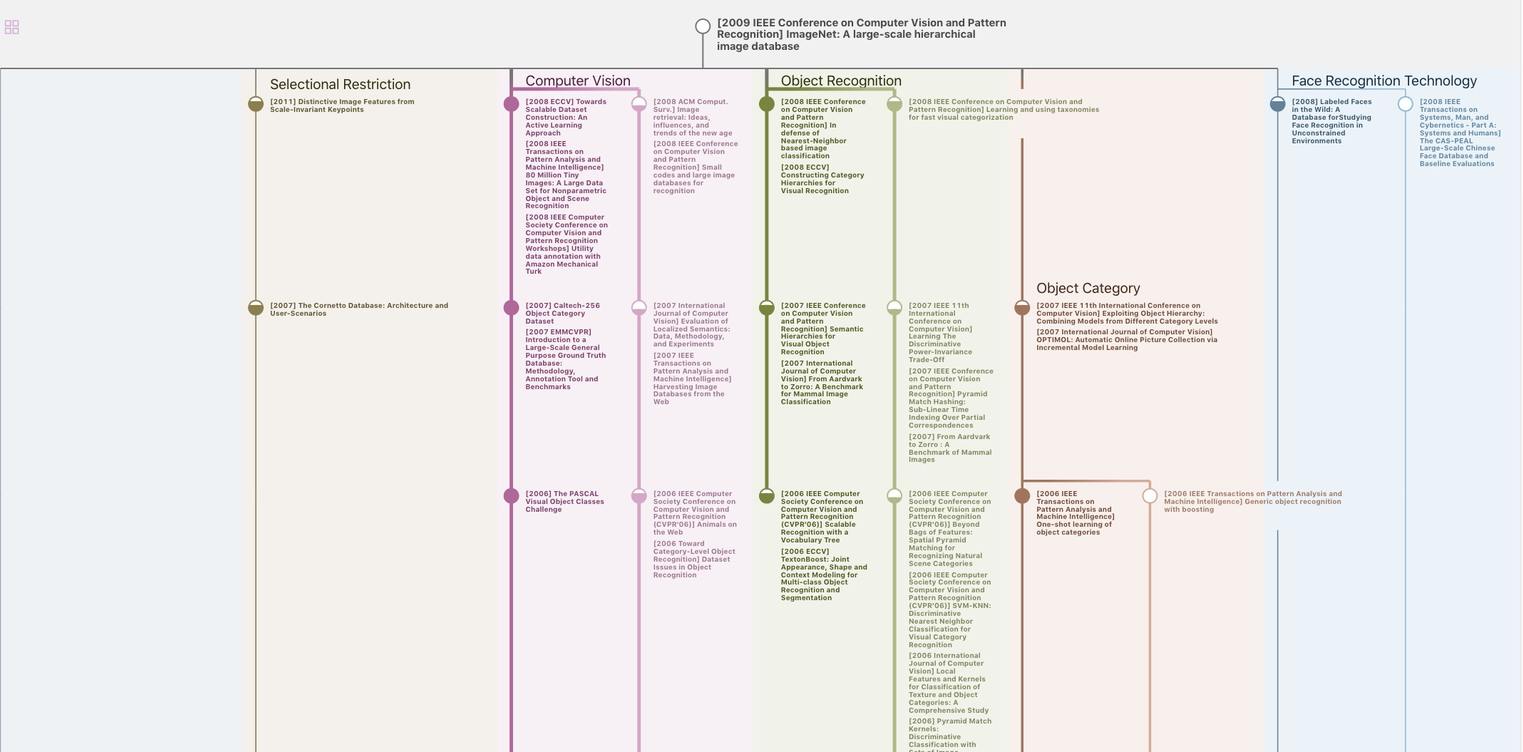
生成溯源树,研究论文发展脉络
Chat Paper
正在生成论文摘要