Dual-Modality Grading of Keratoconus Severity Based on Corneal Topography and Clinical Indicators
OPHTHALMIC MEDICAL IMAGE ANALYSIS, OMIA 2023(2023)
摘要
Keratoconus (KC) is a blinding eye disease characterized by corneal dilation, thinning, and conical protrusion. Currently, most studies just focus on KC screening and use only one modality. In this paper, we utilize two modalities of data, corneal topography and clinical indicators, to grade KC severity as normal, mild, moderate, and severe, rather than screening. Considering the data characteristics of each modality, we model their data in a targeted manner. For corneal topography, a Global Feature Extraction (GFE) block based on Self-Attention is designed as a parallel branch to obtain global information. In addition, a Feature Fusion (FF) module is proposed to better fuse local and global information. For clinical indicators, we utilize a tree model to grade KC and remove redundant information through feature selection. Finally, a trainable fusion model is used to make the final decision. Experimental results show that the proposed method outperforms all the competing methods and achieves 94.47% for weighted recall, improving the accuracy of KC grading effectively.
更多查看译文
关键词
keratoconus,dual-modality,fusion strategy
AI 理解论文
溯源树
样例
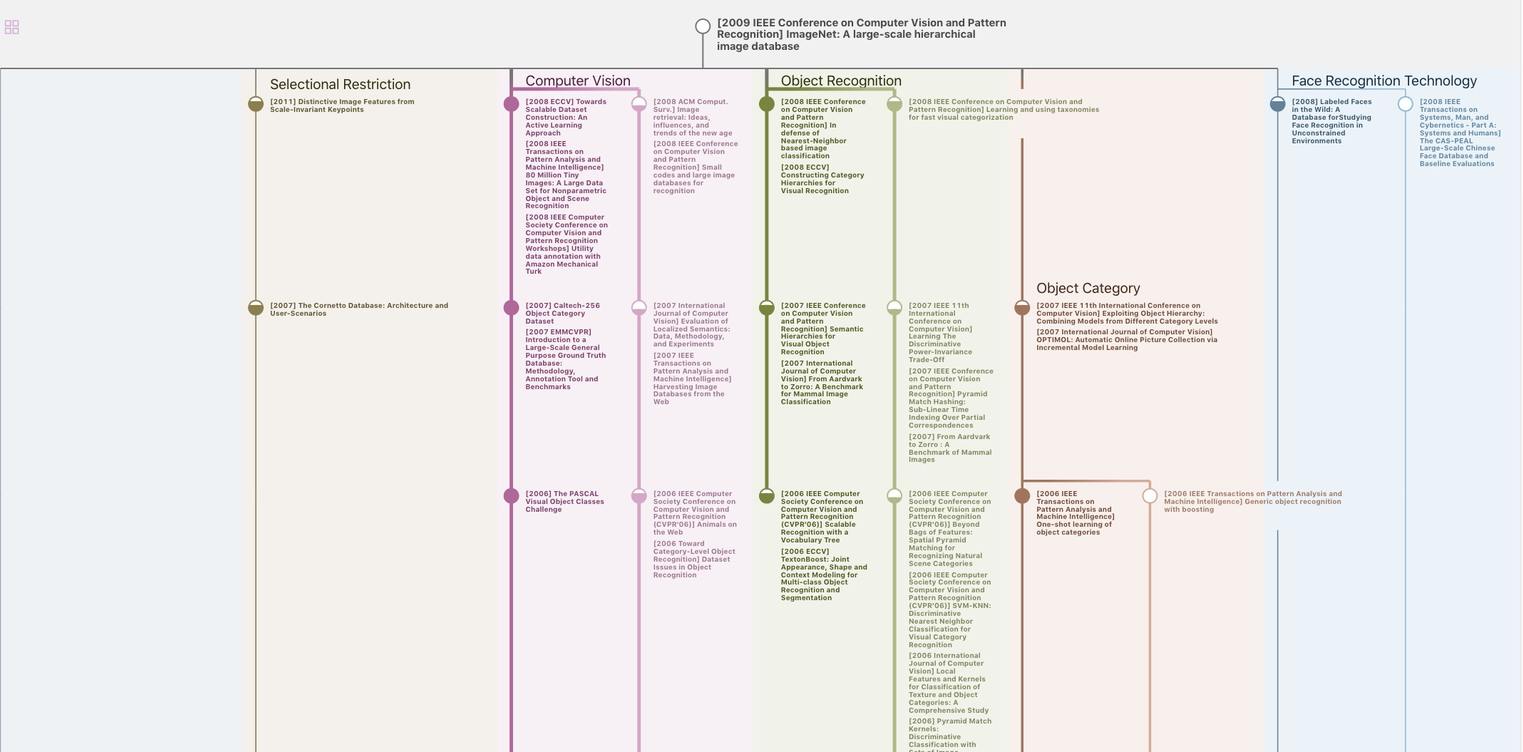
生成溯源树,研究论文发展脉络
Chat Paper
正在生成论文摘要