Multi-relational Graph Convolutional Neural Networks for Carotid Artery Stenosis Diagnosis via Fundus Images
OPHTHALMIC MEDICAL IMAGE ANALYSIS, OMIA 2023(2023)
摘要
The accumulation of carotid plaque leads to carotid artery stenosis, which in turn increases the risk of cerebrovascular disease. Noninvasive diagnosis of carotid stenosis using fundus images offers a promising approach. However, the challenge lies in extracting relevant features from these images, as convolutional neural networks(CNNs) or Transformers, which focus solely on individual images, fail to consider the interdependencies between them, leading to limited diagnostic accuracy. To address this issue, we propose a novel and effective network by combining CNNs and multi-relational graph convolutional neural networks(MGCNs). Firstly, we feed the input images into four distinct branches, which consist of CNNs or Transformers, with each branch associated with a particular relation. This process generates unique feature vectors for each branch. Secondly, we construct a multi-graph for the four kinds of clinical data, such as gender, age, sex and pid, to obtain four adjacency matrices. Finally, the feature vectors and the corresponding four adjacency matrices are input into the graph convolutional network layer respectively to obtain the prediction features, and then the prediction results are obtained through the fully connected layer. Experiments are carried out on a private dataset and the results demonstrate that the accuracy of the proposed algorithm is 10%-20% higher than that of the comparison model. Our code is available at https://github.com/momoyrz/Carotid-stenosis.
更多查看译文
关键词
Carotid artery stenosis,Fundus image classification,Multi-relational Graph Convolutional Neural Network
AI 理解论文
溯源树
样例
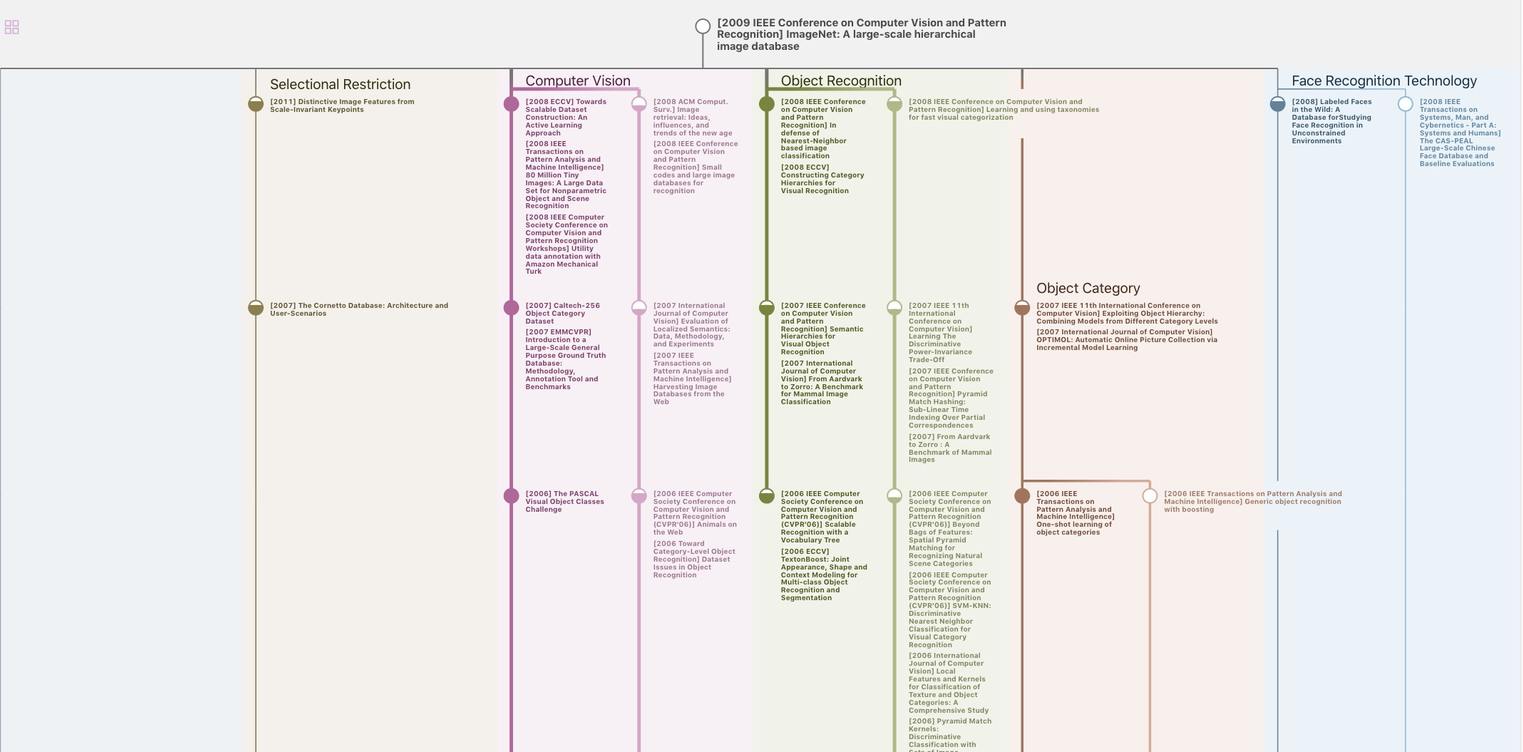
生成溯源树,研究论文发展脉络
Chat Paper
正在生成论文摘要