Fall Detection with Event-Based Data: A Case Study
COMPUTER ANALYSIS OF IMAGES AND PATTERNS, CAIP 2023, PT II(2023)
摘要
Fall detection systems are relevant in our aging society aiming to support efforts towards reducing the impact of accidental falls. However, current solutions lack the ability to combine low-power consumption, privacy protection, low latency response, and low payload. In this work, we address this gap through a comparative analysis of the trade-off between effectiveness and energy consumption by comparing a Recurrent Spiking Neural Network (RSNN) with a Long Short-Term Memory (LSTM) and a Convolutional Neural Network (CNN). By leveraging two pre-existing RGB datasets and an event-camera simulator, we generated event data by converting intensity frames into event streams. Thus, we could harness the salient features of event-based data and analyze their benefits when combined with RSNNs and LSTMs. The compared approaches are evaluated on two data sets collected from a single subject; one from a camera attached to the neck (N-data) and the other one attached to the waist (W-data). Each data set contains 469 video samples, of which 213 are four types of fall examples, and the rest are nine types of non-fall daily activities. Compared to the CNN, which operates on the high-resolution RGB frames, the RSNN requires 200x less trainable parameters. However, the CNN outperforms the RSNN by 23.7 and 17.1% points for W- and N-data, respectively. Compared to the LSTM, which operates on event-based input, the RSNN requires 5x less trainable parameters and 2000x less MAC operations while exhibiting a 1.9 and 8.7% points decrease in accuracy for W- and N-data, respectively. Overall, our results show that the event-based data preserves enough information to detect falls. Our work paves the way to the realization of high-energy efficient fall detection systems.
更多查看译文
关键词
Fall detection,Wearable cameras,Event-based,Deep learning,RSNN,CNN,LSTM
AI 理解论文
溯源树
样例
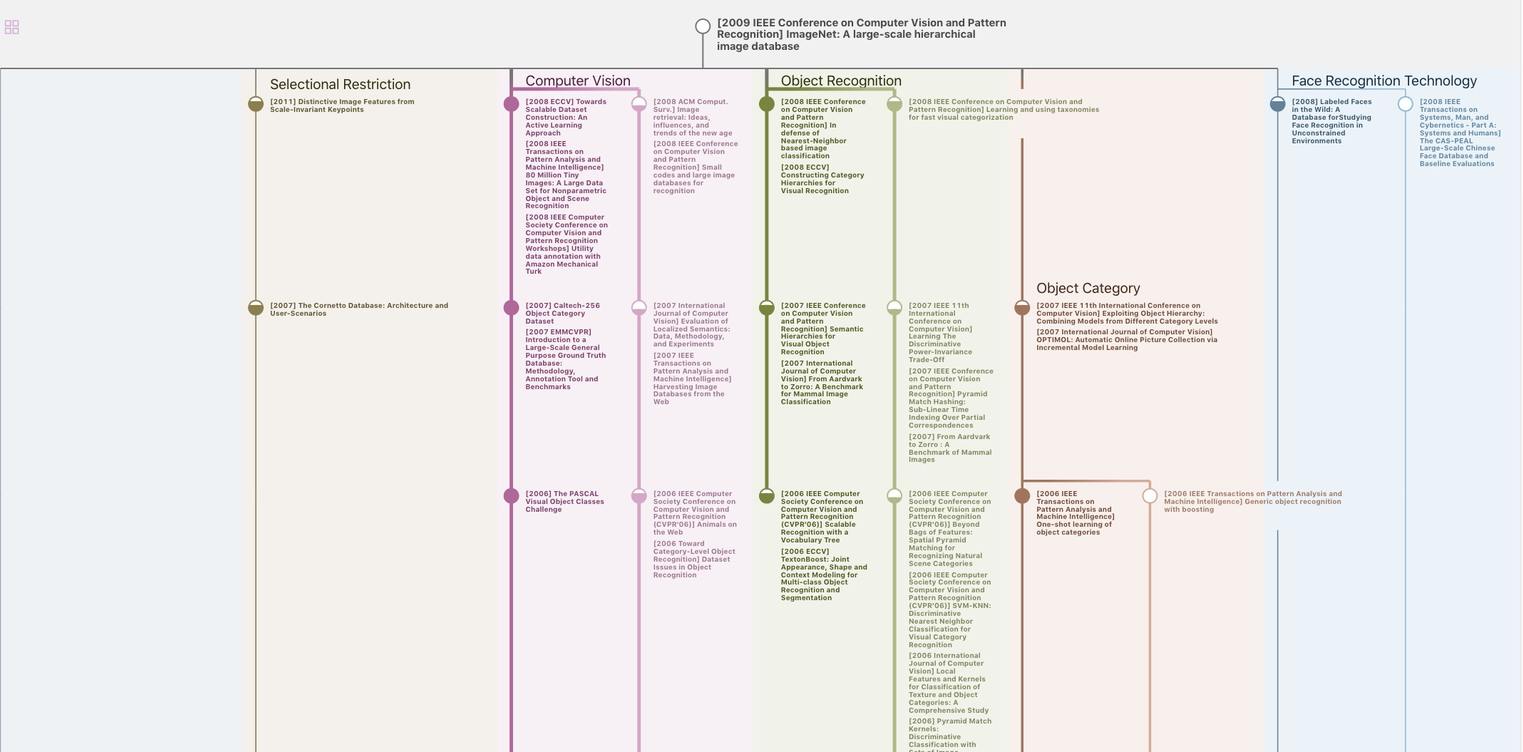
生成溯源树,研究论文发展脉络
Chat Paper
正在生成论文摘要