Using Diffusion Models for Dataset Generation: Prompt Engineering vs. Fine-Tuning
COMPUTER ANALYSIS OF IMAGES AND PATTERNS, CAIP 2023, PT I(2023)
摘要
Despite the notable achievements of deep object detection models, a major challenge remains to be the need for vast amounts of training data. The process of acquiring such real-world data is laborious, prompting the exploration of new research directions such as synthetic data generation. In this study, we assess the capability of two distinct synthetic data generating techniques utilising stable diffusion, namely, (1) Prompt engineering of an established model and (2) Fine-tuning a pretrained model. As a result, we generate two training datasets, manually annotate them, and train separate object detection models for testing on a real-world detection dataset. The results demonstrate that both prompt engineering and fine-tuning exhibit similar performance when tested on a set of 331 real-world images, in the context of apple detection in apple orchards. We compared their performance with the baseline setting where the model was trained on real-world images and witnessed only a 0.07 and 0.08 average precision deviation from the baseline model. Qualitative results demonstrate that both models are able to accurately predict the location of the apples, except in instances of heavy shading. This study distinguishes itself from prior research by focusing on object detection instead of image classification. Furthermore, we are the first to apply diffusion model fine-tuning in the context of dataset generation. Our findings underscore the potential of synthetic data generation as a viable alternative to the laborious collection of extensive training data for object detection models.
更多查看译文
关键词
Stable Diffusion,Prompt Engineering,DreamBooth,Detection
AI 理解论文
溯源树
样例
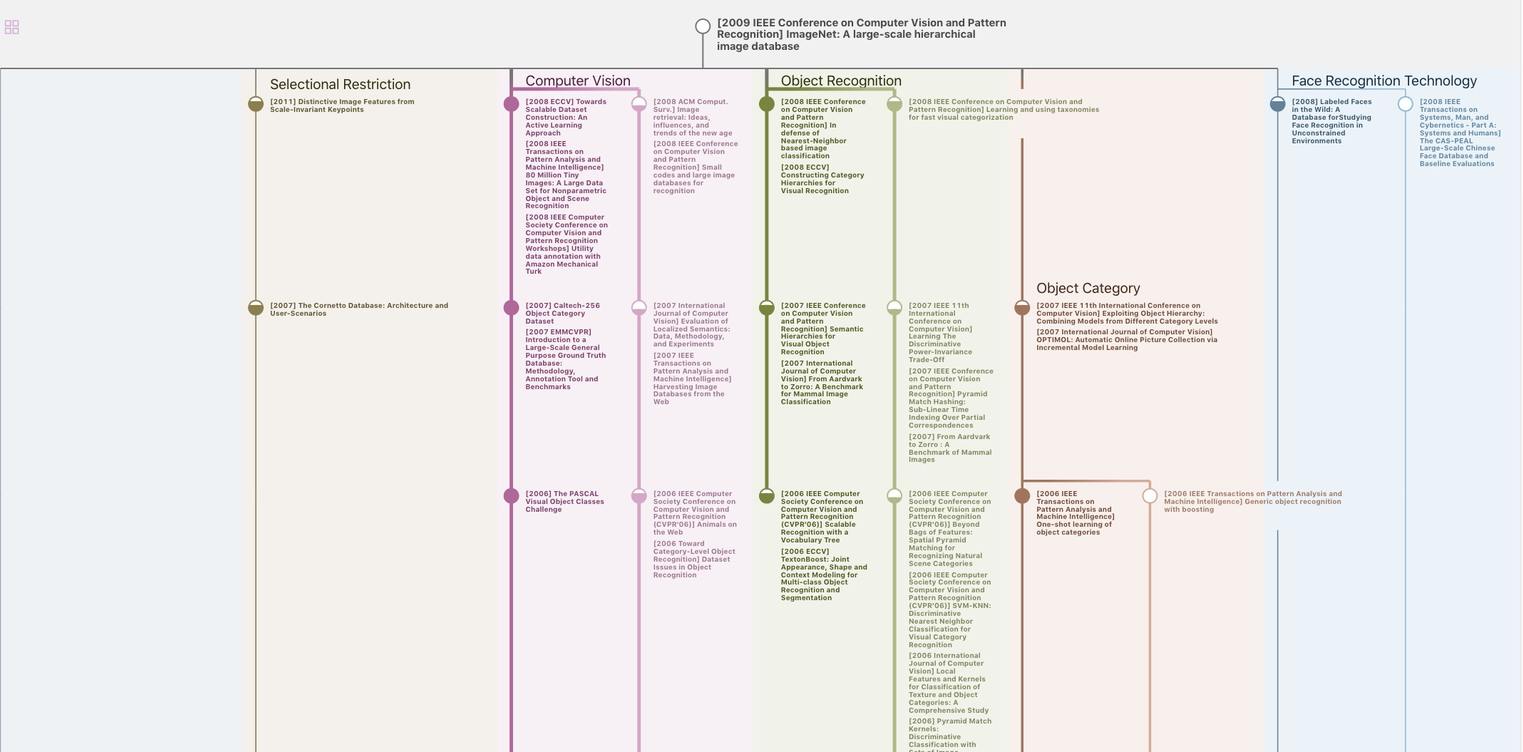
生成溯源树,研究论文发展脉络
Chat Paper
正在生成论文摘要