kProp: Multi-neuron Relaxation Method for Neural Network Robustness Verification.
FSEN(2023)
摘要
With the increasing application of neural networks in safety-critical domains, their robustness becomes a crucial concern. In this paper, we present a multi-neuron relaxation-based verification framework kProp for ReLU neural networks with adversarial distortions in general norms. In contrast with existing verification methods tackling general distortion norms, the proposed multi-neuron relaxation method is able to capture the relations among a group of neurons, thus providing tighter convex relaxations and improving verification precision. In addition, existing methods based on linear relaxation may include infeasible inputs to the neural network for robustness verification, which further leads to verification precision loss. To address this problem, we propose a region clipping method to exclude infeasible inputs to further improve the verification precision. We implement our verification framework and evaluate its performance on open-source benchmarks. The experiments show that kProp can produce precise verification results where existing verification methods fail to produce conclusive results, and can be applied to neural networks with more than 4k neurons in general distortion norms.
更多查看译文
关键词
neural network robustness verification,neural network,multi-neuron
AI 理解论文
溯源树
样例
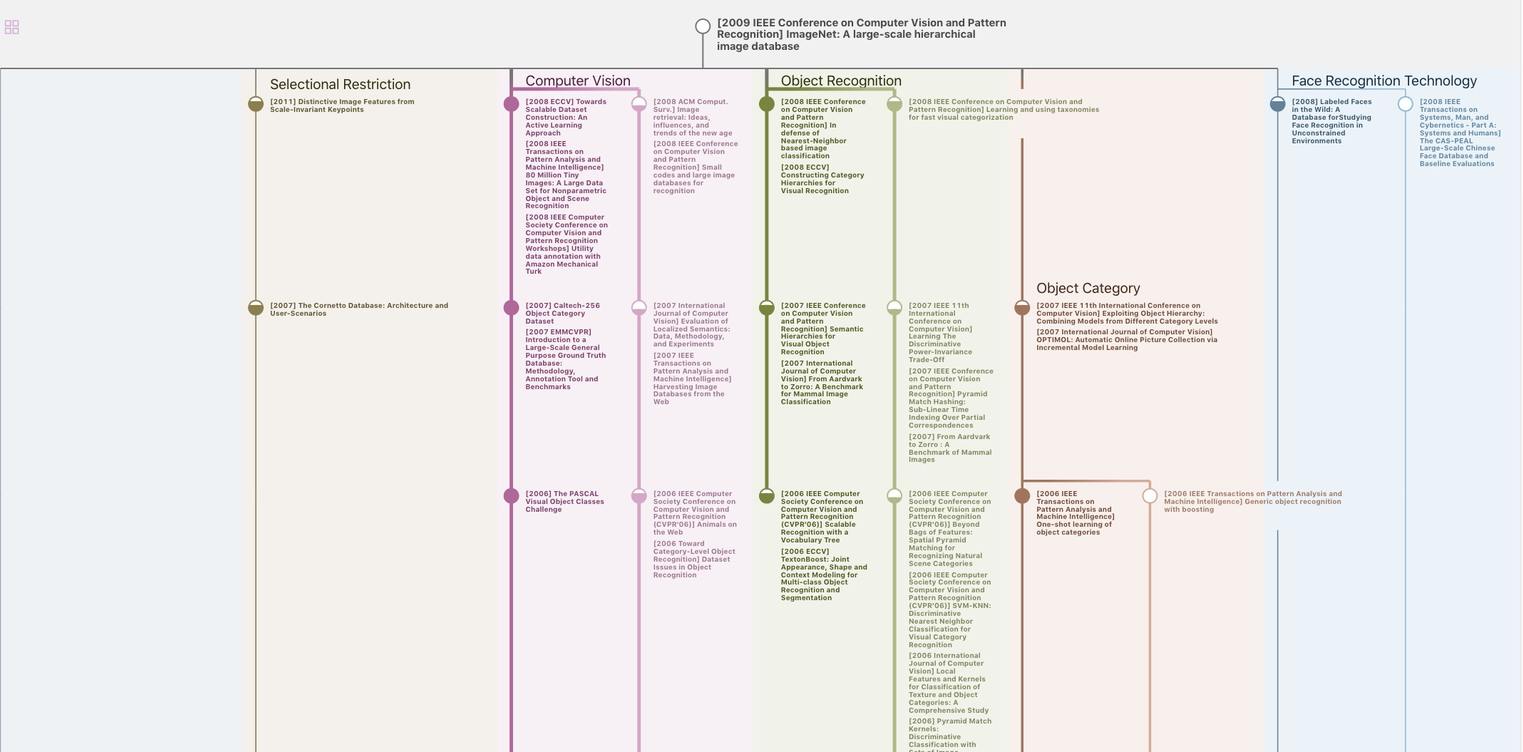
生成溯源树,研究论文发展脉络
Chat Paper
正在生成论文摘要