Financial Distress Prediction in an Imbalanced Data Stream Environment.
HAIS(2023)
摘要
Corporate bankruptcy predictions are crucial to companies, investors, and authorities. However, most bankruptcy prediction studies have been based on stationary models, and they tend to ignore important challenges of financial distress like data non-stationarity, concept drift and data imbalance. This study proposes methods for dealing with these challenges and uses data collected from financial statements quarterly provided by companies to the Securities and Exchange Commission of Brazil (CVM). It is composed of information from 10 years (2011 to 2020), with 905 different corporations and 23,834 records with 82 indicators each. The sample majority have no financial difficulties, and only 651 companies have financial distress. The empirical experiment uses a sliding window, a history and a forgetting mechanism to avoid the degradation of the predictive model due to concept drift. The characteristics of the problem, especially the data imbalance, the performance of the models is measured through AUC, G mean , and F 1 -Score and achieved 0.95, 0.68, and 0.58, respectively.
更多查看译文
关键词
prediction,data
AI 理解论文
溯源树
样例
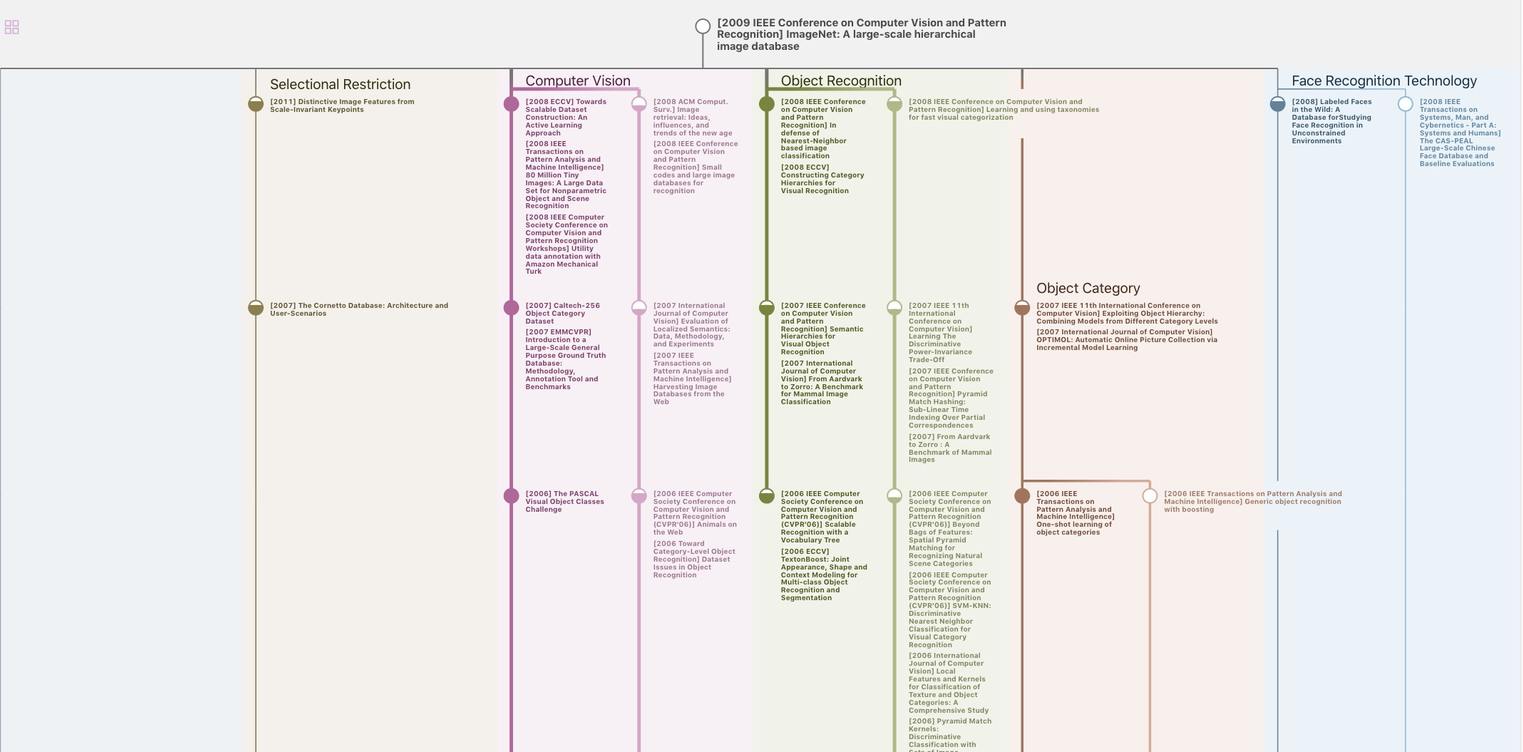
生成溯源树,研究论文发展脉络
Chat Paper
正在生成论文摘要