Meta-Learning for Hyperparameters Tuning in CNNs for Chest Images.
HAIS(2023)
摘要
Hyperparameter tuning is a time-consuming task for deep learning models. Meta-learning offers a promising solution to reduce the time required for this task. In this work, we propose a meta-learning approach to simulate a set of experiments and select a hyperparameter configuration (HC) that achieves high accuracy using a deep model. Our formulation involves conducting a grid search over hyperparameters to train a convolutional neural network and get an overview of their space. Then, a meta-regressor was trained using the experiment data to predict accuracy as a function of hyperparameter sets. Subsequently, the trained meta-regressor was employed to simulate diverse HCs and assess the corresponding deep model performance. Our approach was tested across two different domains: COVID-19 detection using X-ray images, and lung detection in computer tomography volumes. Furthermore, we evaluated the proposed approach with two different architectures. Our results show that the proposed method can simulate a set of experiments using the meta-regressor, saving time and computing resources during hyperparameter tuning.
更多查看译文
关键词
cnns,hyperparameters,images,meta-learning
AI 理解论文
溯源树
样例
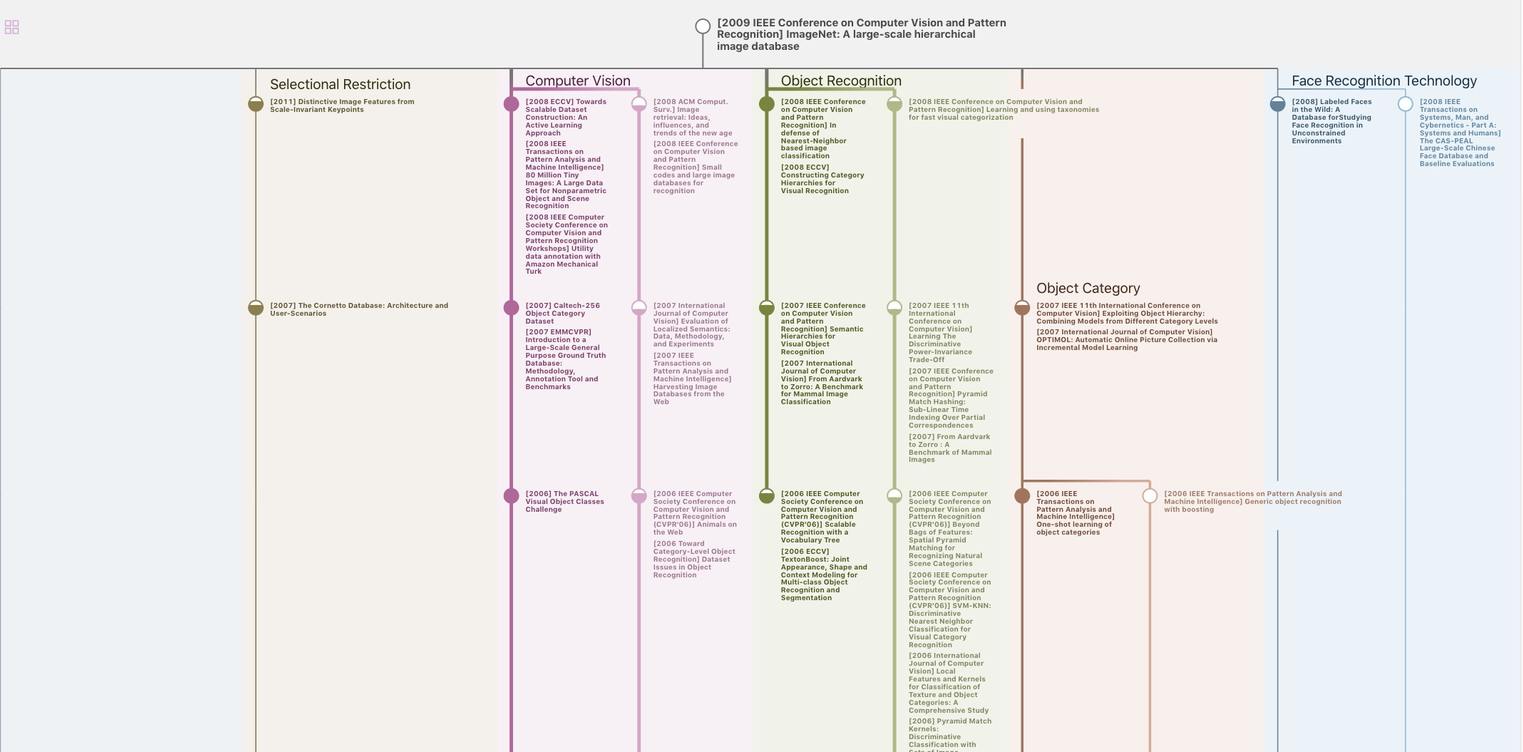
生成溯源树,研究论文发展脉络
Chat Paper
正在生成论文摘要