Inference of Infrastructure Network Flows via Physics-Inspired Implicit Neural Networks.
CCTA(2023)
摘要
We study the problem of inferring edge flows and nodal injections in infrastructure networks. Leveraging the Thomson’s Principle from the electric circuits literature, we setup a framework to jointly learn network parameters and missing states. Despite being application agnostic, the proposed approach captures the fundamental physics of the infrastructure, and is able to handle partial observation, node and edge features as well as operational constraints. The physics inspired learning framework leads to a bilevel optimization problem, which is NP hard in general. By exploiting convexity properties, we reformulate the problem as a single level optimization, composed of a graph neural network and an additional implicit layer. The resulting architecture can be trained using standard gradient-based methods. We assess the validity of the proposed approach on two different infrastructure networks (power and traffic), and show it outperforms the current state of the art.
更多查看译文
关键词
bilevel optimization problem,edge features,edge flows,electric circuits literature,fundamental physics,gradient-based methods,graph neural network,infrastructure network flows,network parameters,NP hard,physics-inspired implicit neural networks,single level optimization,Thomsons principle
AI 理解论文
溯源树
样例
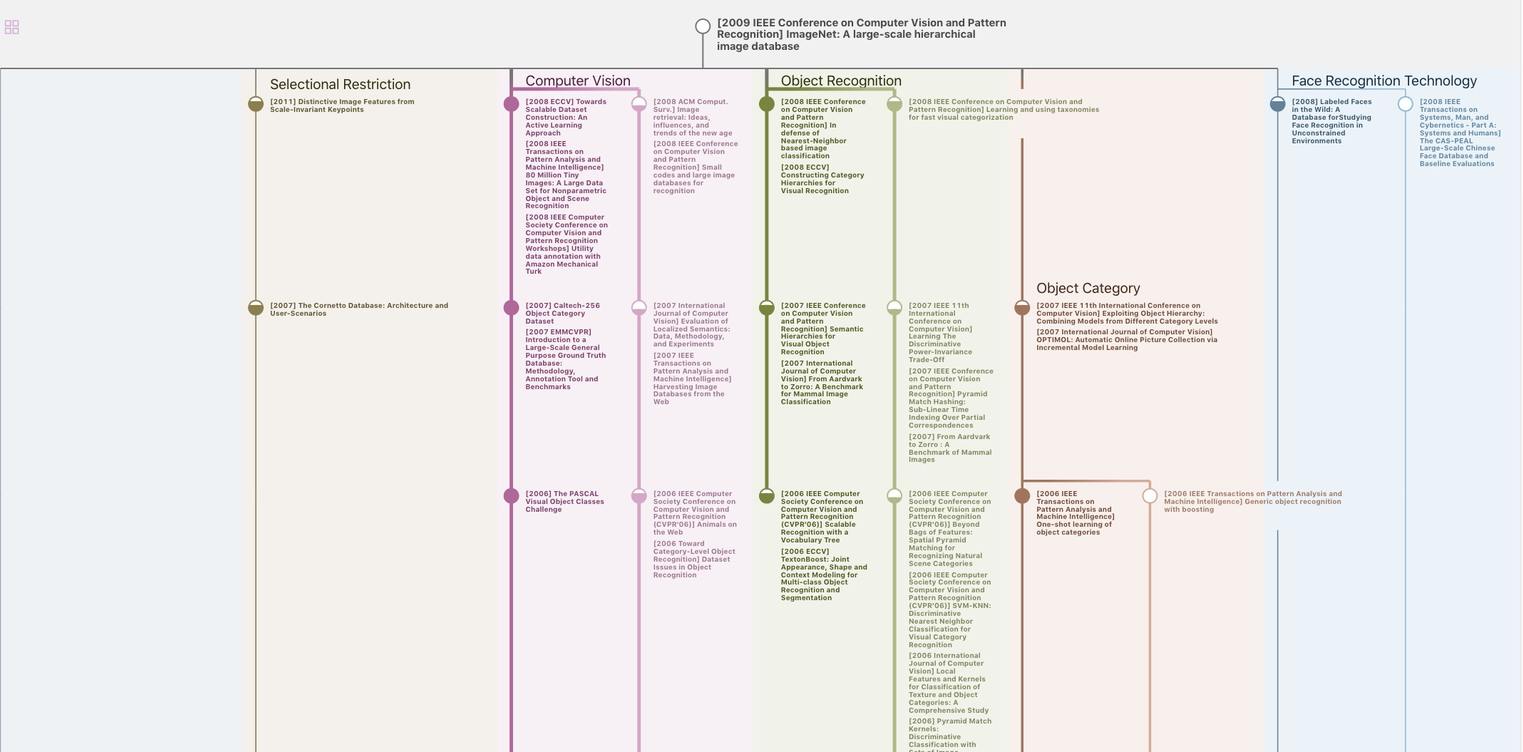
生成溯源树,研究论文发展脉络
Chat Paper
正在生成论文摘要