An Assessment of Self-supervised Learning for Data Efficient Potato Instance Segmentation
TOWARDS AUTONOMOUS ROBOTIC SYSTEMS, TAROS 2023(2023)
摘要
This work examines the viability of self-supervised learning approaches in the field of agri-robotics, specifically focusing on the segmentation of densely packed potato tubers in storage. The work assesses the impact of both the quantity and quality of data on self-supervised training, employing a limited set of both annotated and unannotated data. Mask R-CNN with a ResNet50 backbone is used for instance segmentation to evaluate self-supervised training performance. The results indicate that the self-supervised methods employed have a modest yet beneficial impact on the downstream task. A simpler approach yields more effective results with a larger dataset, whereas a more intricate method shows superior performance with a refined, smaller self-supervised dataset.
更多查看译文
关键词
Self Supervised Learning,Instance Segmentation,Small Datasets,Agriculture,Agri-robotics
AI 理解论文
溯源树
样例
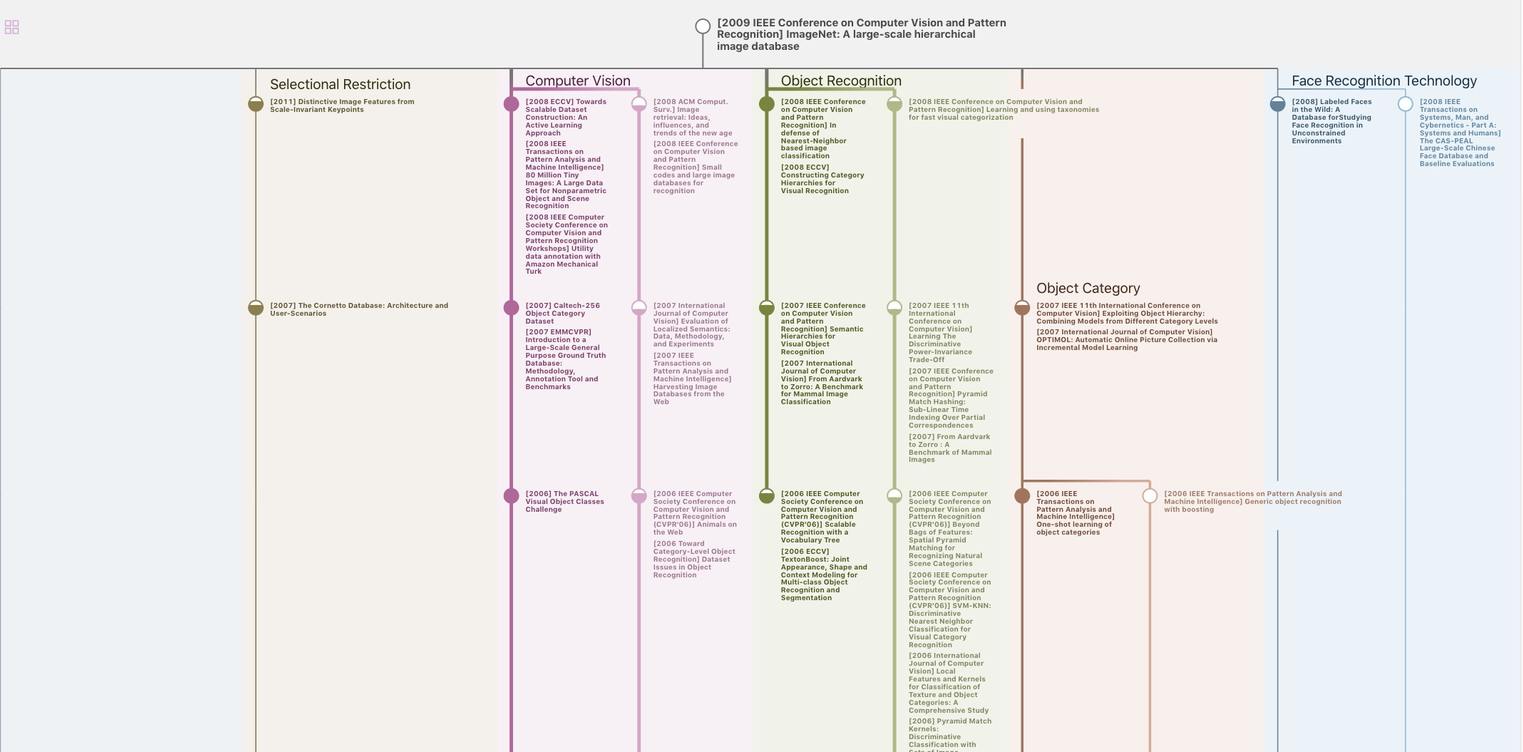
生成溯源树,研究论文发展脉络
Chat Paper
正在生成论文摘要