Unified Agile Accuracy Assessment in Computing-in-Memory Neural Accelerators by Layerwise Dynamical Isometry.
DAC(2023)
摘要
Deploying neural networks (NN) on computing-in-memory (CIM) neural accelerators incurs additional hardware factors in the test accuracy, which add substantial extra evaluation overhead. This work takes the first step to quantitatively analyze how information propagates in CIM neural accelerators as well as how additional CIM factors influence that information propagation. From our analysis, we propose a new metric named Unified-QCN that is theoretically linked to the test accuracy according to layerwise dynamical isometry (LDI), providing us with a compass to avoid direct time-consuming simulations. Our method consistently delivers high correlations with the test accuracy for various NN backbones on different datasets.
更多查看译文
关键词
quantization,computing-in-memory accelerator,neural architecture search
AI 理解论文
溯源树
样例
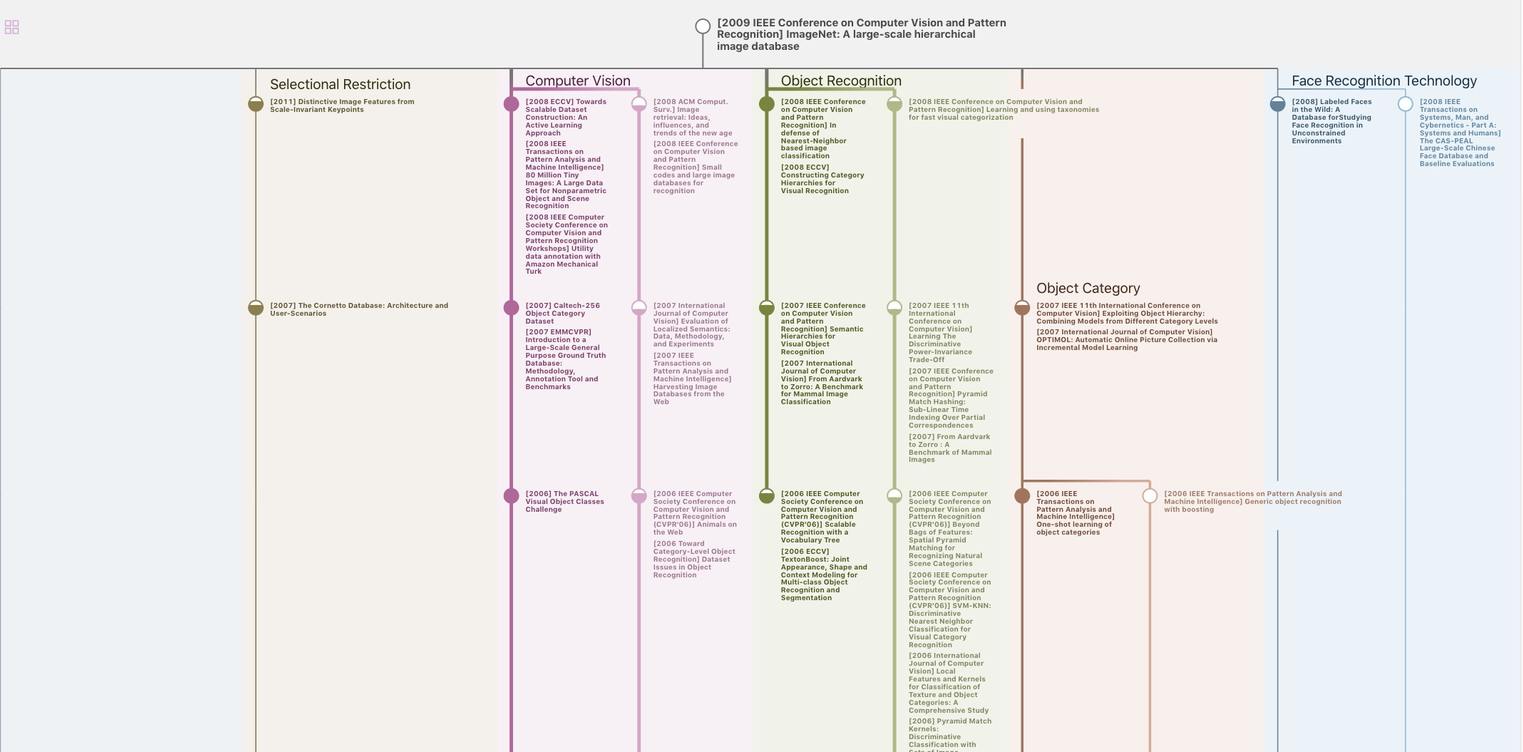
生成溯源树,研究论文发展脉络
Chat Paper
正在生成论文摘要