Graph Representation Learning for Microarchitecture Design Space Exploration.
DAC(2023)
摘要
Design optimization of modern microprocessors is a complex task due to the exponential growth of the design space. This work presents GRL-DSE, an automatic microarchitecture search framework based on graph embeddings. GRL-DSE uses graph representation learning to build a compact and continuous embedding space. Multi-objective Bayesian optimization using an ensemble surrogate model conducts microarchitecture design space exploration in the graph embedding space to efficiently and holistically optimize performance-power-area (PPA) objectives. Experimental studies on RISC-V BOOM show that GRL-DSE outperforms previous techniques by 74.59% on Pareto front quality and outperforms manual designs in terms of PPA.
更多查看译文
关键词
automatic microarchitecture search framework,compact embedding space,complex task,continuous embedding space,design optimization,ensemble surrogate model conducts microarchitecture design space exploration,exponential growth,graph embedding space,graph embeddings,graph representation learning,GRL-DSE uses graph representation,GRLDSE,manual designs,modern microprocessors,multiobjective Bayesian optimization,performance-power-area objectives
AI 理解论文
溯源树
样例
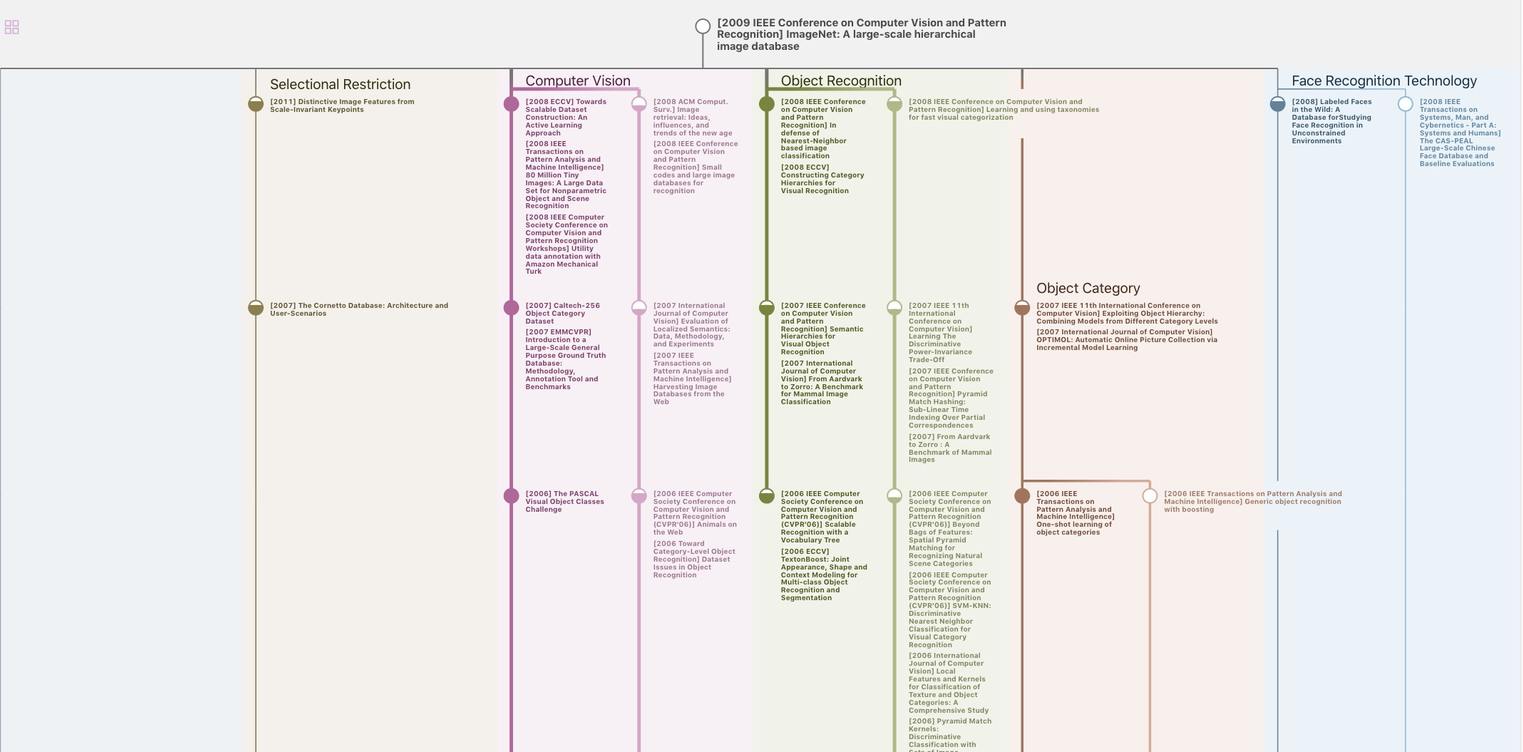
生成溯源树,研究论文发展脉络
Chat Paper
正在生成论文摘要