A Learnable Counter-Condition Analysis Framework for Functional Connectivity-Based Neurological Disorder Diagnosis.
IEEE transactions on medical imaging(2024)
摘要
To understand the biological characteristics of neurological disorders with functional connectivity (FC), recent studies have widely utilized deep learning-based models to identify the disease and conducted post-hoc analyses via explainable models to discover disease-related biomarkers. Most existing frameworks consist of three stages, namely, feature selection, feature extraction for classification, and analysis, where each stage is implemented separately. However, if the results at each stage lack reliability, it can cause misdiagnosis and incorrect analysis in afterward stages. In this study, we propose a novel unified framework that systemically integrates diagnoses (i.e., feature selection and feature extraction) and explanations. Notably, we devised an adaptive attention network as a feature selection approach to identify individual-specific disease-related connections. We also propose a functional network relational encoder that summarizes the global topological properties of FC by learning the inter-network relations without pre-defined edges between functional networks. Last but not least, our framework provides a novel explanatory power for neuroscientific interpretation, also termed counter-condition analysis. We simulated the FC that reverses the diagnostic information (i.e., counter-condition FC): converting a normal brain to be abnormal and vice versa. We validated the effectiveness of our framework by using two large resting-state functional magnetic resonance imaging (fMRI) datasets, Autism Brain Imaging Data Exchange (ABIDE) and REST-meta-MDD, and demonstrated that our framework outperforms other competing methods for disease identification. Furthermore, we analyzed the disease-related neurological patterns based on counter-condition analysis.
更多查看译文
关键词
Resting-state,fMRI,Adaptive feature selection,Transformer,Prototype learning,Explainable AI
AI 理解论文
溯源树
样例
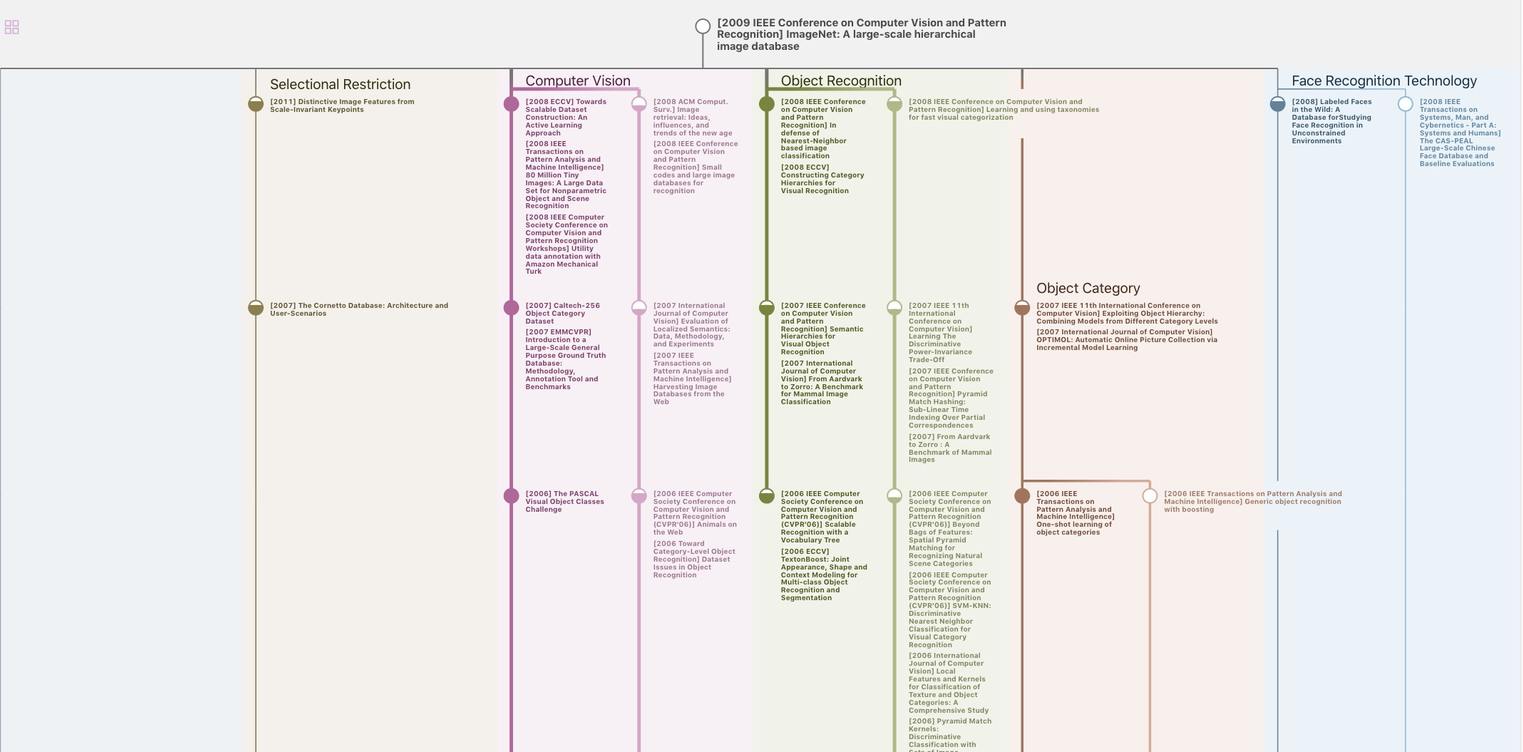
生成溯源树,研究论文发展脉络
Chat Paper
正在生成论文摘要