Chameleon: Increasing Label-Only Membership Leakage with Adaptive Poisoning
ICLR 2024(2023)
摘要
The integration of machine learning (ML) in numerous critical applications
introduces a range of privacy concerns for individuals who provide their
datasets for model training. One such privacy risk is Membership Inference
(MI), in which an attacker seeks to determine whether a particular data sample
was included in the training dataset of a model. Current state-of-the-art MI
attacks capitalize on access to the model's predicted confidence scores to
successfully perform membership inference, and employ data poisoning to further
enhance their effectiveness. In this work, we focus on the less explored and
more realistic label-only setting, where the model provides only the predicted
label on a queried sample. We show that existing label-only MI attacks are
ineffective at inferring membership in the low False Positive Rate (FPR)
regime. To address this challenge, we propose a new attack Chameleon that
leverages a novel adaptive data poisoning strategy and an efficient query
selection method to achieve significantly more accurate membership inference
than existing label-only attacks, especially at low FPRs.
更多查看译文
关键词
Privacy Attack,Membership Inference,Data Poisoning
AI 理解论文
溯源树
样例
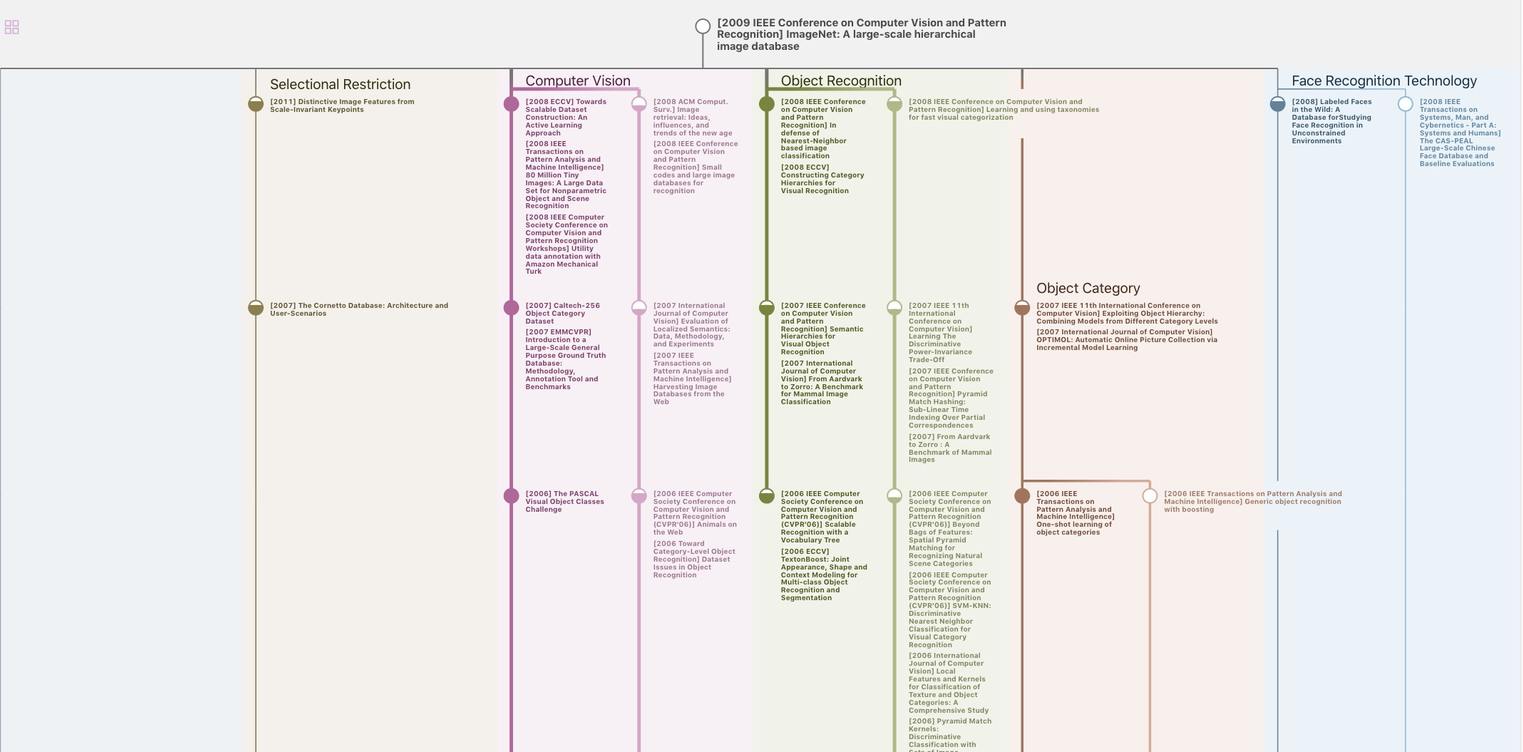
生成溯源树,研究论文发展脉络
Chat Paper
正在生成论文摘要