Predicting the impact of feedback on matter clustering with machine learning in CAMELS
MONTHLY NOTICES OF THE ROYAL ASTRONOMICAL SOCIETY(2023)
摘要
Extracting information from the total matter power spectrum with the precision needed for upcoming cosmological surveys requires unraveling the complex effects of galaxy formation processes on the distribution of matter. We investigate the impact of baryonic physics on matter clustering at z = 0 using a library of power spectra from the Cosmology and Astrophysics with MachinE Learning Simulations project, containing thousands of (25(-1)Mpc)(3) volume realizations with varying cosmology, initial random field, stellar and active galactic nucleus (AGN) feedback strength and subgrid model implementation methods. We show that baryonic physics affects matter clustering on scales k greater than or similar to 0.4Mpc(-1) and the magnitude of this effect is dependent on the details of the galaxy formation implementation and variations of cosmological and astrophysical parameters. Increasing AGN feedback strength decreases halo baryon fractions and yields stronger suppression of power relative to N-body simulations, while stronger stellar feedback often results in weaker effects by suppressing black hole growth and therefore the impact of AGN feedback. We find a broad correlation between mean baryon fraction of massive haloes (M-200c > 10(13.5) M-circle dot) and suppression of matter clustering but with significant scatter compared to previous work owing to wider exploration of feedback parameters and cosmic variance effects. We show that a random forest regressor trained on the baryon content and abundance of haloes across the full mass range 10(10) <= M-halo/M-circle dot<10(15) can predict the effect of galaxy formation on the matter power spectrum on scales k = 1.0-20.0 Mpc(-1).
更多查看译文
关键词
methods: numerical,galaxies: haloes,large-scale structure of Universe,cosmology: theory
AI 理解论文
溯源树
样例
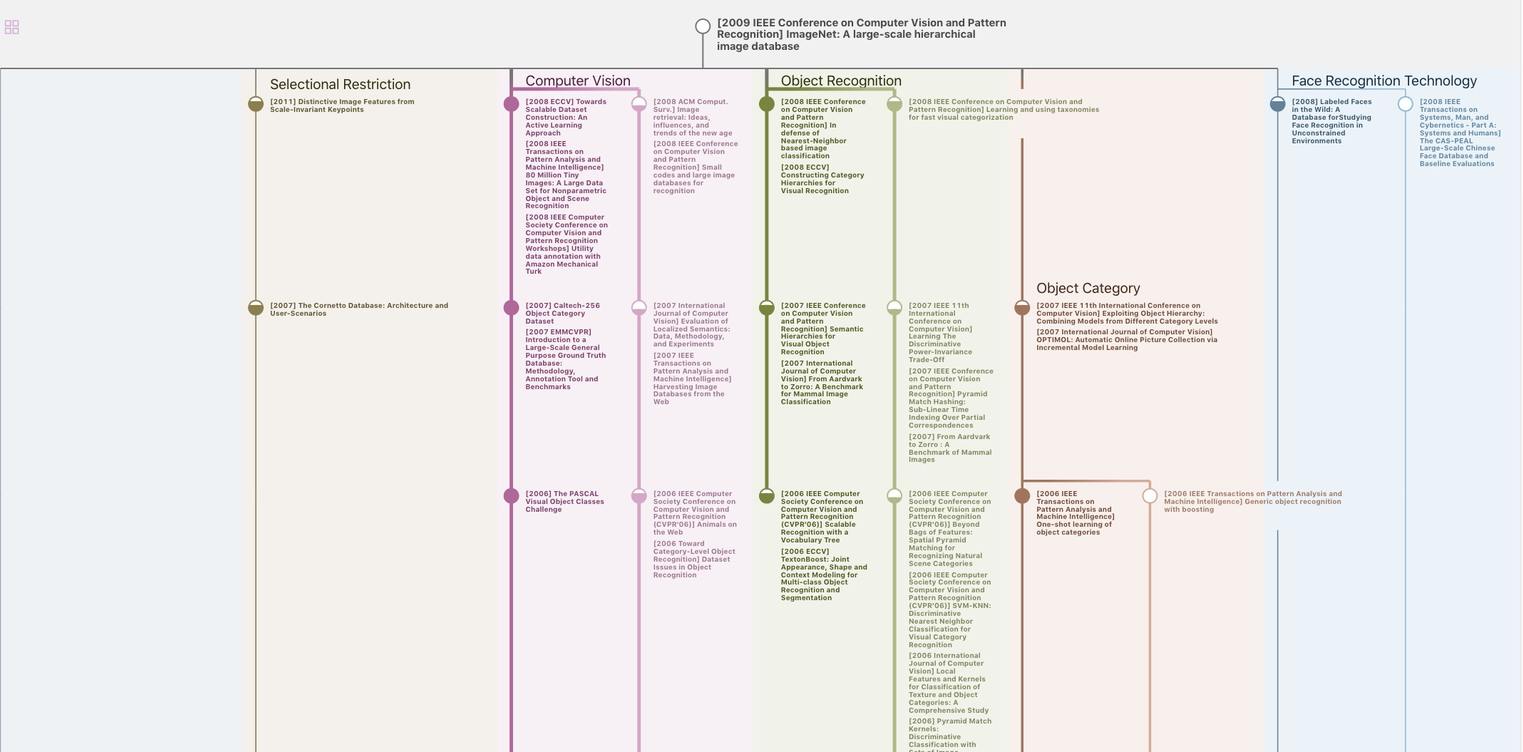
生成溯源树,研究论文发展脉络
Chat Paper
正在生成论文摘要