Inferring plasticity rules from single-neuron spike trains using deep learning methods
bioRxiv (Cold Spring Harbor Laboratory)(2023)
摘要
Synaptic plasticity is a core basis for learning and adaptation. Determining how synapses are altered by local signals – the learning rules – is the hinge about which brain activity pivots. A large number of in vitro characterizations have focused on restricted sets of core properties [e.g., spike-timing-dependent plasticity (STDP), burst-dependent plasticity (BDP)], but it remains to be established which learning rule is most consistent with the changes in activity patterns observed while the network learns to perform a task. To address this question, we hypothesize that correlations between features of single post-synaptic neural activity and subsequent plasticity of the representations could distinguish between learning rules. Because this correlation was expected to be diluted by the notoriously large variability of brain activity, we propose to infer the learning rules from passive observations using artificial neural networks. Using surrogate data to test the validity of our approach, we found that transformers and temporal convolutional networks could classify learning rules far above the chance level (20%), with transformers achieving a better overall accuracy (78%) than the convolutional models (73%). This performance is achieved despite the presence of noise and representational drift. Although not as accurate as the complex transformer-based classifier, the convolutional model can provide insights into the interpretable features used by the algorithm to perform the classification. Our work establishes support for using deep learning methods to infer the category of learning rules given spiking data.
### Competing Interest Statement
The authors have declared no competing interest.
更多查看译文
关键词
spike trains,single-neuron single-neuron,plasticity rules
AI 理解论文
溯源树
样例
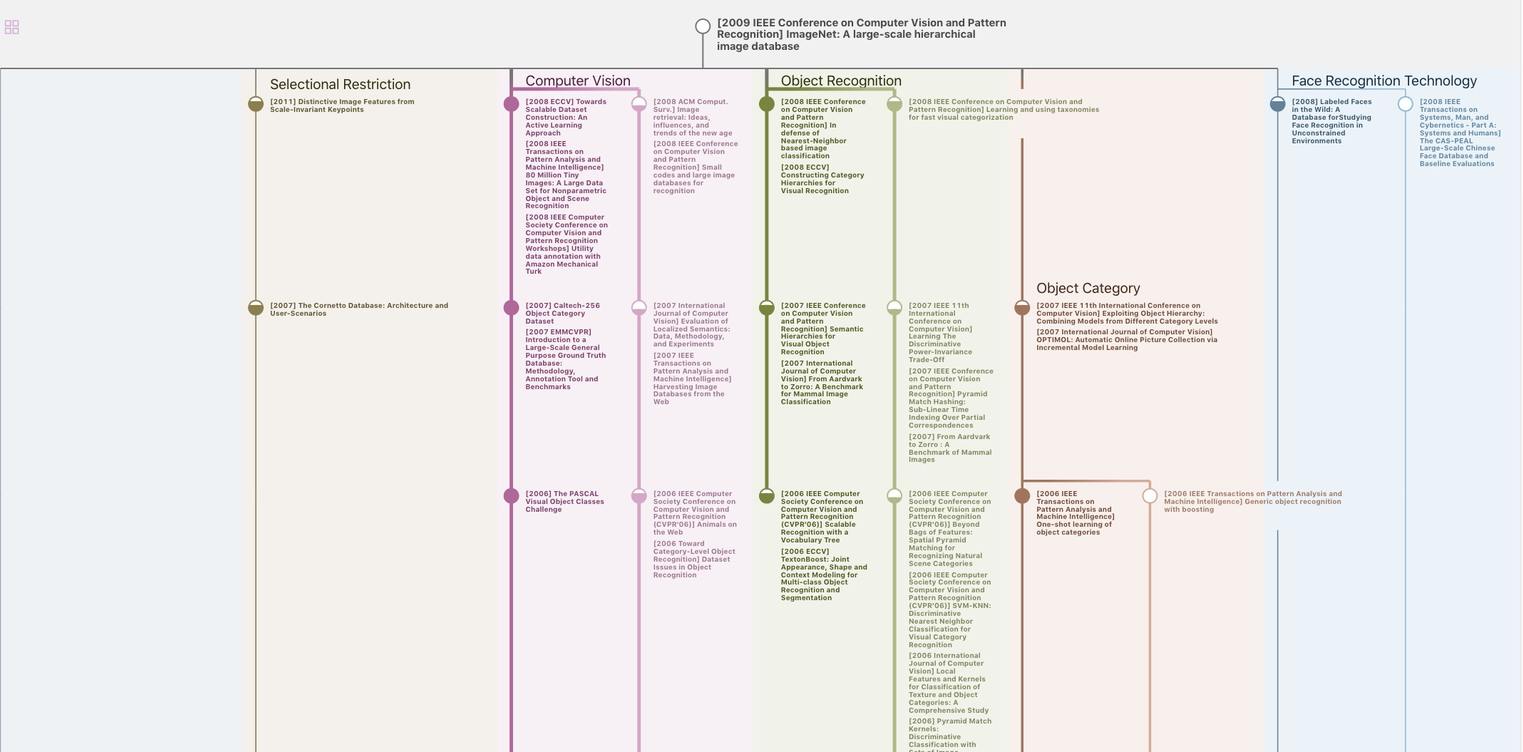
生成溯源树,研究论文发展脉络
Chat Paper
正在生成论文摘要