Adversarial Transformer for Repairing Human Airway Segmentation
IEEE Journal of Biomedical and Health Informatics(2023)
摘要
Automated airway segmentation models often suffer from discontinuities in peripheral bronchioles, which limits their clinical applicability. Furthermore, data heterogeneity across different centres and pathological abnormalities pose significant challenges to achieving accurate and robust segmentation in distal small airways. Accurate segmentation of airway structures is essential for the diagnosis and prognosis of lung diseases. To address these issues, we propose a patch-scale adversarial-based refinement network that takes in preliminary segmentation and original CT images and outputs a refined mask of the airway structure. Our method is validated on three datasets, including healthy cases, pulmonary fibrosis, and COVID-19 cases, and quantitatively evaluated using seven metrics. Our method achieves more than a 15% increase in the detected length ratio and detected branch ratio compared to previously proposed models, demonstrating its promising performance. The visual results show that our refinement approach, guided by a patch-scale discriminator and centreline objective functions, effectively detects discontinuities and missing bronchioles. We also demonstrate the generalizability of our refinement pipeline on three previous models, significantly improving their segmentation completeness. Our method provides a robust and accurate airway segmentation tool that can help improve diagnosis and treatment planning for lung diseases.
更多查看译文
关键词
Segmentation, airway, GAN, transformer, refinement, explainable
AI 理解论文
溯源树
样例
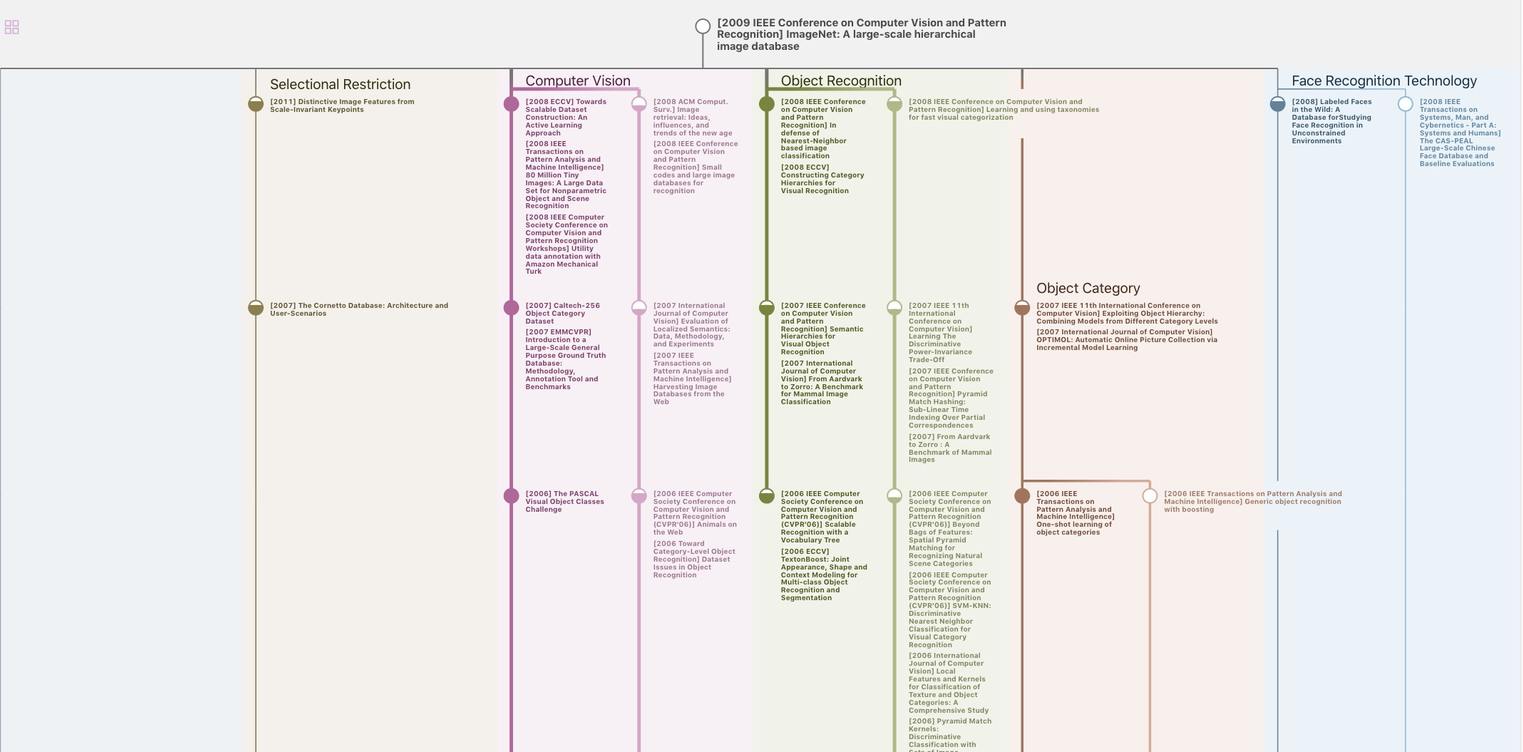
生成溯源树,研究论文发展脉络
Chat Paper
正在生成论文摘要