Causal Inference in Gene Regulatory Networks with GFlowNet: Towards Scalability in Large Systems
CoRR(2023)
摘要
Understanding causal relationships within Gene Regulatory Networks (GRNs) is essential for unraveling the gene interactions in cellular processes. However, causal discovery in GRNs is a challenging problem for multiple reasons including the existence of cyclic feedback loops and uncertainty that yields diverse possible causal structures. Previous works in this area either ignore cyclic dynamics (assume acyclic structure) or struggle with scalability. We introduce Swift-DynGFN as a novel framework that enhances causal structure learning in GRNs while addressing scalability concerns. Specifically, Swift-DynGFN exploits gene-wise independence to boost parallelization and to lower computational cost. Experiments on real single-cell RNA velocity and synthetic GRN datasets showcase the advancement in learning causal structure in GRNs and scalability in larger systems.
更多查看译文
关键词
gene regulatory networks,regulatory networks,gflownet
AI 理解论文
溯源树
样例
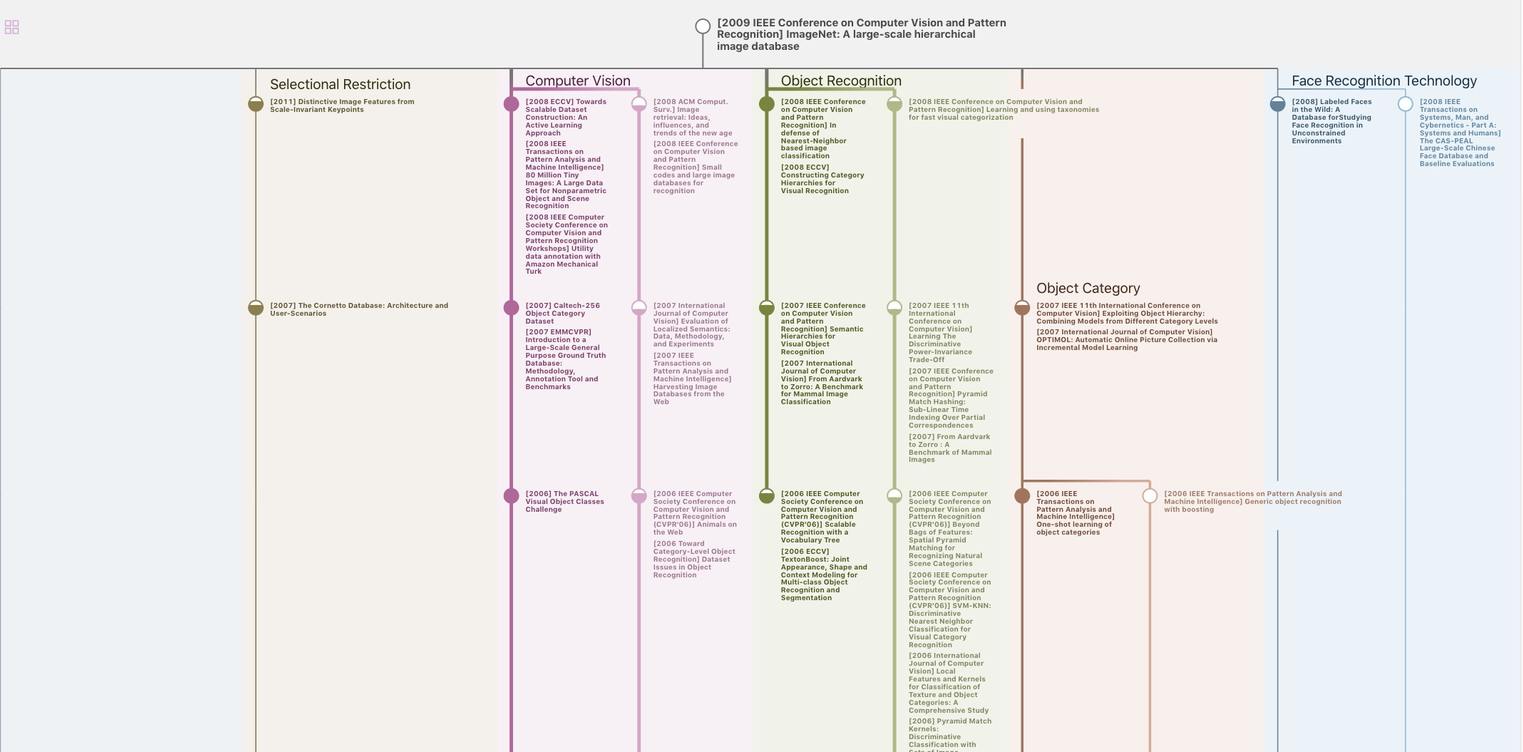
生成溯源树,研究论文发展脉络
Chat Paper
正在生成论文摘要