Learning Personalized Story Evaluation
CoRR(2023)
摘要
While large language models (LLMs) have shown impressive results for more objective tasks such as QA and retrieval, it remains nontrivial to evaluate their performance on open-ended text generation for reasons including (1) data contamination; (2) multi-dimensional evaluation criteria; and (3) subjectiveness stemming from reviewers' personal preferences. To address such issues, we propose to model personalization in an uncontaminated open-ended generation assessment. We create two new datasets Per-MPST and Per-DOC for personalized story evaluation, by re-purposing existing datasets with proper anonymization and new personalized labels. We further develop a personalized story evaluation model PERSE to infer reviewer preferences and provide a personalized evaluation. Specifically, given a few exemplary reviews from a particular reviewer, PERSE predicts either a detailed review or fine-grained comparison in several aspects (such as interestingness and surprise) for that reviewer on a new text input. Experimental results show that PERSE outperforms GPT-4 by 15.8% on Kendall correlation of story ratings, and by 13.7% on pairwise preference prediction accuracy. Both datasets and code will be released at https://github.com/dqwang122/PerSE.
更多查看译文
关键词
evaluation,learning,story
AI 理解论文
溯源树
样例
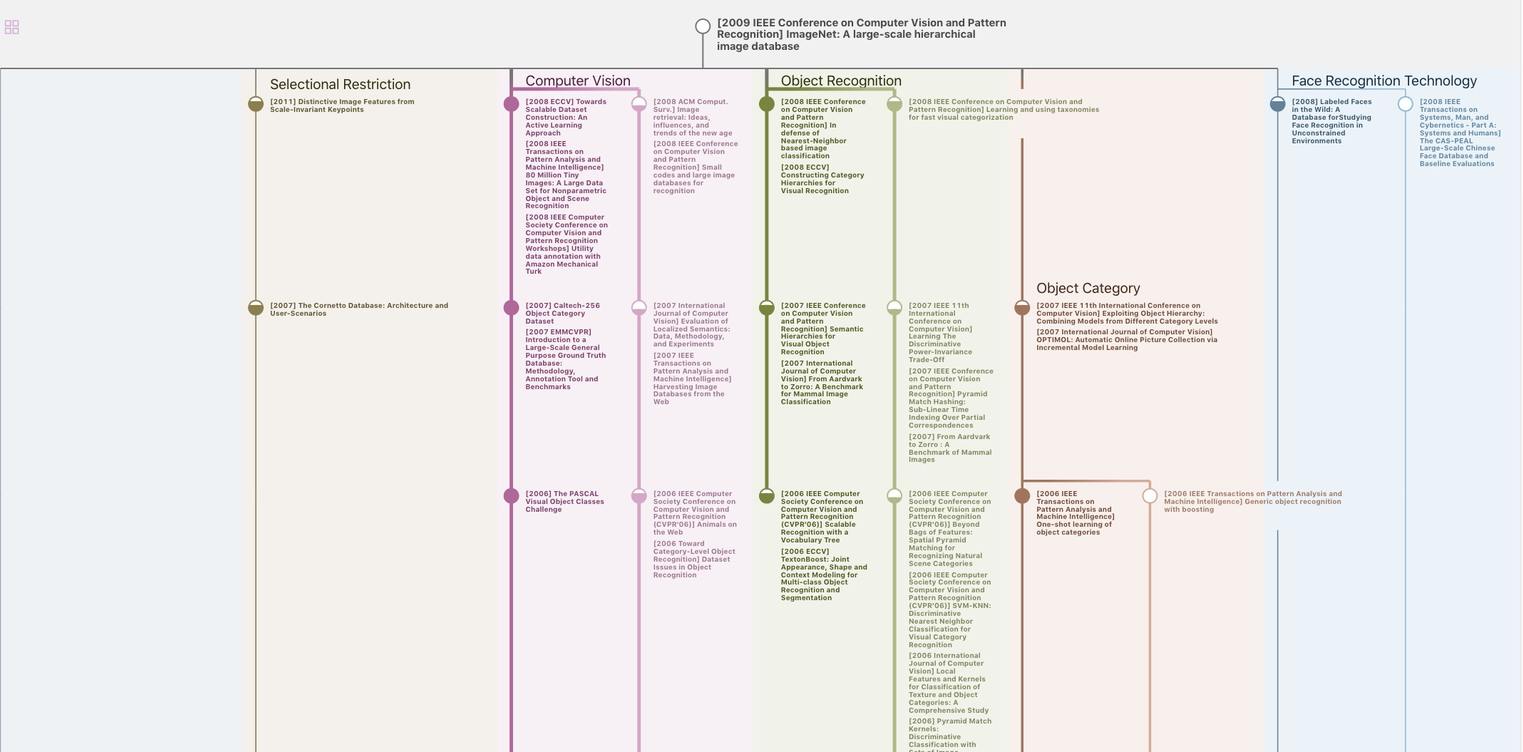
生成溯源树,研究论文发展脉络
Chat Paper
正在生成论文摘要